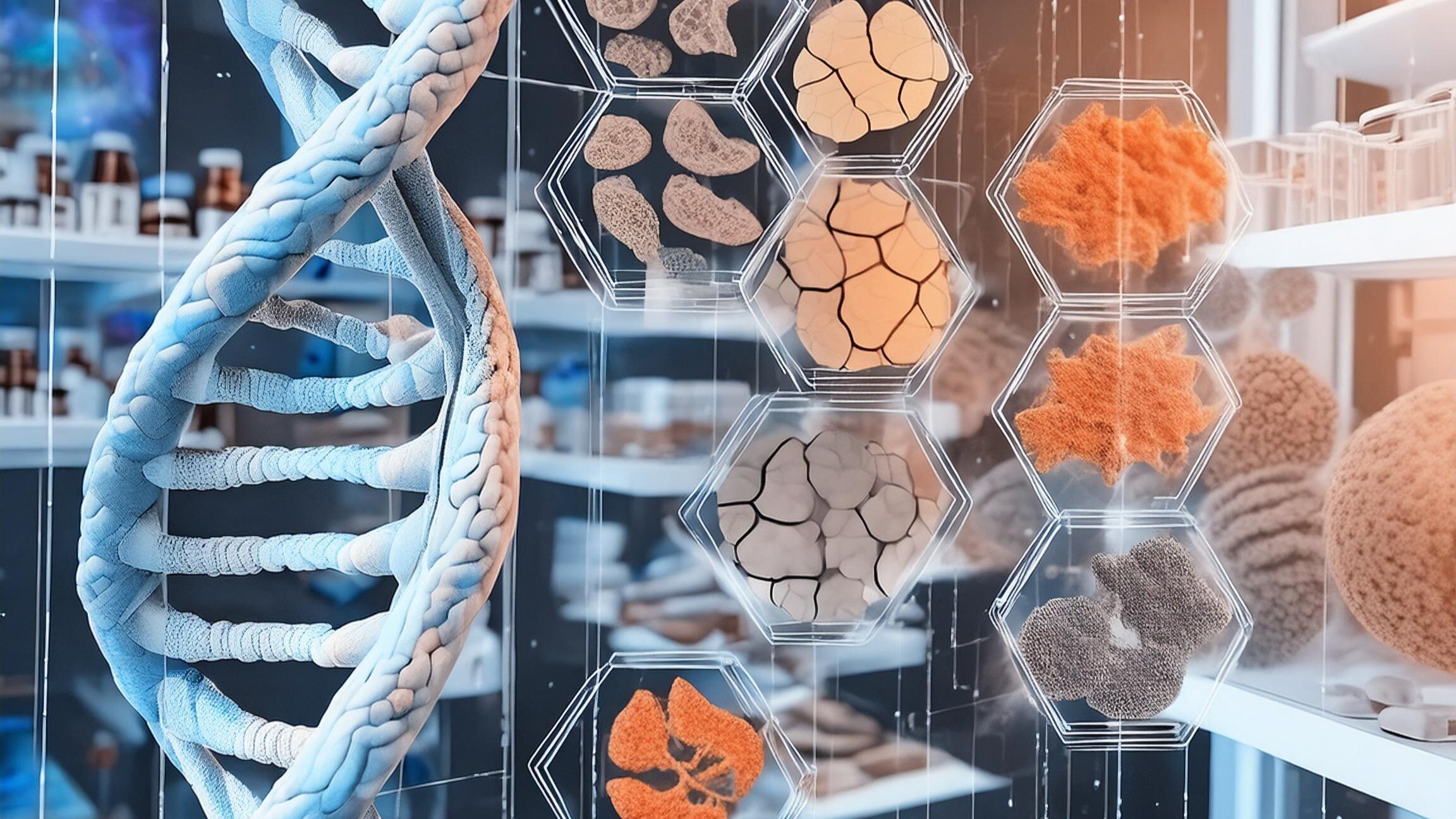
Colorectal Cancer Genomics and Differential Expression Analysis
Colorectal cancer (CRC) is a major cause of cancer mortality, influenced by genetic, lifestyle, and environmental factors. Genetic mutations and hereditary syndromes are significant contributors to CRC, with around 35% of cases attributed to inherited susceptibility. Studies, including those from The Cancer Genome Atlas (TCGA), have highlighted the genetic diversity of CRC, identifying over 250 risk associations and categorizing CRC into four consensus molecular subtypes (CMS), which aids in understanding the disease's heterogeneity and in developing personalized treatments.
Bulk RNAseq and differential expression analysis are pivotal in CRC research, offering insights into the molecular mechanisms of cancer progression. By comparing gene expression in tumor tissues to adjacent normal tissues, researchers have identified genes that are significantly altered in CRC. This analysis, leveraging data from the Sequence Read Archive (SRA), underscores the potential of transcriptomics to identify biomarkers for early detection, prognosis, and treatment response, driving advances in personalized medicine for CRC management.
Discover more insights in our full CRC whitepaper, including additional methodologies like single-cell transcriptomics and survival analysis:
Objectives
- Simplify Complex Analysis with g.nome®:
- Enable researchers and clinicians to effortlessly conduct comprehensive differential gene expression analysis using g.nome’s intuitive platform. This objective focuses on reducing the technical barrier to performing advanced genomic analysis, allowing users to quickly derive meaningful insights from complex data without extensive bioinformatics expertise.
- Leverage Public Data for Enhanced Understanding of CRC:
- Utilize publicly available transcriptomics datasets from repositories like the Sequence Read Archive (SRA) to deepen the understanding of CRC at the molecular level. By analyzing these rich datasets within g.nome, the goal is to uncover novel insights into CRC genetics and transcriptomics that can drive future research and therapeutic approaches.
- Identify Biomarkers for Early Detection and Prognosis:
- Through differential gene expression analysis on g.nome, aim to discover potential biomarkers by comparing gene expression levels in CRC tissues to those in normal tissues. These biomarkers can help in early detection of CRC and serve as prognostic indicators to categorize patients by disease risk and progression.
Workflow
- Data Acquisition:
- Utilize g.nome to fetch four RNAseq datasets from the Sequence Read Archive (SRA), focusing on colorectal tumor tissues and adjacent normal tissues.
- Quality Control and Alignment:
- Perform initial quality assessment with FastQC.
- Pre-process read files with fastp, focusing on quality filtering and adapter trimming.
- Align reads against the human genome using the STAR algorithm, and store alignments in BAM format.
- Differential Expression Analysis:
- Use the featureCounts algorithm to quantify RNA transcripts.
- Employ DESeq2 to compare gene expression levels across samples, identifying differentially expressed genes.
- Results Interpretation:
- Focus on key metrics like log fold change and p-value to assess the significance and magnitude of gene expression changes.
- Highlight the top differentially expressed genes, such as KRT23 and ETV4, which have documented roles in CRC progression.
SRA accession number |
# of colorectal tumor tissues |
# of adjacent normal tissues |
Study name |
Reference |
SRP219837 |
5 |
5 |
Epigenomics landscape of colorectal cancer |
[12] |
SRP301216 |
5 |
6 |
Identification of genes associated with the onset of colorectal cancer by transcriptomic analyses of the adenoma-carcinoma sequence |
[13] |
SRP344867 |
5 |
4 |
RNA Sequencing in Adenoma-Cancer Transition |
[14] |
SRP245232 |
3 |
3 |
RNA-seq of CRC tissues |
[15] |
Table 1. RNAseq datasets used in this study |
g.nome streamlines access to SRA datasets through an easy-to-use workflow illustrated in Figure 1. Users simply input sequencing run IDs from one or more projects, and g.nome leverages the NCBI SRA toolkit to efficiently download and extract FASTQ files, which include both read sequences and their quality metrics. The platform allows customization of download parameters, such as using the fasterq-dump tool with the "split=3" option. This setting organizes paired reads into two files and unpaired reads into a third, ensuring data is neatly arranged for subsequent analysis steps.
Figure 1. Fetching RNAseq datasets from the SRA database according to a list of accession numbers. |
Following this, the workflow for quality control and alignment, as shown in Figure 2, was initiated. It began with evaluating the data's initial quality using the FastQC software. FastQC offers comprehensive insights into several fundamental quality metrics, such as per-base sequence quality, GC and N content, sequence length distribution, and identification of over-represented sequences (refer to Figure 3). Configurable options in FastQC allow for further customization, enabling users to input lists of known contaminants and adapters, enhancing the precision of the quality control process.
Figure 2. g.nome workflow for quality control and alignment of reads. |
Figure 3. Read quality assessment by FASTQC. The graph shows per base sequence quality for forward reads corresponding to the sequencing run SRR16832117 from the study of Orouji et al. [12]. |
Read files underwent pre-processing with fastp, which indicated that for the sequence run SRR16832117 (referenced in Figure 3), about 95% and 90% of bases had quality values exceeding 30 and 20, respectively. Fastp detailed the count of reads meeting quality standards and those discarded due to low quality, unresolved bases, or inadequate length, alongside reads and bases trimmed for adapters.
Subsequently, both filtered direct and reverse reads were mapped to the human genome's reference sequence using the STAR algorithm, resulting in alignments stored in BAM format. For instance, from the sequencing run SRR16832117, 39 million reads, with an average length of 294 bases, were processed. About 92% of these reads were uniquely aligned to the genome, 4.6% aligned to multiple locations, and the rest were unaligned for various reasons. g.nome further employed Picard tools for mate-pair verification and duplicate marking, deriving from the same DNA fragment.
These curated alignments, stored in BAM files, fed into the primary differential expression analysis workflow (illustrated in Figure 3), employing the preprocessing and alignment steps as foundational workflows. This showcases g.nome's capability to integrate simpler workflows into more elaborate analyses hierarchically, allowing for workflow reuse across different studies. The featureCounts algorithm quantified RNA transcript abundance by aligning reads to the genome, with the generated gene count matrix analyzed by DESeq2 to identify differentially expressed genes across varied conditions or groups.
Central to evaluating and understanding gene expression differences are two metrics: log fold change, which reveals the extent and direction of gene expression shifts, and p-value, assessing the statistical relevance of these changes. The analysis spotlighted the top 10 genes with significant differential expression, detailed in Table 2 and depicted in Figure 4, many of which are recognized for their roles in colorectal cancer. For instance, research by Zhang et al. highlighted how Keratin type I (KRT23)'s overexpression accelerates CRC cell proliferation and migration, suggesting its potential as a therapeutic target due to the pronounced impact of its suppression on tumor growth. This finding is supported by Hosseni and Nemati's identification of several keratin family genes as notably overexpressed in CRC contexts.
Figure 4. The main workflow for differential expression analysis. |
Figure 5. Volcano plot visualizing the genes differentially expressed between CRC samples and normal adjacent tissues. The top ten differentially expressed genes listed in Table 2 are highlighted by larger circles. |
Gene name |
Log2 fold change |
Adjusted p-value |
Protein name |
Known roles in colorectal cancer |
Selected references |
KRT23 |
6.7 |
6.9e-23 |
Keratin, type I |
Promotes colorectal cancer growth by activating the expression of human telomerase reverse transcriptase, which has been associated with the proliferation and migration of CRC cells |
[20] |
ETV4 |
4.0 |
1.7e-22 |
ETS translocation variant 4 |
Plays a crucial role in the progression of colorectal cancer by enhancing cell proliferation, invasion, and metastasis, as well as influencing the tumor microenvironment |
[21, 22] |
CPNE7 |
4.6 |
8.0e-22 |
Copine-7 |
Unclear, suggested to be a prognostic factor and therapeutic target in CRC |
[23] |
GRIN2D |
4.1 |
6.4e-21 |
Glutamate receptor ionotropic |
Angiogenic tumor endothelial marker specific to colorectal cancer vessels. Its expression correlates with improved survival in CRC patients. |
[24] |
BEST4 |
6.1 |
3.1e-20 |
Bestrophin-4 |
Suppresses epithelial-to-mesenchymal transition in colorectal cancer cells. |
{Wang, 2023 #37} |
STRA6 |
4.5 |
6.6e-19 |
Receptor for retinol uptake |
Plays a key role in colon cancer stem cell maintenance and contributes to high-fat diet-induced colon carcinogenesis |
[25] |
CDH3 |
5.8 |
2.0e-18 |
Cadherin-3 |
Elevated expression in CRC, demethylation linked to advanced CRC |
[26, 27] |
KLK6 |
9.5 |
1.9e-17 |
Kallikrein-6 |
Prognostic biomarker due to dramatic upregulation in CRC, key role in colon cancer cell migration and invasiveness |
[28, 29] |
LARGE2 |
3.7 |
3.1e-17 |
Xylosyl- and glucuronyltransferase |
Increased levels in CRC compared to benign colonic epithelial cells. Involved in CRC cell migration and adhesion |
{Dietinger, 2020 #36} |
MMP7 |
7.5 |
1.2e-16 |
Matrilysin |
Required for CRC tumor formation, affects drug resistance |
[30, 31] |
Table 2. Top 10 genes differentially expressed between tumor and normal tissues. |
Interested in more? Discover additional insights, including single-cell and survival analysis, by downloading the full document.