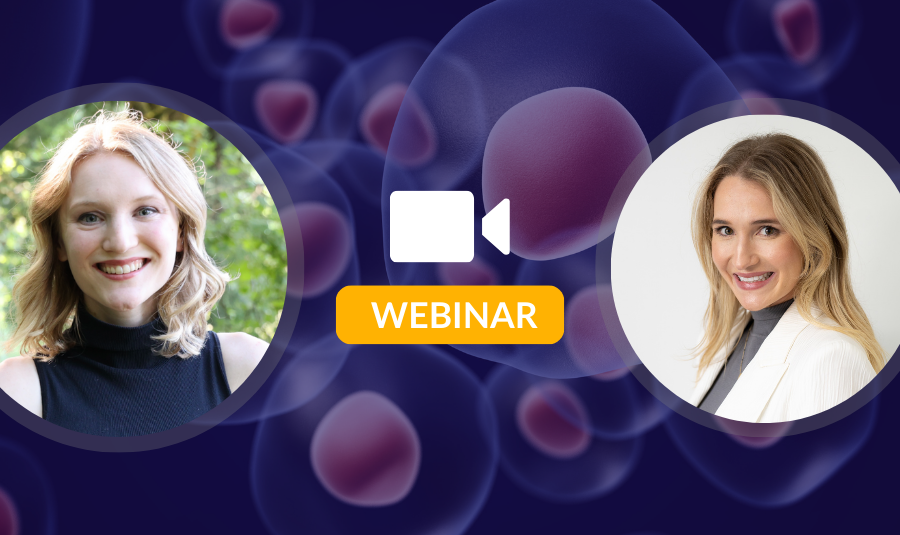
WEBINAR: Visualize & Annotate Single-Cell Data with g.nome®
In the ever-evolving landscape of genomics, the analysis of single-cell data has become increasingly pivotal. This webinar is designed to empower genomic researchers by providing essential skills for seamlessly navigating single-cell analysis using g.nome®, no coding capabilities needed. The g.nome® platform offers a user-friendly graphical interface, ensuring accessibility for researchers of all backgrounds. Watch to gain practical insights on how to leverage the power of single-cell analysis and to unlock valuable genomic information so that you can stay at the forefront of genomics with confidence and ease.
Key webinar highlights:
- Data Processing Made Easy: Learn to process raw sequencing files or pre-processed data, such as Seurat objects, and efficiently filter out and remove poor-quality data for robust analysis.
- Visualize with Precision: Explore the art of data visualization using techniques like UMAP, t-SNE plot, and violin plots. Gain insights into annotating cell clusters for a comprehensive understanding of your data.
- Differential Gene Expression (DGE) Analysis: Discover how to compare differential gene expression between different conditions, unlocking valuable insights.
Webinar Transcript
Hannah Schuyler:
Great. Well, I'm going to kick this off. Thank you everyone so much for joining our webinar on visualizing and annotating single cell data with g.nome. My name is Hanna Schuyler. I am a director of business development here at Almadem Genomics, and we also have our in house single cell expert Nora Kearns joining us as well. So thank you so much and excited to be talking through some of these capabilities with you today.
Just a few quick things before we get started. This webinar is being recorded and will be sent out to all attendees. So thank you for joining, and then please enter any questions that you have for the presenters in the chat box, and they will be addressed at the end during the live QA session. So definitely want to make sure that we get those addressed and type those in the question box for us.
So what will we be discussing today? We have a few things that we'll be covering first I'll go over an overview of Almaden Genomics. So who we are, where we came from, and how we're helping a variety of our different clientele. Analyzing genomic data. Then we will talk through how you can actually visualize and annotate your single cell data with our guided workflows. Allowing you to go from raw data to processed without having to use code. We'll leave some time for live QA at the end to go through any questions that you guys have. And then, lastly, we'll be talking through next steps on those that are interested in testing and piloting the software as well.
Just to give you guys a little bit of context in terms of who we are. We are a spin-off company from IBM Research Center in Almaden California, and the software was originally built as an internal need for pet and food safety and the team quickly saw that there was a need for building out these pipelines internally. Especially for those that did not know code and so that's how g.nome was born. And now we work with a variety of different clientele, including pharmaceutical clients, academic clients, biotech clients to help them with their data analysis. Whether that's using some of our pre-built workflows that we're going to talk through today. Access to curated data sets from some of our partners, as well as bioinformatics, consulting services as well.
The goal here at Almaden Genomics really is to help streamline the collaboration between the data scientists and the biologists. It's a very complex space, as I'm sure many of you know. The biologist has a goal of analyzing their own data, whether it's in house sequencing data or public data. And their biggest priority is really creating visualizations and making sense of that data. And it's possible that they may not know code so having to build a pipeline might be a little bit scary for them, and so they often are relying on bioinformaticians to do this analysis for them. This can be a time-consuming process. There's not necessarily a common language between both parties and it's not necessarily an easy process. And then, of course, on the other side of the coin we have the bioinformatician. Their main priority is building pipelines, workflows, leveraging open-source tools, and really being tasked with helping the biologists analyze their data. And so our goal here really is to help kind of streamline that process and bring the collaboration between both of those 2 teams.
And so of course, today's webinar is focused on single cell. And I think it's important we touch on why this type of analysis is so important. And before I jump into some of the reasons why single cell is so important, I'd like to host a poll and see how maybe you're actually working with single cell data right now. So, in just a second, you should see a poll pop up. So are you working with single cell data now, or planning to do so in the near future? Go ahead and answer if you see that come up on your screen. So yes, I am working with single cell data now. Not yet, but I'm planning to in the near future. Or no I'm curious to learn more. Awesome. It looks like we kind of have a combination of those that are working with single cell data, and some that are interested to learn more. So, thank you so much and I think this is a perfect transition as to kind of why single cell is so important. I'm sure many of you have worked with bulk RNA-seq data in the past and what's so powerful about single cell analysis is that you're able to uncover insights at the cellular level versus an average of what is happening in all of the cells which is typical of bulk RNA-seq, right. And so, with single cell analysis, you have the ability to analyze the gene expression profiles of individual cells which can also reveal cellular heterogeneity and rare cell populations that might be masked when you're looking at your bulk, RNA-seq data. And then, lastly, single cell analysis have really been critical in furthering scientific discoveries. Some of those may include how to predict how a disease may evolve over time, uncovering the mechanism of action for different drugs, or even discovering new cell types.
So while single cell sequencing is very transformative with a lot of potential. There's several challenges that we hear from researchers that they face when it comes to analyzing these complex data sets. And the first being is that these single cell data sets are typically quite large, making storage and processing very computationally intensive. And so this means that there's longer computation times for the researchers as well as potentially higher costs in actually analyzing this data. There's also a lack of standardization or data analysis as it relates to single cell. Over the years we've seen so many different platforms, open source tools, technologies that have been deployed to process single cell data which, of course, complicates any sort of cross study comparisons, data integration or standardization as you're looking to process these different types of data sets. And then, lastly, being able to accurately identify and annotate cell types is a very time consuming process, which I'm sure if you are working with single cell data, you're very familiar, that this is sometimes a tedious process. And so clustering of the cells really doesn't mean anything if you aren't able to identify and annotate what those clusters are. So there's typically 2 different approaches. Researchers either must do this manually to identify cell types meaning they would have to know the marker genes associated with their cell types or use some sort of automation. The catch with the automation, of course, is that you need to have some sort of training data set or reference data set and, as you can imagine, both of these types, or I guess, approaches have their own challenges. You either are unsure of what you're looking for in the cell types, or you lack a strong reference. So it's a common challenge that researchers face quite frequently.
And so the goal here, really, with our software g.nome is to help reduce some of those complexities for researchers with our pre-built workflows that can be used by biologists, data scientists and bioinformaticians. There's many different ways you can actually bring in different types of single cell data. So one of those options is the ability to pull in public data from our SRA integration and run it through our SRA tool. So accessing any of those public data sets, can be processed on our software. You can also process in-house sequencing data files. So if you're pulling that from a local server or an S3 bucket, and then you also have the option to really process that data at any point in the journey. So whether that's starting with a FASTQ file an HDF5 file, or even a filter gene barcode matrix file. And then we're also working with data partners to bring in those curated data sets. So you can process, analyze and visualize those data sets on our software as well.
And the goal is that you would start with a pre-built workflow, right? So we're using SRA or start solo go from the raw data files to those meaningful visualizations. But one of the benefits about this opera is that you can actually do a variety of different custom modifications to the workflow. So you have the option to modify parameters. You could do batch correction, adjust normalization, evaluate cell cycle genes and even filter out cells. And then with this guided workflow that Nora is going to talk us through in just a bit. You also can perform cell type annotation with access to over 28 different reference data sets. So this again helps kind of cut down on that time to actually annotating the cell clusters for you. And then, lastly, we also produce interactive reports and visualization. So you get UMAP’s, violin plots, cell counts, top genes. All of this can be imported into Jupiter notebooks as well to take kind of one extra layer in terms of making it more customized, based on what you guys are specifically looking for. And so with that I would love to pass this over to Nora, to give a more formal walkthrough of how you can actually set up a guided workflow on our software, and how this will translate into visualizations and interpreting of your single cell data.
Nora Kearns:
Great. Thank you. Hannah. Okay. Screen sharing good
Hannah Schuyler:
Screen sharing. Good.
Nora Kearns:
Awesome. Okay? So today, I'm going to talk to you a bit about the g.nome software. But really what I want to talk to you about is how I approach the analysis of single cell data, some interesting insights that we can gain from single cell data. And you know, homing in on the whole meaning of the webinar, which is visualizing and annotating single cell data. So just to start when you enter g.nome, this is your project management space. So for each analysis that you are performing, you'd want to create a project. I have already done this cooking show style. So I'm just going to dive into this project we have here. And this is our project management page. So we can, you know, see the cost of our project, manage our project, change metadata, things of that nature or delete the project. Okay so, when we're running a single cell analysis, the first thing that we have to think about is how we're going to get our data from point A to point B, this is a problem that bioinformaticians experience all the time, because bioinformatics data tends to be very large. You might have files that are 10 to 20 GB. And so it can actually be difficult to, you know, download your data and then upload it somewhere else. Those are challenges that that Bioinformaticians commonly run into. So on g.nome we have 2 ways of helping you with that challenge. The first is that we can configure setup to an S3 bucket if you're using S3, so you can import your data directly from that S3 bucket. You can also import files locally using or from your local machine using a high speed file transfer tool called Aspera. So either those are options to help you get your data on platform.
I've already imported a data set that we're going to work with today. But once I have my data in the in the right project space, then I get to start thinking about running my analysis. The g.nome workspace can kind of be thought of from 2 perspectives. And it's meant to support 2 types of scientists. So one is the scientist who, you know, has the biological know-how, they know how to set up experiments, prep sequencing libraries and run that data. But they may not have the coding expertise to perform analysis, you know, with code. And then we also have the bioinformatician who does have a bit more expertise on how to set up these workflows. And this tool or genome is meant to support both of those kinds of scientists. But I first want to talk about the guided workflows because those are our workflows that are really meant to support the biologists. So I'm going to run a guided workflow.
We have guided workflows available for both RNA-seq and single cell. But I'm obviously going to focus on single cell today. So I will select that workflow. I can label the run however I want to. And then we have to select our input data type. So when you are performing single cell analysis, you may be starting from 2 different points. You could be starting with raw sequencing data. If you've done the experiment yourself and you want to analyze it, so that would be in FASTQ format. Or you may be starting from the point of account matrix. So possibly you've gone out and looked through papers. You found some interesting data hosted on Geo. You want to pull that in and try to reproduce their analysis or gain additional insights from someone else's public data. So we have the ability to start from either point. But for today's webinar, we'll talk about FASTQ data.
So, from my data bucket, I would select my data set just takes a second to load. There we go. So, I have this PBMC 1K FASTQ dataset with all of my files contained within it. Then I'm going to select a sequencing chemistry. So we support 4 different chemistries, 10 x 3 prime, v. 2 and v. 3, and then 10 x 5 prime paired end and R. 2 only.
Then I need to pair my fastQs. So this is essentially creating a sample sheet. And the important functionality about this is that it ultimately is what parallelizes the processing of your data. So you might have 5, 6, 10 samples and you want to make sure that those samples are processed in parallel, all at the same time, rather than painstakingly going through and processing each sample individually. So this tool is essentially taking care of that for you. And I'm going to leave the sample names as default but you also have the ability to go in and change those to whatever you want them to be. So, once we paired our fast queues, we need to create a metadata file to associate samples with important biological characteristics. So you may have a sample metadata file that you can import, or you can manually create a metadata file. So I'm going to just have an experimental group and a control group, and then associate each sample with its correct condition.
Then I want to select my reference genome. On g.nome we supply the GRCH38, so human reference genome, and the mm10 mouse genome. These are specifically configured by cell Ranger for cell ranger data. But if you are working with a non-human or mouse organism. Let's say you're working with rat or zebrafish. You can also supply a genome FASTQ file and a GTF file, and the workflow will generate your reference genome for you.
Okay, I want to take a quick pause here and talk about the steps that we've been through up to this point. So we have imported our FASTQ files, created a metadata file, and selected a genome. All of those steps are important for running the first part of single cell analysis, which is QC in alignment. QC means evaluating the quality of our sequencing data. So actually, how good was the imaging of your flow cells? How good is your data? And then alignment is aligning the RNA that was sequenced, that's in your FASTQ files, against a reference genome and counting the number of RNA transcripts observed from each gene. So it's generating the counts data. So everything up to this step has helped us generate the counts data or set us up to generate the counts data. Now we have to start thinking about the actual counts data, and the first step in doing that is filtering parameters.
So there are a few filtering parameters to think about. There's maximum RNA features per cell, minimum RNA features per cell, and Max mitochondrial DNA. So maximum RNA features per cell can help us eliminate doublets which are occurrences of multiple cells kind of stuck to each other that are observed as a single cell. So we're getting way way way way more counts than we should be getting if it was from a single cell, so we can set a maximum for that. The default is 10,000 as well as minimum RNA features per cell. If a cell is barely expressing anything, we probably want to exclude it. A good default value, for that is 200. And then, lastly, Max mitochondrial DNA. So you want to set a maximum mitochondrial DNA percentage, because mitochondrial or high quantities of mitochondrial DNA can be indicative of cell damage and cell stress. So we want to get rid of cells that have very high counts of that, generally a good range to go by is 5 to 20 percent PBMC's, which is the type of data in this example. You can typically go a bit higher. So around 15 to 20 percent. So that's the default value that we have set there. Then you want to just decide if you want to evaluate cell cycle genes. So at the end of a single cell analysis, you get these beautiful clusters, or hopefully beautiful clusters. And ideally, you want those cells to cluster together based on type. But there are lots of different reasons why cells may cluster together. Ultimately, clustering is a sign of similarity between cells. So there, you know, there are other reasons why cells may be similar, and one of them is that there are cells in the same stage of the cell cycle. So because they're expressing the same set of cell cycle genes, they are similar to each other, and they may cluster together. But we don't really want that to happen. So we can choose to evaluate and regress out the effect of cell cycle genes on our data. By default, the human cell cycle genes are pre-loaded in Seurat. But you can also choose to supply your own input S. Phase genes and G2M phase genes.
Okay, then we want to think about if we have multiple samples, do we want to integrate and correct for batch effects in those samples? So batch effect correction is correcting for technical covariation and the data that is the result of you know, technical setup in your sample prep. So, for example, you processed or prepped your first sample on Monday at 10 Am. And then you processed another sample, on Tuesday at 10 am. Or 2 different people prepared those individual samples that can create unwanted variation in the data that's solely the result of that technical reason. But it's not indicative of underlying biological difference in the data. So we want to prevent that from creating unwanted differences. So let's say, we do want to perform integration in batch effect correction. Then we have to decide, what algorithm do we want to use? Seurat by default chooses or uses CCA and CCA is a really good algorithm to use when you expect to see conserved cell types across your conditions. So the same cell types across your condition. But there may be significant shifts and expression that are the result of your experimental conditions or disease states like, you might have 2 different donors with 2 different types of breast cancer. It's also very good when you're integrating across modalities. Another option is to use RPCA, which is for reciprocal PCA. RPCA is great if you have a very large data set, because it is less computationally intensive and significantly faster than CCA. And it's also good when you expect to see differences in cell types across your sample so essentially prevents over correction and helps preserve biological variation in the data.
So let's go with CCA for these for these ones, because I anticipate seeing the same cell types. And then, lastly, we have to think about whether or not we want to perform cell type annotation on g.nome we support reference-based cell type annotation, which means that we have a data set of already annotated cells, and we are going to compare it to your unannotated cells, and try to find similarities to in the expression of annotated versus unannotated cells, to assign annotations to your cells. So we use a tool called single R for that, single R examines a reference data set and assigns annotations to your cells based on that.
The reference data sets that we have available. There are 28 of them hosted by the Celldex Library and the scRNA-seq Library cell. Celldex hosts 7 well validated data sets that are generally compiled from individual bulk, RNA-seq data sets. So they took a single cell type, ran bulk RNA-seq analysis on those cell types to establish an expression profile and then compile them. So these are really strong reference data sets, but they are a bit skewed towards immune data. So they're great if you're working with PBMC's, for example. But if you're not, we also have the data sets available from SCRN-seq. These are from individual publications. But they do have more diverse tissue types represented, which is great. And our data sets do span human and mouse. So let's say, I just go with the cell decks Monaco immune data. Go with that, click next, and then I see a summary of the run that I am about to launch, so I would just click launch. I've already done that, and I have a run ready for us to view. I'll click launch on my run and then it will take me back to this guided workflow page. That shows me the runs that I have previously done. So I'm going to go in and view the results of this run that I did yesterday and so I have all my results divided into individual directories, indicating what the results contained in them are. So we'll go into this HTML report directory and then I would just download the report and open it up. Oh, sorry over here.
So during the single cell workflow. There are multiple steps that happen. There's filtering, there's identifying variable features, running or generating a UMAP. And each of those steps produces visualizations. And essentially, what we've done here is we have compiled all of those visualizations into a single report that allows you to download it and share it across. You know your team, for example. So let's dive in to some of these visualizations and talk about you know what's meaningful about them. What kind of insights we can gain from this data.
So the most interesting thing that you get at the end of single cell analysis is your beautiful UMAP or hopefully beautiful, or a tSNE plot. What we show you here are the annotated cell types. If you perform cell type annotation as well as how Seurat identified your clusters. And this can be kind of interesting, because Seurat may find further differentiation between specific groups of cells than your annotations would indicate. So it's just kind of 2 perspectives of looking at your TSNE. We also show you cell counts. So the number of cells observed in each cluster. And this can be interesting if you're comparing 2 different groups. Do I see you know way more mono sites in one sample than I see in another? As well as the top genes expressed from each cluster. Here, we're just seeing the top 10, but you can change it to observe the top 100 or top 50. However, you want to do that.
And then, lastly, this interactive gene visualization. So this is what helps you look at differential expression of genes across your clusters. So for example, here I see this CD 6 gene is really strongly expressed in this group of let's see, these are these are T cells. I see that that's really strongly expressed in this group of cells. If things,or if your clusters are not what you expected them to be. Then you can kind of take a look at your QC and see you know, maybe my data isn't super high quality. Or maybe my filtering wasn't great. How can I adjust things to get closer to what I would expect to see? So we show you your QC results. You see here the distribution of total transcript counts in each cell, the distribution of genes observed in each cell in the center here, and then the distribution of mitochondrial DNA. We also show, this is essentially the same data in a different format. So you know, RNA transcripts on the X axis here versus mitochondrial DNA, or number of unique genes versus transcript counts. This perspective of the data can be really interesting because it can help you identify patterns of quality in your data. So this data actually looks pretty good. You generally want to see kind of a nice tight line here. But let's say that you had, you know, 2 different lines that were kind of shooting out on this plot. That may show you that. Oh, there's some kind of technical thing going on in my data, where one group is higher quality than the next. So just helps you kind of learn more about the quality of your data. We also show a variable feature plot. So the top variably expressed genes within each sample. And then, lastly, an annotation QC plot. So you can see there's a lot of annotations here, lots of things on the X-axis. But basically this delta distribution plot is showing the difference in confidence between the first place annotation assigned to each score and the second place annotation. So you would want the Delta to be high, because that would mean there's a very clear winner in the annotation assigned to yourself.
So on that subject I want to dive a bit deeper into the subject of annotation. 1 second, I'm trying to move this screen over. There we go. Okay. So when we're performing cell type annotation, we want you to think of running analysis of single cell on g.nome, as being very similar to running an experiment at the bench, so you may get to the point of clustering of your cells, and you see that those clusters are not really what you'd expect. You know you're not. They're not clustering well. So there's a lot of things that you can adjust, and that's totally normal. And except it's not like you are, you know, fudging the data at all, you need to try some different filtering parameters. Maybe the first time you filtered your data wasn't the best. You need to go back and adjust some things, that's very normal in the processing of single cell data. And, in fact, many people say that you should expect to spend about 80% of your time in single cell analysis in this space of trying a few different parameters out and getting to the point of what you, as a biologist, would expect to see in your data.
I’m just going to show you how I annotated the single cell data using 2 different reference data sets. So this is the same tSNE annotated with the human primary cell Atlas and the Monaco immune data set. So let's dive into what we're looking at here. We have this tSNE, and it's broken out into monocytes, B cells, NK cells and T cells. How do we know that those annotations were actually good annotations? So this heat map on the right here is how we would evaluate that. So what we're seeing in this plot is that each of these individual little columns like these stripes is representing a single cell and the colored bars across the top are showing the groupings of cells. So this yellow bar is showing the B cells. This blue bar is showing the Monocytes and you can see that the kind of sizes of these chunks of yellow are corresponding to the approximate sizes, a number of cells that we're observing on the UMAP. And then down the right hand side of this heat map are the all the possible annotations. Essentially. So let's talk specifically about this group of monocytes here. Basically, the algorithm was very confident that these cells were monocytes, because in the monocyte label, it's yellow. So it's very high confidence in the annotation. But as we go down the possible annotations of CD 4 positive T cells, CD8 positive T cells, B cells, and NK cells, it's blue. So that meant the algorithm does not think that it is any of those cell types. In contrast, the T cells. There's a bit more fuzziness here. So the algorithm was pretty confident that a single cell was a T cell. But it was also uncertain it might be a CD8 positive T Cell, as opposed to CD4 positive T Cell. And this is to be expected, because within a certain cell type, more genes are, the expression genes is largely shared. There's just a few genes that are differentiating the 2 cells from each other. So you'd expect to see that the algorithm has a bit harder time discerning within a specific cell type.
In contrast, here is the Monaco immune data set. So this is nice because we get really high resolution or granularity of cell types in the annotation. So we can differentiate between non switched memory B cells and switched memory B cells. And that's really interesting to see. But as you can imagine, it introduces a lot less or a lot more ambiguity, and less confidence in the exact cell type. So within a certain cell type, you can see like this, this group of cells, we're pretty confident is monocytes. But is it a classical monocyte? Is it an intermediate monocyte or a non-classical monocyte? Then it becomes harder to differentiate. But this is an example of the kind of iteration that you can do on g.nome, and that's something that we want to support, and one specific characteristic that we use to support that is cloning our runs. So we don't want you to ever have to go all the way back to the beginning of your analysis and run it all the way to the end because cell type annotation is kind of the last step you perform. You can just clone your run and run only this step on you know, 2 or 3 different data sets until you see what you're kind of expecting to see.
The next thing that I want to talk about is batch effect correction and data integration. Which is also really important into gaining confident insights into your data. So here's an example of 2 different breast cancer tumors. One of these tumors is HER2 positive and estrogen receptor positive. The other one is just HER2 positive. So we have each individual sample processed without batch effect correction on the left here, and then we have the data batch effect corrected and integrated on the right. If I were looking at these unintegrated samples, I would observe, okay, I have these, like 2 large groups of cells across the samples, and they may be really similar. But I'm not sure. But once I run batch that correction, I can say with confidence, Okay, these are the same groups of cells across my 2 samples. So these are really similar. But I am starting to see some interesting differentiation across the 2 samples. For example, this cluster here is largely observed in the in or this cluster of cells here is dominant in the HER2 positive tumor and you know, this cluster of cells here is mostly observed in the HER2 positive ER tumor.
Once we've done that batch effect correction and integration, we can it annotate ourselves and start visualizing how different genes are expressed differently in the 2 tumors. So just as an example of this, I've taken a gene, SFRP 2, and visualized it across the 2 different tumors and I see that one there's higher across the board expression of that gene. But particularly this group of cells here strongly expressing SFRP2. Compared to kind of weak expression in this other tumor. This group of cells is a set of cancer associated fibroblasts, and this is consistent with what we would expect to see, because SFRP 2 is a marker gene of CAF’s. CAF's are interesting because they can promote tumor aggressiveness and so this helps us understand, you know, why might this person be responding better to treatment or worse to treatment. Why might this person's tumor be growing more aggressively than another person's tumor? This is the kind of meaningful insight that single cell data can help us gain.
Okay so that is everything that I wanted to show about the guided workflows and the outputs of single cell data going as a step deeper. You know the guided workflows, the goal there is to one empower biologist to run analysis with autonomy. You don't have to wait on a core to do your analysis for you or wait on a data scientist who might have, you know, 15 different projects going on. You have the power to run that analysis yourself, but we also acknowledge it. Acknowledge that you're scientists by nature, and you are curious, and you want to know exactly what's going on in this kind of analysis even though the goal of the guided workflow is to eliminate the complexity. But at the end of the day you might want to see that complexity. You might want the ability to fine tune and adjust your analysis. So that's what the canvas view allows you to do. And this is also a nice view for the bioinformatician who wants to know what kind of tools and parameters are being used.
So in the canvas workflow we have 4 different plug and play tools. So QC and alignment analysis, cell type annotation and then complete single cell RNA-seq, which runs all 3 of those in sequence.
To dive in to one in particular. This is the plug and play version of the single cell analysis workflow. So it has the same kind of input parameters that the guided workflow had, such as whether or not you want to evaluate cell cycle genes or run a UMAP, run a tSNE. But there is a bit more fine-tuned control here, and if you want to see, you want to have that control, and you want to see what's going on under the hood. We can click this sub workflow button and it will pop us out to the actual workflow that's being run. Okay. And so now we can see the individual tools that are running in sequence for this workflow. So we have a Seurat pre-processing tool, Seurat normalization, Seurat find variable features, Seurat cell scoring. So lots of Seurat and the whole workflow is powered by Seurat. So if you are bioinformatician, and you're familiar with the Seurat workflow. Basically, each of these tiles is a graphical representation of a Seurat tool. All of the inputs that are exposed in that tile are the same as the inputs for that Seurat tool. So you have the ability to go in, and I've just done this here, normally, the Seurat find variable features algorithm returns the top 2,000 most variably expressed genes in each sample. Here I just changed it to a thousand. That's just a random example of how you could go in and change individual parameters in your analysis.
So basically, by drilling down to this level, you, you know, can see exactly what's going on, and also that helps with reporting. So if you are, you know, writing a publication, you want to show exactly how you conducted your analysis. So this makes it a lot easier to do that. And it also allows you to really understand underlying workflow.
Okay, so that is kind of a wrap on the single cell stuff. The last thing that I wanted to talk about is a common thing that we do as biologists, which is, we want to look at someone else's paper and understand it, dissect it and reproduce the analysis. So, that can sometimes be difficult because of the size of the data that you are working with. So let's say, someone that has hosted a bunch of fast queue files on SRA, how are you going to get access to their data, pull it to a place that you can work with, and reproduce their analysis. So a specific tool that we have on g.nome to allow you to do that is called SRA import. With SRA import you will essentially import an SRA file which contains the accession numbers to that data on SRA. So if we view this file, you can see, I just have a small data set here with 2 different accession numbers. Then we can go into our SRA import tool and attach that file into the required inputs and it will create this FASTQ output. We just run the tool, go to our runs tab, and look at the outputs. So first, or before I show the individual files, I just want to highlight, this is 43 GB of data. This is 355 GB of data. You may not even have enough like storage on your computer to download that kind of data. So this is why it's really important as bioinformaticians to have appropriate data management tools like this. So we can go ahead and see all the individual files that we just pulled from SRA.
Okay? So that is a wrap on the g.nome demo and talking about single cell data. At this point I'd really like to open the floor to any questions that I can answer.
Hannah Schuyler:
Thank you so much, Nora. It looks like there's a few questions that have been entered into the chat box and again feel free to type in if there's more that come up. But the first one is which methods are used for batch effect correction.
Nora Kearns:
Yeah. So I think I mentioned briefly, we use the SRA algorithm for batch effect correction. But within SRA, there's multiple different algorithms that you can use to perform the batch effect correction. So one of those is CCA, which I believe is canonical clustering analysis and then RPCA which is reciprocal PCA or reciprocal principal component analysis to be more specific. So CCA is great if you have conserved cell types across your samples and you expect, but you expect there to be significant shifts and expression based on experimental conditions, or you're taking them from different donors. And then RPCA is better if you may expect to see differences in cell types across your samples. And it's also great if you have really large data sets because it's less computationally intensive.
Hannah Schuyler:
Great. There's another question, on what types of customization can you do in the single cell workflow?
Nora Kearns:
Yeah. So I showed in the canvas workflow builder the different kind of tools that are running, but essentially anything that you can do in Seurat to customize your, in this Seurat workflow, to customize your analysis you can do in g.nome. So you can adjust filtering parameters. You can adjust the normalization method. You can adjust whether or not you want to regress out technical covariates like UMI accounts. All that is at your disposal, and then we also have the ability to import custom code. And so if there is another tool that you want on g.nome, we can bring that in for you.
Hannah Schuyler:
Great. And I think that's the follow up to that first question around batch correction, asking if there's harmony? And then which version of Seurat are we utilizing?
Nora Kearns:
We use Seurat version 4.3 in a couple of months we'll be uplifting to Seurat V5, which was recently released and we don't currently have harmony available, but as a part of the uplift to Seurat V5, harmony will be available, and that is through Seurat's tool, integrate layers, which also accelerates batch correction. So that's nice.
Hannah Schuyler:
Great. Okay, another question just came in. How can I rerun my single cell analysis with different parameters?
Nora Kearns:
So if you have launched a run, and you decided that you actually want to go back and rerun your analysis but adjust a few things. You can go into your runs tab and clone a run, and then just adjust the parameter. And so let's say you're halfway through the analysis. Or you've already run the analysis. But you decide, you know what I want to go back. And I want to change the batch effect correction algorithm that I used. I want to change it from RPCA to CCA. So you can clone your run and then just adjust that specific parameter and run again, and it will only run from that point on. So that's time saving, which is really nice because sometimes, if you're using, you know, tens of samples, you really do not want to rerun that entire analysis from the beginning.
Hannah Schuyler:
Yep. okay. A few more. If I don't know what annotation to use is there one you would recommend I start with?
Nora Kearns:
Yeah, I think a good kind of default is to use the human primary cell Atlas, because that contains really broad cell types. The only trade off there is that it doesn't have like fine resolution. So, for example, if you're using you know, like the Monaco data set that I showed, that could show, is it a non-switched memory B cell or a switch memory B cell. With a human primary cell Atlas you're not going to get that level of resolution. But you could see, okay, this is a lung cell, or this is a muscle cell, that kind of resolution.
Hannah Schuyler:
Great. And then one last question, is there reason why I wouldn't run batch correction and can I do this with g.nome?
Nora Kearns:
Absolutely. So you do not have to run batch effect correction on g.nome. I would say don't. Actually, there's a lot of cases where batch effect correction doesn't occur like, let's say you did run technical replicates, but they're prepped by the same person on the same day. You probably don't need to do that, and you are expecting to kind of see the same exact thing across samples. So let's say I had 1,000 PBMC's that I prepped at 1055 and 11 am. Like, there's probably not going to be significant technical difference there. And then another one is, if you have, like, drastically different things. So you took one sample from the mouse prefrontal cortex and one sample from. I'm not a brain expert, some different part of the brain. You wouldn't expect to see any overlap in those cells, so you'd want to process those samples differently and not try to integrate the data there.
Hannah Schuyler:
Okay, and then another question. What tools are available for downstream analysis?
Nora Kearns:
That's a good question. So for things like tertiary analysis, we do output all of our files. So we produce intermediate files for every step in the workflow. So you can really download the file at any point that you want. And then, obviously, the output file, the process file at the end. So you can take that file, it's an R data object and you could put it into something like cell by gene for further expression. But particularly if you have this, R data object, you can definitely import it into R and perform further analysis there if you want.
Hannah Schuyler:
Perfect. Thank you, Nora. And then I saw a question come in about the recording, and when it will be sent, we will send this out after today's webinar. So you should get access to this shortly. And if there are not any other questions I want to say thank you to Nora, and then have one last screen that I can share just for everyone that is still on the call, we do offer a trial. So, if you're interested in testing the software for single cell or public data or RNA-seq. That's something we'd be happy to set up with you. You can either scan the QR code or sign up with the link. And essentially, we give you access to g.nome, and we would work with you, kind of understand your research goals and make sure that we can help from a data analysist standpoint. So, thank you everyone so much for joining, very much so appreciate it. And we will be following up with the recording and reach out with any questions in the meantime. Have a great rest of your day.
Moderator
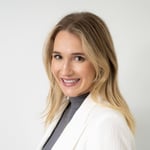
Director of Business Development
Almaden Genomics
Hannah Schuyler graduated from The University of Texas and spent the beginning of her career focused on using digital analytics to inform a marketing strategy for pharmaceutical, biotech, and medical device clients. She has spent the last few years working with clients to help them identify data analysis solutions for NGS and genomic data.
Speaker
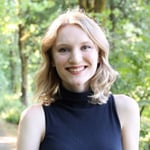
Bioinformatics Engineer
Almaden Genomics
Nora Kearns is a bioinformatician with a specific interest in engineering methods, both biological and computational, which accelerate the research and discovery process. She began her career as a bioinformatician in the cell therapy industry, building pipelines to process data from high-throughput design assays in immune cells. Since joining Almaden she has focused on developing automated workflows for analysis of Single Cell RNAseq data on g.nome. Nora completed her master's in Bioinformatics and Genomics at the University of Oregon where she worked in a molecular engineering lab focused on developing high-throughput assays for protein characterization.