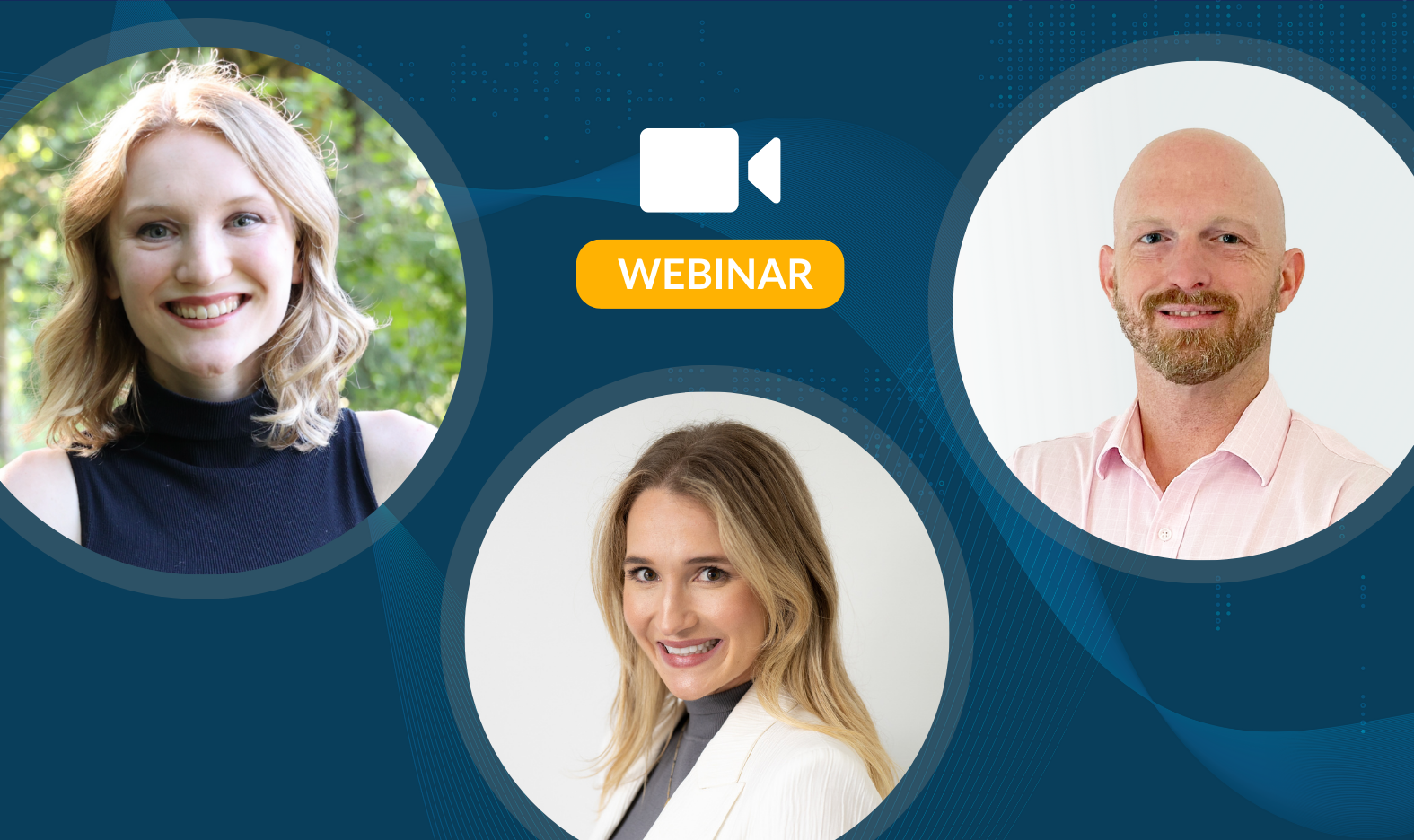
Single-Cell Mastery: Drug Discovery Data Analysis with g.nome®
In the dynamic landscape of drug discovery and therapeutic development, single-cell analysis has emerged as a game-changer, offering unprecedented insights into cellular heterogeneity and paving the way for breakthroughs in precision medicine.
In this fireside chat, we embark on a transformative journey into the world of single-cell discovery and learn how g.nome® can simplify the complexities of single-cell data analysis, empowering you to unlock the full potential of this revolutionary approach. Whether you're considering integrating single-cell techniques or optimizing your current workflows, this fireside chat will equip you with the tools and knowledge to drive innovation and success in your life science research.
Key webinar highlights:- The Significance of Single-Cell Analysis: Explore why single-cell techniques are pivotal in drug discovery, enabling the identification of rare cell populations and the discovery of actionable biomarkers.
- Challenges in Single-Cell Data Analysis: Gain insights into the complexities of single-cell data, including quality control, data integration, and the extraction of biologically meaningful information.
- Cloud Solutions for Single-Cell Data Analysis: Discover how to simplify the single-cell data analysis process.
Webinar Transcript
Hannah Schuyler:
Well, thank you. Everyone for joining our discussion today on single cell. We're super excited to have this discussion with you guys today.
So today's conversation, of course, will be focusing on single cell as it relates to everything in the landscape. My name is Hannah Schuyler. I'm a director of business development. Here at Almaden Genomics we also have our senior director of product management, Kit Furman joining us as well as Nora Kearns. Our in-house single cell expert environment so super excited to have our panelists today for the discussion.
Just a few quick things before we get started, this fireside chat is being recorded. And we will send it out to all attendees and registrants after the session wraps today, and then please enter any questions that you have for myself or Nora or Kit in the chat box. And we will address those at the end during a live QA. Session. So please feel free to enter them in, and we will discuss those at the end. During our live QA. Session.
So what will we be discussing today we will be talking through everything as it relates to a single cell. So what's new and exciting in terms of why single cell is important and what that means for researchers.
We will also talk through some of the challenges that researchers face when analyzing single cell data. I know, of course, analyzing large data sets is sometimes complex for researchers. So we'll be talking through some of those complexities. And then we'll also be talking through some of the advancements. So of course, single cell is important. But then researchers wanted to get one step further and doing multi omics or spatial analysis will be touching on that as well.
And then, lastly, we will have, some few minutes at the end for live. QA. So definitely want this to be interactive. If you have questions, please feel free to put them in the chat box, and we will address this at the end.
So just briefly, before we get into the discussion. I wanted to give you guys an overview of what Almaden Genomics is and who we are.
We are a software company that spun out of IBM Research Center in Almaden, California. Hence the name Almaden Genomics so the software was originally built as an internal need for pet and food safety, and the team quickly realized that there was a need and value for building pipelines and workflows for customers that may not know code and so that's how genome was born.
Over the last year we've been working with a variety of different biotech, pharmaceutical and academic clients to help researchers process and analyze their data.
And what are we trying to do here at all? But in genomics I think one of the biggest things that we are focused on doing is really bridging the gap between both users being the biologist and the data scientist and the bioinformatician. I'm sure many of, you know that a biologist goal typically is to analyze their data and experiments. They have either in house sequencing data or publicly available data. And they're really focused on interpreting that data, making visualizations and making sense of the data.
So being able to do that without knowing code or how to build pipelines is super important for them, and they often are relying on bioinformaticians to do the analysis for them. And this can be a very time consuming process. And there's not always a common language between the biologists and the virus and the data scientists. And then, on the other side of that coin is the and the data scientists. Right? They are really focused on building pipelines, workflows, leveraging open source tools. And their biggest thing is, you know, helping the biologists to analyze their data.
With that, we are really focused on trying to bridge the gap between both the biologists and the data scientists and bioinformatician.
So on g.nome, we have a variety of different pre-built workflows that a user can access and so today's discussion, of course, is focused on single cell. And there's many ways that you can actually process single cell data on the software, one of that being publicly available data through our Sra integration. So you have the ability to pull in public data process that through our SRA tool from start to finish.
You can also process in-house sequencing data that you're working on. So whether that's pulling data from a local file. Excuse me, a local server or an bucket. So you can process large amounts of data in that way as well.
The third component of that is some of the data partners that we're working with. So we're working with a variety of data partners that provide harmonized and curated data sets that can be supplemental to the data that you guys are working with. So all of that can be processed through our single cell workflows.
And then the goal is that you can actually utilize one of our pre-built workflow. So we're using Seurat and star solo for our workflows so you can start with that. Upload your FASTQ file. Bring in your metadata file and go start to finish with those visualizations, or you can make modifications to the workflow as well. So there is that custom aspect of it. Where you can do batch correction, you can adjust normalization filter itself. We really want to provide that custom layer to our researchers as they're looking to analyze their single cell data.
And then you can also create custom visualizations as well. So we do have Jupiter notebooks integrated into the platform. So this is again allowing you to create those custom visualizations. And then the last component of that, of course, is creating and sharing reports. So we do have interactive plots and reports that can be shared with your colleagues as they are looking to visualize and analyze the single cell data. So that's kind of a quick overview of online genomics and our software genome.
And so I would like to kick off this fireside chat and discussion with a poll for the audience. So you should see it pop up in just a second. So if you could go ahead and answer this. Are you working with single cell data right now, or planning to in the near future? Yes, I'm working with single sets even now. Not yet. But I'm planning to in the near future. Or no, I'm just curious to learn more. Give it a couple minutes for the responses to show.
Awesome. So it looks like we actually have a mix across the board with people working with single cell data. As well as some that are not yet, and are just curious to learn more, so I think this is a perfect segue to kick off our discussion with Nora and Kit. Nora, I know that you are our in house, single cell export. Do you wanna give us an overview of single cell sequencing? And why this is such a revolutionary technique in the space.
Nora Kearns:
Yeah, of course. So single cell RNA sequencing refers to the process of sequencing the transcribed RNA from individual cells. And this is really useful, because, let's say, you have a sample of lung tissue that you're interested in exploring, and you want to identify the genes that are expressed in that lung tissue.
Well, there's multiple different cell types in lung tissue and then traditional bulk, RNA sequencing, we would only be able to find the average gene expression across the entire population of cells in that lung tissue, but with single cell cells are basically isolated in little individual droplets before we sequence them. So we are able to measure gene expression in each individual cell in the tissue sample. So within the lung you'd be able to see how epithelial cells, immune cells and nerve cells all express varying levels. Even within their own cell types. So how do the different? How does each individual epithelial cell express differently. So the analogy that's frequently used to describe single cell compared to bulk RNA-seq is that of a smoothie versus individual fruit. So when you're doing bulk, RNA-seq. You're basically creating a smoothie, and you might not be able to tell where each kind of flavor is coming from. But when you have the individual pieces of fruit that went into that smoothie? No, okay. So the sweetness came from the strawberry, and the acidity came from the lemon, or something like that, I think, is a good analogy for it.
And single cell is again very useful, because profiling the gene expression, activity in cells is really considered one of the best approaches to understanding cell identity and cell state as well as function, and then response to different stimuli which might be really relevant in terms of drug discovery and disease understanding.
Hannah Schuyler:
Yeah, that's great. Any you did touch on this briefly. But what are researchers really trying to uncover from a scientific standpoint when they're looking at their single cell data.
Nora Kearns:
So I kinda think of this as having 2 sides, the translational side as well as the research and discovery side. So I think translation is what's really most relevant to the to the general population and to medicine and I think single cell has the the ability to really advance personalized medicine. So, for example, in cancer research single cell can reveal the existence of sub populations, of cells within. Perhaps a solid tumor that might have different mutations, or different response to treatment.
And that can help doctors really understand, you know, why individuals respond differently to therapies, and help them develop personalized medicine strategies. And I think a good example of this is we recently read a paper at Almaden on breast cancer research and using single cell to understand breast cancer tumors and traditionally with breast cancer diagnostics. There's only a few, you know, diagnoses of breast cancer, how we classify tumors. But when you use single cell to explore individual tumors from patients, even within the same kind of diagnosis category. So all these tumors are all triple negative breast cancer. We see that there's actually massive diversity between those tumors, and that that can really help us understand why one patient might not be responding as well to a certain type of therapy as another. It helps us move towards better personalized medicine.
And then, I think, from a research and discovery standpoint for people like myself, single cell can help us enhance our understanding of biology overall and create resources that will help the field, accelerate research and discovery. There's one initiative I'll speak to in particular which is the Human Cell Atlas project.
The Human Cell Atlas project is an international collaborative consortium with the objective of basically charting all of the cell types in a healthy human body from childhood to adulthood. So that would just be a fantastic research or resource for the field to have kind of a high resolution catalog of cells for you know a number of reasons. It would facilitate our understanding of disease mechanisms. It would be an educational tool and also as an open source tool, it would really help democratize research. So Labs don't have to spend a lot of money doing more basic experiments just to get kind of an idea of what cells they're looking at. It would just kind of you'd have that resource available. So I think it's really valuable from those 2 perspectives of translational medicine. And then also, in terms of basic and discovery and research.
Hannah Schuyler:
Yeah, I definitely would agree with that. And I'm sure, Kit, a lot of this resonates with what you're hearing from customers. Can you maybe expand a little bit of on what what you're hearing from our customers when they're reaching out about single cell?
Kit Fuhrman:
Yeah, absolutely. You know, people are doing such complex experiments now, whether they're in the pre clinical space and doing, you know, research. Or they're close to the clinic, and it's sitting clinical trials. If there, you know. There's so much analysis that needs to be done. And it's such a critical component of the the scientific workflow. But you know, we're all struggling to find, you know, time, the right collaborators or the the right software tools to analyze this data. And you know, as as Nora stated, you know, single cell has become an important component of all of these different types of studies. And so you know, analyzing the single cell data is a real challenge for the whole community. And that's why you know, people come to g.nome to find solutions to those problems. Right? You know they're trying to right now, splice together. Different solutions from different software packages or open source tools. You know, what? What I hear from customers is they really like the ability to bring in software tools from various places all into one place in the g.nome canvas, and, you know, have them presented as tiles and visual elements that they can then change parameters and draw connections between tools. And really have access to all of that open source. Environment to build and modify workflows. You know. So they have access to Seurat right in the canvas. And they don't have to load up a coding environment. They can do it in a canvas in g.nome, no code environment. And just build those workflows, or actually, you know modify the pre-built workflows that Nora has built for our customers.
Hannah Schuyler:
And I know it's something that I hear quite a bit from our customers as well, right as they just struggle with getting the analysis done. And so it's great to hear that. You know we have solutions in place to help with that. So as a follow up to that Nora are there any breakthroughs within the single cell research that you are most excited about?
Nora Kearns:
Yeah. So as an engineer, I think what's always exciting to me is seeing how technology develops single cell, really only came about in 2009. And since that time we've seen just so many advancements. And so it's always exciting to me to see just the the field accelerating a really fast pace. And I'm always excited to see how people take a technology and then adapt it and get really creative with it. So one that all mentioned in particular that I thought was exciting is the ability for live sequencing. So one of the limitations of single cell is that our we capture it and a static point. So we only see gene expression from 1 point in time, and the you know, performance of the analysis or the processing of those cells kills them. But live sequencing allows us to study cells. You know, in real time, basically by repeated sampling of the cytoplasm. And that opens up, you know, a couple of doors for us. So we can start looking at changes and expression over time which help us understand response to stimuli which is relevant in drug discovery as well as understanding. You know, the trajectory of a cell over the time course of its development.
And then another one that's a bit similar. Is lineage tracing with single cell, which is where a cell is engineered with an inheritable barcode. So all of its progeny inherit that same bar code, and that allows us to, you know, trace the lineage of a cell. And it's relevant to developmental biology. So we can learn how complex organisms develop from individual cells through this bar coding, which I think is very exciting
Hannah Schuyler:
Definitely. Thank you for your perspective on that. I think another huge component of single cell, right is the analysis. And we've both kind of touched on this and that. There's a lot of moving pieces. There's large data sets. There's complexities when it comes to analyzing these types of data. Nora, can you explain, you know, some of the pain points that researchers might face when they're trying to analyze these complex data sets.
Nora Kearns:
Yeah, so I'll just talk about a few things that I feel like I experience all the time when I'm working with single cell. Probably the first most obvious is just the the sheer data size and the complexity of the data. So single cell data sets are typically very large. If you think about it, you're basically taking, you know, thousands to hundreds of thousands of replicates that are all getting sequenced. So that's just a massive data set, and then also, as cost per cell, you know, decreases data. Size is, it is increasing even more and so that makes storage and processing pretty computationally intensive. So you have to have architecture in place for that.
Another big one is standardization. So as single cell has grown more popular and more pervasive across different fields. We've seen more platforms, for creating the data. And then technologies and software for processing the data arise. And I think kind of an interesting little number is that since single cell was developed in 2009, we've seen the number of publications using single cell grow exponentially. So in 2020 there were 2,000 publications which generated single cell data using a number of different technologies, and when the technologies, or when the kits are different and when the software for processing is different, it really can start to complicate how we compare studies and how we integrate data, and it can affect, you know, reproducibility of analysis. So I think standardization is a big problem. And then and we need better kind of architecture for standardization.
And then cell type annotation is a big one that I'm sure a lot of biologists ex experience as a pain point. You know, at the end of a single cell analysis, you, the goal is to cluster your cells and identify what those cells are. So it really doesn't mean anything. If we're, we aren't actually able to identify and annotate what those cells are. And there's a few methods to do this which can involve varying levels of manual and automated annotation. So if manual annotation, it really requires a user to know the marker genes that are associated with their cell types and automated annotation can take a couple of different forms, but regardless of which form it takes, it does require some sort of reference or training data set, meaning that there has to be previously established data sets of cells and their associated annotations by which you can compare your own unannotated data. And as you can imagine this would be very difficult. If you don't know what you're looking for in your experiment, or if you are trying to identify novel cell types for which there's no good reference. And I think this kind of echoes our need for again, for a comprehensive cell Atlas and for strong data sharing and standardization practices. So that people just have access to a broader database of you know, re, or yeah, broader resources by which to do their analysis.
Hannah Schuyler:
Yeah, and I think there's definitely a lot to unpack here. You mentioned things around data and complexity as well as trying to annotate some of these cell types. Okay, Kit, it would be great to get your perspective on this. And how do you know g.nome might be able to help address some of those things that Nora talked to.
Kit Fuhrman:
Yeah, absolutely. And I think you know, as as a scientist, you know, the first thing that you do after you run these, one of these big NGS experiments is, you're like, what am I gonna do with all of this data? And it's this whole new problem that you have to solve. You know. Fortunately, and even with the basic IT infrastructure, it's still difficult to figure out what where to put it and how to analyze it. Fortunately, g.nome, you know, offers a single place to store, process, and analyze. You know, all different types of Ngs data. And so you know, you were able to kind of easily move the data to there and process it.
In addition, with all the publications, as Nora said, there's so many different tools and standards that have been developed over the last 10-15 years for genomic data. It's it's hard to know which ones to run, and you know, ha! Converting between different standards and file types can be very daunting. And it's nice to have a single place like genome where users can do all of that in in a single environment. And so we're really reducing the complexity of single cell analysis. But providing a lot of these tools in the environment and these pre-built workflows with popular tools, such as Seurat, so that you can, you know, cluster and annotate your cell populations with, you know, data from other places. And so it makes it a lot easier to move to the point of interpretation. So you're just getting to the point where you're, you know, modifying workflows or swapping in different tools. And you're able to quickly go and make those comparisons and analyses. You know, for your experiments and actually make comparisons like control versus treatment groups or Time series, and kind of gain that understanding from your data as quickly as possible.
Hannah Schuyler:
Nora, from your standpoint, is there a feature in genome that you are most excited about?
Nora Kearns:
Yeah. So I think what's always exciting for me is when I and we as a team, are able to take something that I previously experienced as a real pain point in doing analysis. You know, off of platforms off of g.nome. And then we bring it onto g.nome. And we're able to resolve that issue for the user. So one example of this is processing really large data sets. I recently analyzed a data set 26 samples 130,000 cells and just wasn't able to do it locally, you know, as your as your compute resources become more limited, it gets really challenging to integrate, you know, 26 different samples. And so I was excited about how we handle that on g.nome. Just to make you know, we don't want the user to worry about compute resources, allocation and things like that. It's just I want to be able to run this big data set without it, you know, failing when it when it hits the start point. So I'm really excited about that and how we've built in parallelization as well. So if you're processing lots of data it should take the same time. It's just processing one sample, because all of these steps are parallelized. So I think that's one that I'm really excited about and then, as someone who loves data visualization, I'm really excited about the reporting that we've brought in. So it's so important to be able to create figures that are easily digestible and understandable, and to be able to share your data with co-workers or your manager. And so I'm really glad that we brought that capability onto g.nome as well, that every time you do an analysis run, you get information about the QC. And the clustering and feature visualization and things like that that our users are really interested in just all in one concentrated place.
And I know I'm really excited about what we've done with allowing researchers to actually customize the workflows. That was something that I would hear a lot from our customers is that either it's too simplified right, and that there's no ability to actually modify the workflow, or it's too complex, and that you have to know command, line and code. And so something on g.nome of course, right is that you can do things like batch correction or normalization or filter out.
Hannah Schuyler:
Some of those cells which is, of course, super super important to the researcher. So I'm very excited about just how the workflow has been built itself. So thank you both for your perspective on that and we talked a lot about some of the challenges. I think one of the other things that we hear a lot in the space is artificial intelligence. And how this is revolutionizing drug discovery. It's a word that's thrown around, thrown around quite a bit. So I'd love to get your thoughts, Nora, on the role of machine learning, and AI, as it relates to addressing some of these challenges around single cell, maybe, as it relates to clustering or automated cell type identification.
Nora Kearns:
Yeah, so single cell is really the perfect application for machine learning. In my opinion, because we start with a very large, high, dimensional data set, and the goal is to detect very subtle patterns and differences.
Ml is already critical to single cell analysis, whether we recognize it as that or not. But we use unsupervised Ml, algorithms like Tc and UMAP to cluster cells based on gene expression patterns already. So what I'm really excited about is how I think we're going to see foundational models start advancing single cell analysis. And when I talk about a foundational model. What I'm referring to is a model that is trained on a broad base of data, and it's capable of diverse tasks. So they can serve. These models can serve as a foundation upon which to build more specialized models. An example of this is Sc. GPT which came out of the Bo Wang Lab at the University of Toronto, and their foundational model is valuable because it can be tweaked to fit a variety of downstream tasks which are really pain points and single cell analysis. So it can be tweaked for cell type annotation batch integration as well as multi omic integration and their most updated model was trained on 33 million cells from various tissue types. And when it was tested on a cell type, annotation task was about 85% accurate compared to manual annotation, which I think is already pretty good. But as the field advances and we get more and more and more public data out there, our training data set basically grows. And so we can see that the accuracy, and capability of these models is only going to increase so I think that's something that's going to be really exciting to watch how that affects the field over the next, you know, 5 to 10 years.
Hannah Schuyler:
Yeah. And Kit, I'm sure that this is something that your team has thought about it would, what what is your take on this. What do you think is the greatest potential for AI and Ml, yeah, absolutely. I think. You know, our ability to generate data has far outstripped our ability to analyze and interpret the data we, you know, especially as sequencing becomes less and less expensive. We're just doing more and more genomic experiments. And so
Kit Fuhrman:
The real exciting thing about these foundational models is that we could teach them the basic, the language of nature. I mean, you use them to pull out insights that it would otherwise take us years to figure out. You know, and and single cell GPT is a really good example of this. You know it takes the laborious task of, you know, drawing gates around cells and make it easy. And this is, you know, one of the reasons why I think g.nome is so powerful, and it that it it lets our users take advantage of these cutting edge tools right? And they can bring them into the platform, and they can either, you know, build a whole new workflow or they can tack them onto a workflow that they already have or replacing existing tool. And then all that flexibility as well as the power of these brand new AI, ml, tools. And I know I hear it quite a bit when I'm talking to customers, they're always asking about AI and Ml, and how it relates to our software.
Hannah Schuyler:
So I think for kind of the remaining time of this discussion, I'd like to focus on what's next, and where this where we're heading in this space. So, Nora, how do you think single cell is gonna expand? And what needs to be done moving forward to make sure that we're meeting the needs of our customers.
Nora Kearns:
So I think the most obvious is probably just higher throughput and scalability. So it's cost decreases. We're going to see people doing larger and larger experiments with more cells. And so technologies both, you know, biological and computational need to be even more high throughput to handle these data sets. And that's going to require automation, scalable data storage and scalable processing methods.
And then also, multi-omic integration is a big one. Right now. So we're moving towards integrating multiple types of data. Such genomics, transcriptomics, proteomics, all at the individual cell level. And the complexity of that is a major obstacle. It's going to require development of new technologies and pipelines that can capture and analyze multiple data types simultaneously. And I think, also integrating that volume of data is going to require significant computational resources.
And I think that also kind of leads to my next thought is just on standardization and reproducibility. So as it's becoming more widespread, what I would really like to see is, you know, more better standardization to enable data sharing and reproducibility. Because there have been so many times where I take a public data set. And I'm trying to go to their methods and figure out what they did. And it's always like next to impossible cause. There's just not quite enough information there. So I think that's one of the things that I really value about g.nome, not just as an engineer, but as a user of it all the time, is that I can go back and see every run. What are the exact parameters that I use? And I think tools like that are going to help the field move towards better data sharing and reproducibility.
I think, the last one that's becoming bigger, is spatial transcriptomics. And just as we saw single cell start to become more standard and more and more people using it across different disciplines. I think we'll see the same for spatial transcriptomics. Because it allows you to capture not just the gene expression data of individual cells, but to see where those individual cells are located in space within your tissue, your sample so that you can start to explore questions about the micro environment that that cell's in and how it's communicating with cells around it which will be really exciting to see. You know, the discoveries that are that are made from that.
Hannah Schuyler:
Yeah. And I would agree definitely with all of those points that you touched on from a customer standpoint. That's what I hear all the time. Especially around multi omics. Researchers are wanting to process not only their single cell data. But they want to start to combine it with their ATAC-seq data and look at their gene expression data with other types of data, public data, right? And so I think that that's gonna be a really important component of the analysis, and then, of course, being able to reproduce that right? That's what researchers always wanna do is do that moving forward. And then, lastly, you touched on spatial, which I know I'm super excited about. I know Kit is excited about as well. It's kind of the newest thing on the horizon in terms of adding content to the single cell data that you're analyzing. So Kit would love to get kind of your perspective on this. What does that mean for for us and for our users on g.nome?
Kit Fuhrman:
Yeah, absolutely. So, you know, single spatial allergy is such an important addition to single cell genomics. And you know, instead of just, you know, inferring cell interaction networks through gene expression patterns. You can actually look at those cellular neighborhoods and kind of piece things together about. You know how one group of cells at a certain location are affecting another.
I was at SITC last weekend, and there was some great talks about how the environment around. A tumor acts as a physical barrier, preventing the immune system from destroying the tumor. And you know, we can only start targeting those cells and changing that environment if we know where they're located and how they're interacting together. And it has so much promise for medicine and research. But notably spatial biology is a magnitude greater in data, storage and processing needs. Because it's just so much more information, spatial data. And so you know, that's where cloud computing is so important. You know, it's already important for single cell, and becomes even more important when you layer on spatial data and then try to integrate all of those different data types together. And so you know the great thing about g.nome. We're providing all of that cloud infrastructure to our users to process these large data sites sets so that scientists can really just focus on the data analysis. And not since time managing the cloud accounts or computing cluster. And they could just focus on trying to gain as much insight from their data as possible.
Hannah Schuyler:
Yeah. And I think as a follow up to that I know at Almaden Genomics, we're really focused on becoming that end solution for our customers. Right? Like, right now, we can help with processing the data, whether that's in house data or publicly available data. We're also working, of course with the partners on the upfront portion of the analysis. So those curated data sets that can be brought into supplement as well as the downstream. So that's the predictive modeling, using analytics and really trying to be that end to end solution. Can you talk a little bit more about the future of g.nome and Almaden Genomics. And what this is really gonna mean for our customers.
Kit Fuhrman:
Yeah, absolutely. You know, we have unique solutions today that allow data, scientists, benchtop scientists and scientists of all types to collaborate and analyze large data sets. Today they have our pre-built workflows. That are available to our users where they can come in and you know analyze their data on very reliable and robust informatics, pipelines. And we're also building up brand new functionality. As Nora mentioned, we have a lot of figures and graphs and tables that you get as part of your analysis.
But we're also building workflow reports right where you get all your data in one report. And it generates those useful figures. So you have a detailed layout of all the critical results for a single experiment. I'm really excited to get that in in customers hands.
Finally, we're always working very hard to bring in new open source tools into the environment especially in the realm of AI and Ml and integrate these new data types, such as protein and epigenetics and spatial into genome workflows. So I think the future looks really great for the users on who are analyzing data on the g.nome platform.
Hannah Schuyler:
Awesome. So thank you both for your perspective on you know everything as it relates to single cell it looks like we do have some questions in the chat box, and for those that are still hanging on. If you have questions, feel free to type them in the chat box. But the first one we have is, do you have workflows in place to process publicly available single cell data?
Nora Kearns:
Yeah. So we're right now bringing in a workflow for integration of data from SRA. So that's raw, FASTQ sequencing data. And so that will allow users to take the raw, FASTQ data, pull it in just with the click of a button on genome and then run single cell analysis from there and then we're also working on the same for Gfetch. So GEO is where people typically host, you know, count matrix data. So they've already aligned their data. And they've gotten to the point of count matrix and so we're going to build the same pipeline for GEO. So it's currently available as SRA and then what will be available as fetching data from GEO, because often you just want to start from that point, and or you potentially don't have access to the raw, FASTQs. We want that to be accessible as well.
Hannah Schuyler:
Awesome. And then another follow up question is what visualizations are users able to create on g.nome?
Nora Kearns:
Starting with QC, we show users the distribution of various quality metrics, such as the number of unique genes, as well as number of UMIs in their counts data, and then also the distribution of mitochondrial DNA. We also show them scatter plots of various combinations of those same QC metrics, which can help them identify patterns in the data. And then we also show them the top variably expressed genes within each sample. And then, in terms of clustering, we show them either a UMAP or t-SNE visualization, whichever has been chosen. And then we also show them the top expressed genes per cluster and then we show them the feature visualization, so they can go in and examine a specific gene which is very useful for cell type annotation.
Hannah Schuyler:
Awesome. Thank you. And it looks like we have one last question, how important is data quality and accuracy and single cell analysis, and what steps can be taken to ensure the reliability of the single cell data.
Nora Kearns:
Yeah. So QC is absolutely essential. I think, you know, speaking from experience of trying to reproduce analysis from public data. If you don't know the filtering parameters that you use. You are not gonna get lucky with your analysis. Probably not gonna happen upon that. If there's you know, hundreds of different options that they could have used. So it's really essential to know what QC parameters were used to filter the data. And I think that will just completely change the results of your clustering, and I think steps that need to be taken to ensure the reliability of that kind of what I mentioned before is that you need really good logging of everything that you're running, and as a buyer and partition, if you're doing all of that work yourself off platform. You really need to be accountable and keeping good track of what you're doing. But if, you know, when I run things on platform on g.nome. That is all handled for me. So it's really easy for me to just go to my runs and see exactly what ran, what parameters I use to get kind of the outcome that I desired, because we know that really one of the needs in single cell analysis is for iteration. So you might have to. You might spend 80% of your time iterating and trying to figure out the right parameters. For your data to arrive at good clustering, because just every data set is so different. And it's unlikely that just the default settings are always gonna work for every data set, so it's really important to keep track of that type of information. And yeah, it's nice to have that handled for you.
Hannah Schuyler:
I think that that is it for questions. Unless there's any last minute questions coming through. Thank you, Nora, for your input on this. It's great to hear your perspective on single cell.
Kit. You, of course, as well. It's great to hear your perspective as relates to g.nome. And thank you everyone so much for joining. If you have any, follow up questions, feel free to email us. We will send out this recording for everyone that registered and attended. So thank you so much, everyone, and have a good rest of your day. Thank you. Bye.
Moderator
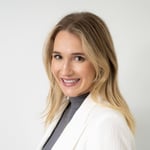
Director of Business Development
Almaden Genomics
Hannah Schuyler graduated from The University of Texas and spent the beginning of her career focused on using digital analytics to inform a marketing strategy for pharmaceutical, biotech, and medical device clients. She has spent the last few years working with clients to help them identify data analysis solutions for NGS and genomic data.
Speakers
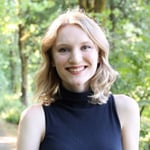
Bioinformatics Engineer
Almaden Genomics
Nora Kearns is a bioinformatician with a specific interest in engineering methods, both biological and computational, which accelerate the research and discovery process. She began her career as a bioinformatician in the cell therapy industry, building pipelines to process data from high-throughput design assays in immune cells. Since joining Almaden she has focused on developing automated workflows for analysis of Single Cell RNAseq data on g.nome. Nora completed her master's in Bioinformatics and Genomics at the University of Oregon where she worked in a molecular engineering lab focused on developing high-throughput assays for protein characterization.
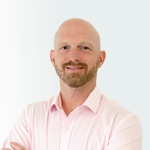
Senior Director of Product Development
Almaden Genomics
Kit Fuhrman is an experienced product manager in the biotech industry. Dr. Fuhrman has built impactful products ranging from NGS sequencing reagents for translational scientists to spatial biology platforms for pioneering researchers. He has a doctorate from the University of Florida in Immunology, studying the role of regulatory T cells in type-1 diabetes, and a master's degree from the University of Central Florida, studying HIV entry inhibitors.