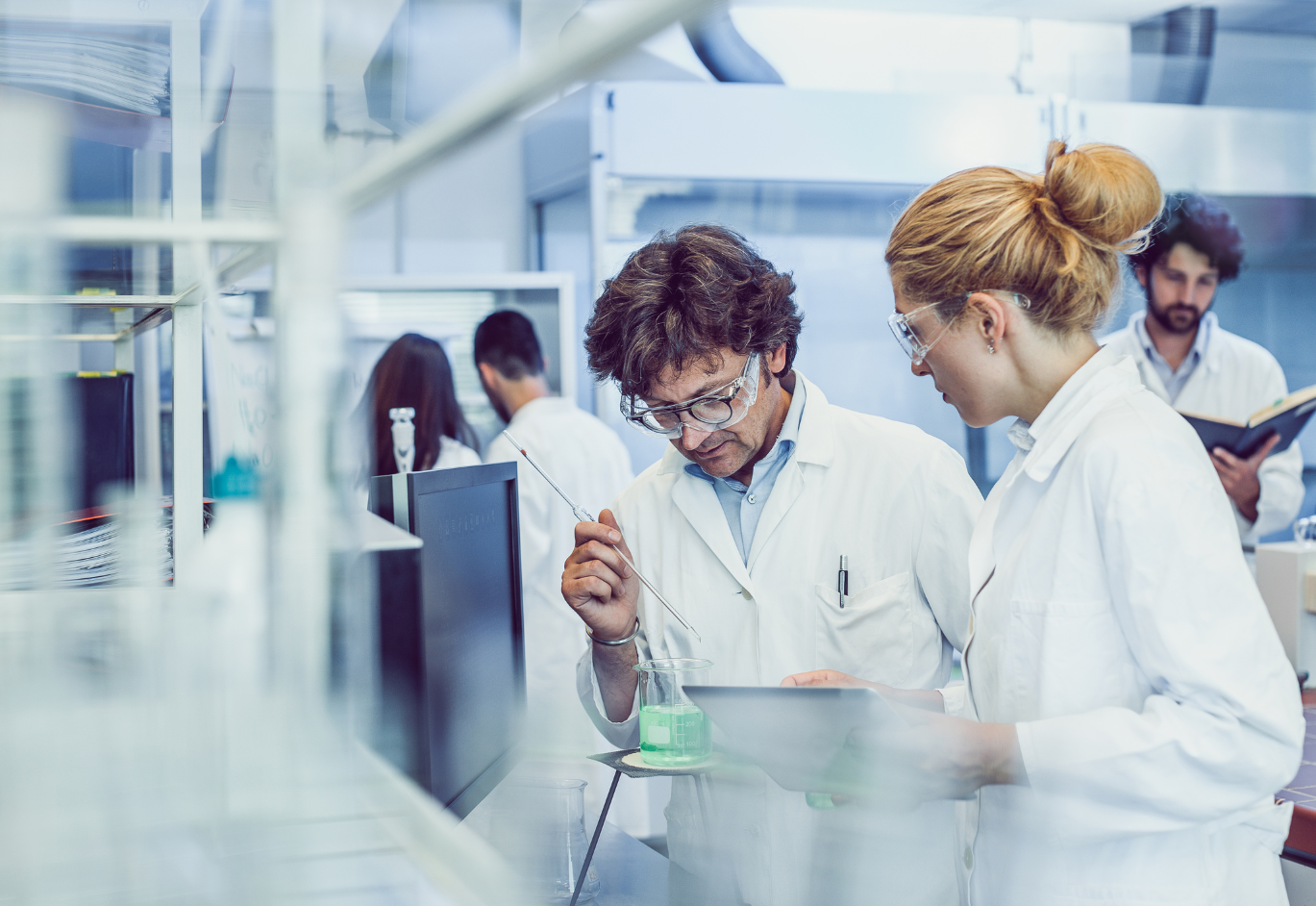
How to Solve the Biological Research Reproducibility Problem
Scientific research holds the keys to a brighter future. Ideation and innovation are rapidly bringing us closer to breakthroughs in personalized medicine, new cancer therapies, and other genomic discoveries. In the relentless search for scientific advancement, biotech leaders are poised to shape both, the future of their organizations and the trajectory of scientific progress.
When moving from the laboratory bench to the printed (or digital) page, scientific leaders face a less lofty, though no less significant, challenge—reproducibility. Here countless experimentation hours get translated into actionable therapeutics strategies. And if these steps are wasted if not taken to ensure efficiency, accuracy, and transparency.
This article explores reproducibility's role in bioinformatics analysis, challenges faced by researchers, and solutions that leaders can implement to ensure reproducibility in their teams’ work.
The Role of Reproducibility
Reproducibility is a fundamental principle of scientific research. Unless other scientists can reproduce the results of a study, there’s no way to verify the findings, detect errors, or build on previous work. Good science is reproducible.
Beyond ensuring scientific accuracy, though, the importance of reproducibility also has to do with ensuring the transparency of a particular line of research. Simply from reading a published study, researchers should be able to re-create experiments, generate the same results, and arrive at the same conclusions. However, too often scientific findings in biomedical research cannot be reproduced. This harms the credibility of particular publications, their authors, as well as the scientific community as a whole.
While studies showing meaningful results are more likely to get published, the problem of reproducibility looms large, becoming a fierce debate in the scientific community. In one frequently-cited report, the Reproducibility Project: Cancer Biology, researchers set out to reproduce 193 experiments from 53 high-profile papers published between 2010 and 2012.
The results were not encouraging. Organizers reported issues such as:
- Effect sizes for cancer biology papers were (on average) 85% smaller than reported in the original papers
- Original negative results replicated 80% of the time, but original positive results replicated only 40% of the time
- Overall, slightly fewer than half of the effects evaluated replicated on more criteria than they failed on
- Only five of the 53 papers had results that could be fully reproduced
Barriers to conducting replications – by experiment. During the design phase of the project the 193 experiments selected for replication were coded according to six criteria: availability and sharing of data; reporting of statistical analysis (i.e., did the paper describe the tests used in statistical analysis?; if such tests were not used, did the paper report on biological variation (e.g., graph reporting error bars) or representative images?); availability and sharing of analytic code; did the original authors offer to share key reagents?; what level of protocol clarifications were needed from the original authors?; how helpful were the responses to those requests? The 29 Registered Reports published by the project included protocols for 87 experiments, and these experiments were coded according to three criteria: were reagents shared by the original authors?; did the replication authors have to make modifications to the protocol?; were these modifications implemented? A total of 50 experiments were completed. Image, caption source: Errington, T. M., Denis, A., Perfito, N., Iorns, E. & Nosek, B. A. Challenges for assessing replicability in preclinical cancer biology. eLife 10, e67995 (2021). Creative Commons license |
Examples like these impact already tight budgets at biotech firms in particular. Although the problem of reproducibility is difficult to quantify, a 2015 meta-analysis regarding the cost of non-reproducible research estimates that in the U.S. alone, $28Bn per year is spent on preclinical research that is not reproducible.
Next, let’s turn to some challenges and solutions in the area of bioinformatics analysis.
Reproducibility Challenges with Bioinformatic Analysis
Reproducible research failures can’t be traced back to a single cause as there are multiple obstacles, variables, and categories of shortcomings. These can explain the non-reproducibility reason for the research in the majority of the cases.
1. Inadequate access to raw data, methodological details, and research materials
To reproduce published work, scientists must have access to the original data, protocols, and critical research materials. Otherwise, researchers are forced to make educated guesses or reinvent the wheel as they attempt to repeat results. Each time an unknown is introduced into the experiment, the chance of reproducibility diminishes.
Sharing information among scientists is difficult as the tools, mechanisms, and systems available for housing raw unpublished data are highly complex and hard to use. Without access to the right information, failures of reproducibility are inevitable.
2. Inability to analyze, interpret, and manage complex datasets
Compounding the difficulties with the tools designed for housing raw unpublished data is the challenge of managing increasingly complex datasets. As advancements in technology enable the generation of massive amounts of data, researchers need tools for analyzing, interpreting, and managing this information. Often these tools can’t keep up.
Also, with limited integration of many tools with internal software and data processing systems, teams must rely on external infrastructure and expertise to analyze results. This may mean that scientists need to learn code or other skills to understand bioinformatics analyses. Such external collaborations are time-consuming, frustrating, and, in many cases, do not yield the information required to meet high publication standards.
3. Use of misidentified, cross-contaminated, or over-passaged cell lines and microorganisms
When biological materials cannot be properly identified, maintained, or authenticated, reproducibility is impeded. For example, if a cell line is not correctly identified, or has been contaminated by mycoplasma or another cell type, this can affect results. Researchers must be vigilant for questionable results and potentially invalid conclusions due to the widespread use of cross-contaminated cell lines in experiments and scientific publications.
In addition, serial passaging due to improper maintenance of biological materials can affect genotype and phenotype. Several studies showed that this can cause gene expression changes, growth rate, spreading, and migration in cell lines—factors hindering the way of reproducing data.
Biologists may rely on past successes when they are unfamiliar with the complexities of data analysis, other pitfalls, and how it can affect their research. However, this is the equivalent of burying our heads in the sand. We can do better.
The creation, distribution, and literature of a cell line: A cultured sample of cells (blue cells) may produce an immortal cell line (red cells), sometimes announced in ‘an establishing paper’ (in white). Cells may then be distributed to other researchers and reported in research papers, the ‘primary literature’. If misidentification of cells is reported in ‘a notifying paper’ (in red, bottom left), this may raise questions about the entire cell line (question marks) and the papers based on it, since misidentification commonly occurs at the source. Notifying papers should be reported to ICLAC, which will decide whether cell lines should be added to the ICLAC misidentified cell line register. Meanwhile, the contaminated primary literature is cited (dotted lines) by ‘secondary literature’, spreading the contamination further. Image, caption source: Horbach, S. P. J. M. & Halffman, W. The ghosts of HeLa: How cell line misidentification contaminates the scientific literature. PLOS ONE 12, e0186281 (2017). Creative Commons license |
Strategies for Achieving and Maintaining Reproducibility
Initiatives like the Reproducibility Project mentioned earlier have done much to shed light on the lack of reproducibility in scientific research. As a result, a set of recommended practices and policy changes have emerged.
Best Practices
For biologists looking to implement reproducible workflows including bioinformatics analytics, there are a number of best practices to follow:
Robust sharing of data, information, materials, software, and other tools: All of the raw data underlying any study should be readily available to researchers and publication reviewers. Asking researchers to add their data to public datasets would increase reproducibility and help scientists to interact and collaborate at a higher level.
Use of authenticated, low-passage biomaterials: If cell lines and microorganisms are verified by a comprehensive system evaluating phenotypic and genotypic traits, as well as contaminants, reproducibility would be improved. By routinely evaluating biomaterials throughout the research workflow, findings and results would be more reliable.
A thorough description of methods: Reproducibility will be improved when research methods are thoroughly described and authors are available to discuss their methods. Researchers should clearly report key experimental parameters, such as whether experiments were blinded, precisely which standard and instruments were used, how many replicates were made, how the results were interpreted, how the statistical analysis was performed, how the randomization was done, and what criteria were used to include or exclude any data.
Tools and Techniques
To help researchers succeed with the best practices above, there are also new tools and techniques designed to aid in version control, containerization, and workflow documentation. These scientific research tools allow researchers to focus on the science.
One way these tools improve reproducibility is by streamlining the sharing of data, information, and research materials. For example, in many labs, collaboration between biologists and bioinformaticians can be time-consuming and frustrating. Often to understand bioinformatics analysis, biologists need to have extensive coding experience, a skillset that most biologists don’t have. This gap in knowledge makes robust sharing of information nearly impossible, which in turn leads to delayed insights and a lack of reproducibility.
For insights and innovations in biology to move smoothly from bench to publication, biologists and bioinformaticians need no code/ low code tools that are user-friendly. g.nome® by Almaden Genomics is one such tool.
Labs using this platform accelerate discovery and increase transparency to improve reproducibility. The Corey Lab at UT Southwestern Medical Center used g.nome to create transparent workflows, which allowed teams and scientists to easily share information and track results. By allowing for containerization within pipelines, g.nome provided the Corey lab with version control and stability for each lab member. Also, the comprehensive logging of tool versions, functions, and parameters ensured that the team could trace their findings and correct for any potential variabilities. g.nome gave Corey Lab researchers more confidence in their results.
Conclusion
Without reproducibility, scientific discovery would not be possible. Researchers and leaders owe it to themselves, their teams, and the scientific community to maintain a commitment to improving transparency throughout from bench to publication. By enabling scientists to follow the best practices outlined above, new tools pave the way for improved reproducibility.