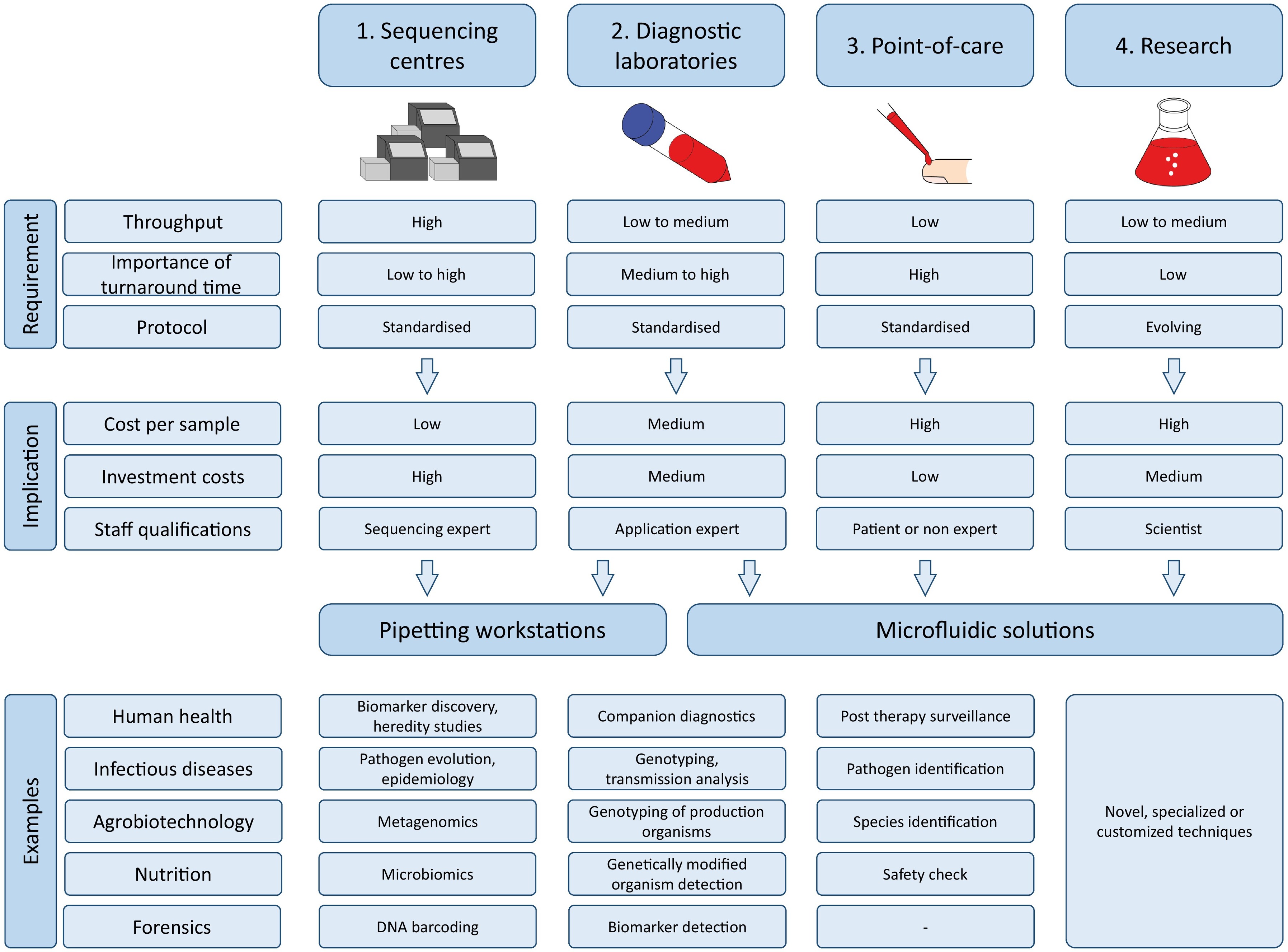
Overcoming Single-Cell RNA-seq Challenges in Cancer Research
Key takeaways:
- Single-cell RNA sequencing is transformative with potential but faces technical challenges.
- High costs make experiments expensive, limiting the number of samples and making scaling up cost-prohibitive.
- Interpreting RNA-seq data, especially from multiple sources processed differently, is challenging. Ensuring data comparability and understanding each dataset's nuances is crucial.
- The power of single-cell RNA-seq lies in transparent data sharing and correct interpretation.
- Collaborative efforts and consortiums are crucial in advancing single-cell RNA-seq, ensuring data processing, sharing, and interpretation meet high standards.
Single-cell RNA sequencing is a transformative technology brimming with potential. However, its true power is hindered by technical challenges. Scientists and bioinformaticians face difficulties ranging from sample preparation to ethical issues. Interpreting RNA-seq data intensifies these challenges, especially when it comes from multiple sources that are processed differently. It's vital to ensure data comparability and grasp the nuances of each dataset.
The true strength of single-cell RNA-seq isn't just in data generation but in its transparent sharing and accurate interpretation. By adopting innovative strategies, we can bridge the gap between its potential and practical use, ensuring it maximizes its impact in modern biology. Transparent sharing promotes collaboration and accelerates discoveries while preventing misinterpretations that can lead to incorrect conclusions.
Challenges Facing Scientists in Single-Cell RNA-Seq Analysis
Scientists encounter numerous challenges when using single-cell RNA-seq for cancer treatment, making the approach particularly interesting, intriguing, and frustrating. In addition, the entire process contains a tremendous amount of complexity. Some of the most common challenges of this type of analysis are:
- High costs: The experiments are expensive. Preparing a single sample for analysis, based on reagent costs, can reach tens of thousands of dollars. This limits the number of samples for study and hinders scaling.
- Technical noise: This problem can be caused by various factors, including the new techniques used in producing libraries for sequencing.
- Signal amplification: The action is necessary due to the limited genetic material in a single cell, which can introduce bias.
- Isolation Process: This process can change the cell's state, introducing misleading signals. Some sensitive cells may also die during isolation, preventing their sequencing.
- Batch Effects: These factors arise from varying conditions like time, temperature, and handling during sequencing.
- Cellular Heterogeneity: Even clonal cell populations that share properties exhibit this.
- Data Complexity: Analyzing the data requires specialized skills and resources.
- Ethical Considerations: Samples might unintentionally provide insights into the health of patients and their families that may not have been the intent of the study under review.
Interpreting data from varied sources adds to the challenge, emphasizing the need for data comparability and understanding dataset nuances. The significant costs and challenges associated with scaling single-cell RNA-seq act as barriers to its broader adoption.
Overview of four different sequencing scenarios for library preparation for next-generation sequencing (NGS). The four scenarios differ in terms of associated requirements, namely throughput, protocol, and importance of turnaround time. These requirements result in varying implications for each scenario. Cost per sample, investment costs, as well as staff qualifications, must be considered for efficient automation of the respective scenario. A selection of potential examples for different domains is sketched at the bottom. The examples for sequencing centers and diagnostic laboratories are already applied in research and industry, whereas those for the point-of-care are predicted opportunities of NGS. Notice that microfluidic solutions can be an attractive option in sequencing centers, and pipetting workstations can be used in research. In general, there is a tendency to use pipetting workstations for high and microfluidic solutions for low throughput applications. Image, caption source: Hess, J. F. et al. Library preparation for next generation sequencing: A review of automation strategies. Biotechnology Advances 41, 107537 (2020). Creative Commons license |
Strategies to Address the Challenges
Innovators are developing strategies to tackle the challenges of single-cell RNA sequencing. Companies like 10X Genomics are leading the way by introducing microfluidic devices and kits that simplify sample preparation. These innovations not only increase the scale of cells but also reduce costs, making the production of single-cell data more cost-effective.
The field is evolving to focus on isolating complex cells, such as tumors and neurons. While sharing data offers its own set of challenges, both competitive and technical, the emphasis remains on transparency and reproducibility. Introducing genomic techniques into clinical settings calls for rigorous standards. Collaborating with other institutions and pooling resources allows us to combine our strengths and cultivate a shared knowledge base.
Collaborative efforts, especially consortiums, are crucial in advancing single-cell RNA-seq. The highest standards in processing, sharing, and interpreting data are ensured by pooling data, resources, and expertise, effectively bridging the gap between potential and practical application. Projects like the Human Cell Atlas aim to consolidate data from various studies, championing the "fair data" concept that is findable, accessible, interoperable, and reusable. Addressing this challenge calls for a blend of biology, computational algorithms, and scalability expertise.
Tools and Techniques to Adopt
To combat high costs, we need a shared data model leveraging public and private sources to create comprehensive datasets. While this model reduces costs, it also introduces challenges like noise and batch effects.
We must ensure data comparability across diverse sources, regardless of their methodologies. Large consortiums now set benchmarks for dataset comparability and offer normalized data. Research publications in the field are surging, highlighting its growing importance. Innovations range from improved sample preparation to novel analyses and applications.
Cutting-edge technologies such as spatial omics and multi-omics are leading advancements in the field. Spatial omics sequence tissues to visualize their 2D or 3D architecture, while multi-omics integration aggregates data from various high-throughput methods.
Each tool and technique is evolving individually, but the true challenge and opportunity lie in seamlessly integrating their analyses for a holistic understanding. While the focus often lies on the application of single-cell RNA-seq in cancer research, the technology revolutionizes various fields of biology.
Simplified graphical representations of the three key spatial omics technologies, namely (A) spatial proteomics assays for in-situ single-cell phenotyping using surface marker; (B) H&E staining for histomorphological assessment, and (C) spatial transcriptomics for in-situ transcriptomics characterization, and representative TME analyses enabled by AI bioinformatics. Image, caption source: Lee, R. Y. et al. The promise and challenge of spatial omics in dissecting tumour microenvironment and the role of AI. Frontiers in Oncology 13, (2023). Creative Commons license |
Envisioning the Future
The future of single-cell research hinges on data comparability and sharing. We must establish a shared data practice that promotes transparency, best practices, and thorough documentation. Our analyses must be transportable and reproducible. While challenges like technical noise and data complexity exist in single-cell RNA-seq, open-sharing practices can address them. This collective approach can push the field's boundaries.
However, we need to strike a balance. Academia pushes for openness, while the corporate world often guards its proprietary interests. Even within academia, some individuals withhold their computational methods for competitive advantages. We must balance these individual interests with the overarching aim of advancing the field.
Moving to an Accepted Standard
Single-cell RNA-seq is a pioneering technology with vast potential. Yet, its full capabilities are constrained by technical and ethical challenges. While data sharing is common, the methodologies behind conclusions often remain ambiguous. As we look towards its clinical applications, regulatory oversight will be crucial. In the open-source domain, the challenge of setting uniform criteria persists, with the emergence of various "standards" underscoring the absence of a universally accepted protocol.
Read more about single-cell RNA seq here:-
The Ultimate Guide to Public RNA-Seq and Single-cell RNA-Seq Databases: A Comparative Review
-
scRNASeqDB - Database for gene expression profiling in human single cell by RNA-seq
Mark Kunitomi
Mark Kunitomi is the Chief Scientific Officer at Almaden Genomics. He was a post-doctoral fellow at UC San Francisco with a background in genomics, bioinformatics and microbiology, and he has a Ph.D. in Biochemistry & Molecular Biology from the University of California, San Francisco.