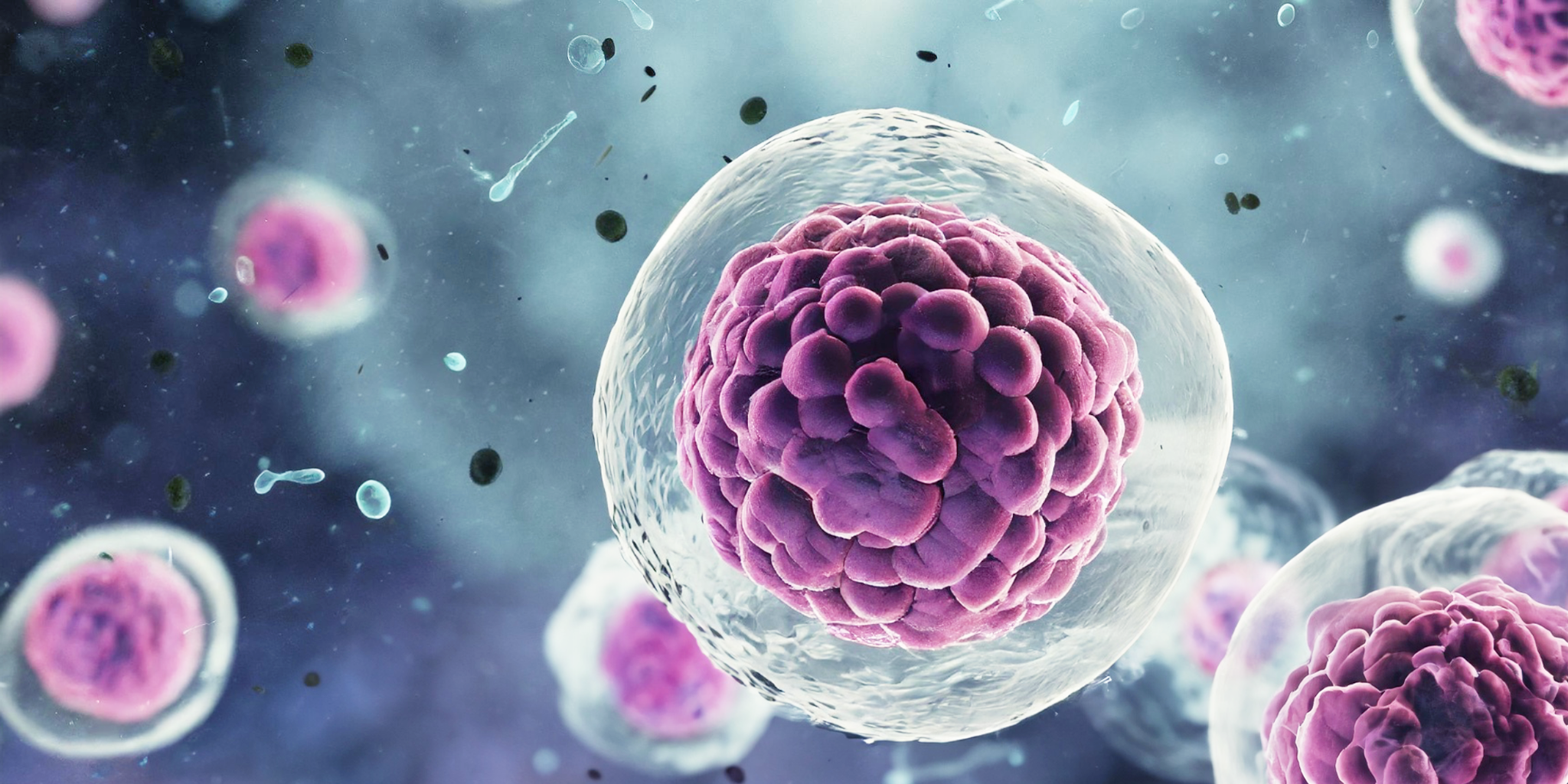
Single-Cell Omics: The Frontier of Biological Research and Computational Innovation
Key takeaways:
- Single-Cell Precision: Single-cell omics enable detailed study of individual cells, providing an understanding of their specific cellular functions and the complex biological systems they compose. This provides insights which was impossible to achieve with bulk analysis methods.
- Computational Demands: The field faces significant computational challenges, including managing large data volumes, interpreting complex data, and developing precise algorithms for accurate analysis.
- Innovative Tools: Developing and integrating specialized software tools, such as Seurat and Scanpy, are critical for efficiently processing and analyzing single-cell omics data.
- Collaboration is Key: Advancements in single-cell omics demand collaboration, data standardization, and knowledge sharing within the scientific community for consistency and quality.
- Impact and Future Potential: Overcoming computational obstacles in single-cell omics can lead to significant real-world applications like developing new drug targets and advancements in personalized medicine. It also enhances more informed environmental monitoring.
Single-cell omics is a breakthrough in modern biological research, revealing details of cell behavior with remarkable clarity. This method has changed the game by letting us study cells individually, uncovering the unique characteristics lost when we only looked at groups of cells together. By focusing on the individual molecular patterns of each cell, single-cell omics is leading us to a richer understanding of biology's building blocks. It's like moving from a painting where colors blend into one to a detailed mosaic where each color stands out. However, this field contains several computational challenges that impact efforts to harness single-cell omics to drive biological insights.
Why Single-Cell Omics Matter?
Single-cell omics reveal insights into individual cells unlocking hidden details missed by analysing large cell groups. This method enables us to understand the diversity and complexity of cells in a particular tissue or organism. Identifying subpopulations can lead to diverse and exciting insights, elucidating a cell's role in health maintenance and disease progression. the technology uncovers individual cells' roles in maintaining health or contributing to disease.
Additionally, single-cell omics is key in revealing cellular heterogeneity — the subtle differences between cells in the same group. These differences have significant implications, especially in developing targeted therapies and personalized medicine. By recognizing and analyzing the variations, we can understand each cell's unique contributions to the body's functions and responses.
The Computational Backbone of Single-Cell Omics
Computational methods are the backbone of single-cell omics, essential for making sense of the vast and complex data this technology generates. Without robust computational and data analysis tools, the rich data from single-cell analysis would be like a treasure trove locked away in a chest. These methods unlock the chest, allowing us to process and analyze the omics database to extract meaningful biological insights.
Computational techniques range from data normalization, to adjusting the data for cell variability, to complex algorithms for identifying patterns and relationships within the data. For instance, clustering algorithms can group similar cells together, revealing the different cell types present in a sample.
We also rely on dimensionality reduction methods, which help simplify the data without losing critical information, making it easier to visualize and understand. These methods are crucial for mapping out the cellular landscape in a way that's both comprehensive and comprehensible.
Computational methods streamline single-cell data analysis, converting complexity into actionable scientific insights.
Tools of the Trade
The software and tools used in single-cell omics are as vital as the laboratory equipment. Currently, we're seeing a lot of development in software tools designed explicitly for single-cell analysis. These specialized tools are engineered to handle the unique challenges of single-cell data, from managing its volume to interpreting its complexity.
Commonly used software includes Seurat for single-cell RNA sequencing data analysis, which allows us to identify and characterize cell types and states. Another is Scanpy, a scalable toolkit for analyzing single-cell gene expression data. These tools mesh seamlessly with our research workflows, enabling us to process and analyze data efficiently.
Integrating the tools into daily work is now standard practice, allowing researchers to focus on biological questions instead of data processing complexities. They are the workhorses behind the scenes, turning raw data into insights that drive the research forward.
Computational Challenges in Single-Cell Omics
The computational landscape of single-cell omics is as rich as it is challenging. The amount of data we deal with is massive, and making sense of it is critical. Handling the sheer volume of data generated by single-cell techniques requires sophisticated data analysis tools and strategies to store, process, and interpret effectively.
Beyond volume, the complexity and heterogeneity of the data add layers of difficulty. Each cell has its own story, told through a unique combination of genetic and molecular information. Unravelling these stories to understand the broader narrative of cellular function and interaction demands advanced computational approaches. We need algorithms that can manage this diversity and learn from it to predict and model cellular behavior.
Developing these robust algorithms is intricate work. They must be sensitive enough to detect subtle nuances, yet powerful enough to handle large-scale analyses. A balance is crucial for accurate and reliable single-cell analysis, and creating precise and efficient algorithms is an ongoing challenge.
Moreover, integrating data from different omics layers—such as genomics, transcriptomics, and proteomics—compounds the complexity. Each layer offers a different perspective on the cell's function and requires a different computational approach. Integrating these layers into a cohesive analysis is like assembling a multidimensional puzzle; each piece must fit perfectly to complete the picture.
These computational challenges are significant, but they are not insurmountable. With each advancement, we move closer to fully realizing the potential of single-cell omics to uncover the mysteries of cellular life.
Impact on Research and Development
Computational delays can significantly slow down research progress. When we hit a computational bottleneck, it doesn't just delay our data analysis but our entire research timeline. The speed at which we can process and analyze data directly impacts how quickly we can make discoveries and develop new treatments for real-world applications.
Accuracy and reliability in computational analysis are non-negotiable. They are the bedrock of our research. If our computational methods are flawed, it could lead to incorrect conclusions, as I've stressed in previous blogs. Ensuring that our analysis is precise is essential for the credibility and utility of our findings in the real world.
Computational delays are more than just a minor inconvenience; they create a domino effect that hampers the pace of research and development. Every hour we spend troubleshooting computational issues is an hour not spent on discovery. The speed of our computational analysis dictates how swiftly we can transition from data to discovery, and, ultimately, to real-world applications.
Moreover, the accuracy and reliability of our computational analysis underpin the entire research process, especially when dealing with gene expression data. We must be able to trust the data, and the data must tell the true story. Ensuring the precision of our computational work is paramount, as it directly influences the validity of our research outcomes.
Paving the Way Forward: Solutions and Strategies
Innovation is key to navigating the computational complexities of single-cell omics. We're crafting algorithms that can digest large-scale data and deliver precise insights. Algorithm innovations are pivotal for maximizing the potential of single-cell research by effectively managing a vast omics database.
Collaboration and standardization are also vital in pushing the boundaries of what we can achieve. By standardizing methods and tools and working together, we're setting new benchmarks for what's possible. This sentiment underscores the collective effort in our field. The shared approach ensures consistency and quality in our work, facilitating advancements and fostering a collaborative scientific community.
Moreover, cloud computing and parallel processing technologies are game-changers, offering the computational horsepower we need. They allow us to process larger datasets more efficiently and with greater speed, significantly reducing the time from experimentation to insight. Embracing these technologies is essential for our progress in single-cell omics research.
Real-World Applications and the Future
Overcoming computational hurdles in single-cell omics has led to real-world breakthroughs. We've seen case studies wherein, data management resulted in identifying new drug targets. These successes showcase the tangible benefits of our computational strides.
Looking ahead, the advancements in computational omics hold immense promise for medicine and environmental science. Imagine tailoring treatments to individual cellular profiles or monitoring ecosystems at a cellular level. The potential is vast, with the power to personalize healthcare and protect our environment through more informed decisions.
Conclusion
Addressing computational challenges is crucial for the advancement of single-cell omics. We must tackle these issues head-on to unlock the full potential of our research. The path forward requires persistent innovation and a collaborative spirit within the scientific community.
I urge my colleagues and the broader field to continue pushing the boundaries of what our computational methods can achieve. Together, we can turn the tide of these challenges into opportunities for ground-breaking discoveries that can transform both science and society. Let's join forces to shape the future of single-cell omics.
Mark Kunitomi
Mark Kunitomi is the Chief Scientific Officer at Almaden Genomics. He was a post-doctoral fellow at UC San Francisco with a background in genomics, bioinformatics and microbiology, and he has a Ph.D. in Biochemistry & Molecular Biology from the University of California, San Francisco.