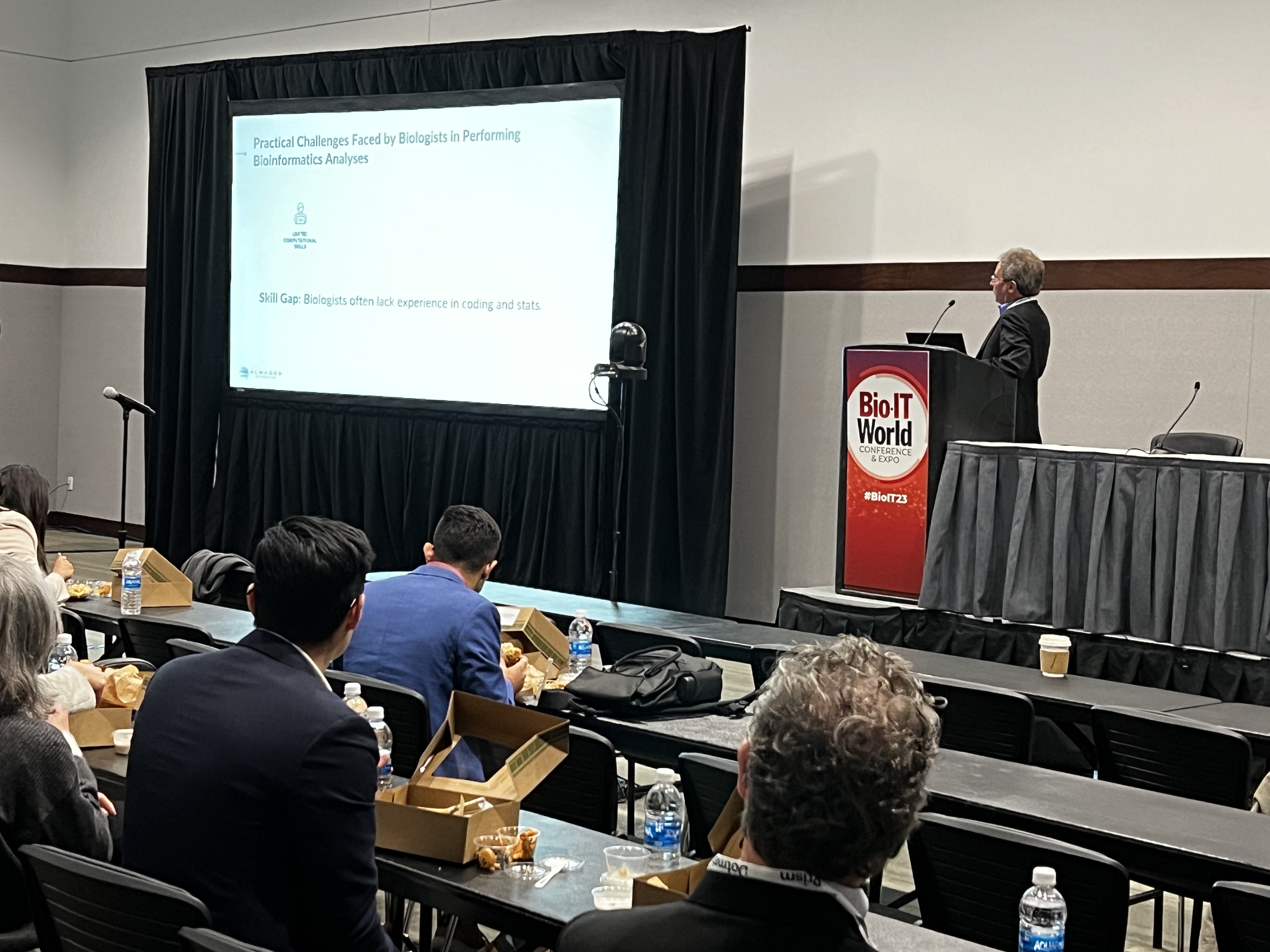
VIDEO: Investigating Nuclear AGO2 in Colon Cancer
We explore Argonaute proteins' binding in human nuclear RNA and its effects on alternative splicing. Our study reveals a novel RNAi mechanism controlling gene expression through splicing, highlighting miRNAs' importance in physiology and disease. Leveraging a state-of-the-art bioinformatics platform, we show how democratizing bioinformatics with reproducible computation, interpretability, and user-friendliness accelerates discovery.
Speaker
David Corey, PhD
Professor, Pharmacology & Biochemistry
UT Southwestern Medical Center
David Corey is a Professor at UT Southwestern Medical Center. He received his undergraduate training in chemistry at Harvard University and his PhD in chemistry at the University of California at Berkeley. He did postdoctoral work at the University of California at San Francisco before joining UT Southwestern in 1992. Dr. Corey's area of interest is modulation of gene expression by synthetic nucleic acids.