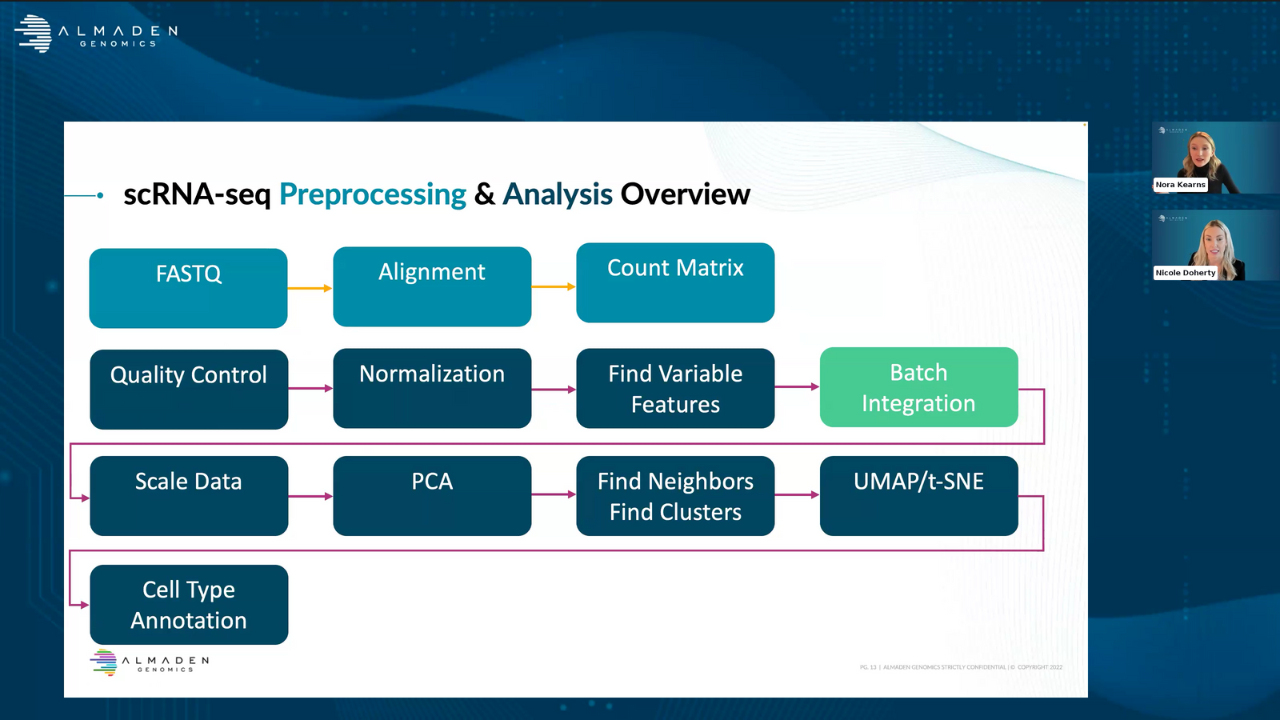
From Instrument to Insight with Single-Cell RNA Sequencing
Single-cell RNA sequencing (scRNA-seq) has emerged as a powerful tool in molecular biology, enabling researchers to delve into the intricacies of cellular heterogeneity with unprecedented resolution. This cutting-edge technology allows for the study of gene expression profiles at the individual cell level, offering insights into cellular diversity, developmental trajectories, and disease mechanisms. As the field continues to evolve, mastering the essentials of scRNA-seq analysis is paramount for researchers seeking to unlock the full potential of their data.
In this insightful webinar, you will learn:
- Data Handling: Learn how to handle diverse data types such as FASTQ, h5, and 10x MEX efficiently, ensuring seamless integration into your analysis pipeline.
- Dataset Access: Discover methods for accessing and utilizing publicly available datasets on platforms like GEO and SRA, empowering you to leverage existing resources for your research.
- Quality Control and Alignment: Master the techniques for effective quality control (QC) and alignment of raw sequencing data, ensuring the accuracy and reliability of your results.
- Artifact Filtering: Explore strategies for filtering out technical artifacts from counts, enabling you to focus on biologically relevant signals in your scRNA-seq data.
- Cell Clustering: Unlock the potential of advanced techniques like Seurat with UMAP and t-SNE for clustering cells, revealing hidden patterns and relationships within your single-cell datasets.
Join us on a journey into the depths of single-cell RNA-seq analysis, where we explore the fundamental concepts and advanced methodologies that drive groundbreaking research in the field. In this webinar, you will gain invaluable insights into the intricate process of handling diverse data types, accessing publicly available datasets, and employing state-of-the-art techniques to analyze scRNA-seq data with precision and accuracy. Whether you're a seasoned researcher looking to refine your skills or a newcomer eager to dive into the world of single-cell genomics, this webinar promises to revolutionize your understanding of scRNA-seq and elevate your research to new heights. Don't miss out on this opportunity to embark on a transformative journey through the realm of single-cell RNA sequencing.
Watch the webinar now and revolutionize your approach to scRNA-seq analysis:
Moderator
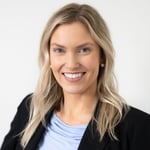
Director of Business Development
Almaden Genomics
Nicole Doherty got her undergraduate degree from UNC Wilmington and started her career at a public relations firm focused on biotech companies. Since completing her Master’s degree in Nutrition and Exercise Science at Montana State University, she has been working with clients to help them identify data analysis solutions for NGS data.
Speaker
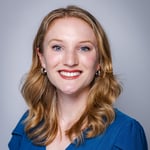
Bioinformatics Engineer
Almaden Genomics
Nora Kearns is a bioinformatician with a specific interest in engineering methods, both biological and computational, which accelerate the research and discovery process. She began her career as a bioinformatician in the cell therapy industry, building pipelines to process data from high-throughput design assays in immune cells. Since joining Almaden she has focused on developing automated workflows for analysis of Single Cell RNAseq data on g.nome. Nora completed her master's in Bioinformatics and Genomics at the University of Oregon where she worked in a molecular engineering lab focused on developing high-throughput assays for protein characterization.
Transcript
Nicole Doherty:
Hello everyone, and welcome to today's webinar: from instrument to insight with single cell RNA sequencing. My name is Nicole Doherty. I'm a Director of Business Development at Almaden Genomics. Joining me today is Nora Kearns, our Bioinformatics Engineer.
A couple of things before we get started. This webinar is being recorded and will be sent out to all attendees after, and will also be available online. Please be sure to enter any questions you have for us in the chat box and they will be addressed at the end during our live Q&A session. On the agenda for today's webinar is a brief overview of Almaden Genomics and how we help researchers analyze different types of genomic data, followed by a deep dive into the essentials of single cell RNA sequencing analysis, such as handling different data types and how to effectively QC and align sequencing data within genomics.
As a software company that's spun out of IBM research. The g.nome software was originally built for an internal project and the team quickly saw the value in building pipelines and workflows for their customers, especially those that didn't know how to code. Today we work with a variety of clientele, including pharma, biotech and academic clients, to help with their data processing, whether through our pre-built workflows, access to curated datasets from our partners, as well as bioinformatics consulting services.
As experimental methods have improved and driven costs down, the size and availability of datasets, especially single cell RNA sequencing datasets has increased. So, one of the first challenges of analysis is data management. Then, biologists are often reliant on bioinformaticians or a bioinformatics core to process their data. This can lead to delays and increase the time to discovery.
There's also a need for transparency and organizational systems to track and reproduce methods. Overall, the life sciences discovery process is very complex, with many moving parts that don't always work well together. g.nome is an omics data science solution that provides scientists with an end to end approach to life sciences discovery. The user’s journey on g.nome begins with the data—connecting to your own servers or public databases.
We provide the ability for users to manage not only the data, but who has access to the data and all the security and logging and storing and processing the data, all of which is hosted on AWS. After processing data through pre-built or custom workflows, scientists can further explore their data with tool generated visualizations or build their own visualizations with Jupyter notebooks. g.nome gives users simplified data exploration, rapid iteration and reproducibility of results.
g.nome was built with the intention of meeting users at their skill level. And on that note, I wanted to host a quick poll to find out about everyone's skill level, experience level with analyzing single cell data, the lowest score on the rating scale meaning I know nothing, and the highest score being I'm an expert.
For biologists or those with less computational experience, we provide guided workflows with a simple form fill interface, guided workflows also come as prebuilt canvas workflows, which offer more flexibility to customize visualizations and results. And for a fully customized pipeline, you can build your own workflows with our canvas builder. Once a workflow is built, it can also be ported to a guided workflow for use by other members of your team. And we'll demo all of these capabilities for you today.
I think maybe everyone's had a chance to answer. There's still some answers coming in. Okay, so let's check and see what we have. So it looks like a pretty wide variety of answers here with the bulk being in the lower level of experience in single cell analysis. So that is awesome to know.
Thank you, everyone. Our goal today is to teach you how to navigate some of those challenges around single cell RNA sequencing, data analysis, and equip you with the tools you need to generate insights from your raw sequencing data. So with that, I'd like to pass it over to Nora.
Nora Kearns:
So as Nicole mentioned, my name is Nora. I'm a bioinformatics engineer here at Almaden Genomics, and I specialize in the analysis of single cell RNA sequencing data.
So to start off, let's just talk about why is single cell analysis important? Why are you here in the first place? Learning how to analyze the data? Well, what single cell RNA sequencing allows us to do is quantify gene expression at the level of individual cells versus an entire tissue. So that's what bulk RNA sequencing lets us do is we can get an average idea of the gene expression across a tissue.
But we know that every cell within a tissue is different. There are really complex relationships between cells going on within a tissue, and so being able to study individual cells is really valuable. Specifically in medicine, you're not targeting a whole tissue—you're looking for a target protein that's expressed by an individual cell for safety reasons and other reasons.
So single cell is powerful because it allows us to better understand mechanism, the mechanism of action of disease as well as mechanism of action for drugs, and identify actionable targets. So single cell has really expanded since its inception. I think the first single cell data set was just a few hundred cells and now we're regularly seeing people sequence hundreds of thousands of cells just as a part of a single experiment.
And everyone pretty much acknowledges that single cell is really valuable and applicable to a lot of disciplines across biology. But there are still some things that have hindered its widespread adaptation into a common practice, and some of those have to do with the analysis of single cell data.
So for one, single cell data sets are really large and complex. You basically have hundreds of thousands of biological replicates that you're trying to sequence, which generates tons of data, and that's computationally intensive. So you often need high performance computing resources to analyze the data. Also, as single cell has expanded, dataset size has expanded, and technology has gotten better for generating single cell data. We've also seen an increase in the number of bioinformatics tools available for processing the data, and that's awesome that we have lots of tools out there now at our disposal.
But there has been kind of a lack of standardization with the analysis. So everybody's doing something kind of different and it makes it hard to facilitate cross study comparison and data integration. Also, with all of these tools, there's a need for bioinformatics expertise to not only understand the biology of the data, but how to process it, what's actually going on during the analysis. And not everyone has the ability to hire for that expertise, particularly if you're at a smaller company or in a lab setting.
So I'm really excited actually to talk today because in addition to bioinformatics, one of my passions is teaching and science communication. And I think especially for bioinformaticians, oftentimes our job is to find the tool and figure out how to use the tool, but the tool can sometimes be a black box, and I don't like to operate that way—I like to understand what's actually going on during my analysis. So that's the goal today, is I want you to develop a framework for how to approach single cell analysis, but also understand what all of these steps are, because every analysis is different.
And when you just know the tools, you don't necessarily know how to adapt and change your analysis when your experiment changes. So just to outline what we're going to be talking about today, we're going to do an overview of the technology, although I'm sure if you're here, you may at least have some idea of what single cell is. So we'll try to keep that brief.
Then we're going to discuss single cell data formats later on. I'm also going to show how to pull data from these public repositories. Then we're going to do an end to end single cell RNA sequencing analysis, and I'm going to demo how to analyze single cell data on g.nome. I think when giving a demonstration or teaching anything, it's also really useful to have an example. So for the sake of this webinar where we're going to be reproducing the analysis from this paper, which did single cell on COVID, flu, and healthy patients and compared them.
Okay, so let's start with an overview of the technology. What single cell does is it allows us to quantify the number of transcripts expressed from each gene and each cell within our tissue of interest. It starts by preparing the sample: you have your cell suspension and then you isolate (or, this is an example from the 10x Genomics kit) but you're isolating individual cells in a little water droplet within an oil emulsion. I think this is just amazing that we can do this. But basically each cell is in its own droplet with a barcode, and that barcode is really important. Or sorry, it's in the droplet with a bead, and that bead is very important because that bead contains barcodes. When the cell is lysed open, the barcode binds the transcript, and now that transcript forevermore has a unique identifier on it that says, I originally came from this specific cell. So once we have all of those transcripts barcoded and ready, they go off for sequencing and then we have our first actual physical data that we can work with for data analysis.
So now let's talk about some of those data formats. So right off the sequencer, what we get is FASTQ format. This is raw sequencing data containing the actual sequences of the transcripts. And I want to specify this example here is from the 10x 3’ v3 kit. There's lots of different kits that you can use to prepare sequencing data, but generally what you're going to get is a read one file, read two file, maybe an index file, but read one and two are really important because read one contains your unique cell barcode and then read two contains your cDNA transcript—if you're a biologist, you may know that RNA is too unstable to sequence itself, so we have to convert it to double stranded cDNA prior to sequencing. So those cDNA transcripts are what actually gets mapped against a reference genome during a step called an alignment.
So we're taking our FASTQ data and we are mapping transcript reads against genes within a genome, and we are counting out how many times we observed a transcript per gene and each cell to develop a count matrix like this one over here. So we observed gene one three times in cell one and only two times in cell two. Once we get to a count matrix, then we start seeing lots of different data formats emerge because bioinformaticians love their different data formats, but I'm just going to go through three of the most standard ones today.
So the first is 10x MEX. This is three files: a barcodes file indicating the cell, features file indicating the gene, and then the matrix with the counts. This is what is outputted by alignment tools like Cellranger and STARsolo. And this is typically how we see unprocessed counts data formatted. Cellranger also outputs an HDF5 format, which is a single file, compressed data format. Both of these formats are compatible in different R tools like Seurat. And then lastly, we have our H5AD, which is a single file. And what I wanted to point out about an H5AD file is that it's useful for storing lots of metadata information. So you tend to see already processed data in H5AD format. This is also kind of the Python-specific format if you're working with the library scanpy to analyze your data.
But H5AD consists of a count matrix, this green box here, and then you have a variable matrix which contains the genes as well as metadata information about the genes such as a what type of gene it is, for example, and then an observation matrix with your cell barcodes and then metadata information about those cell barcodes.
I think what I want your takeaway from this slide to be is there are a lot of formats out there and you have to keep in mind what you want to do downstream with your data so that you're using the correct format. For example, let's say you want to use a tool that wants an H5AD file. So you might just want to start off using scanpy and generating an H5AD because the interoperability between these file formats is actually not as easy as we would like it to be.
All right, so now let's go through an end-to-end single cell RNA sequencing analysis. Some really great advice that I got from a data science friend of mine is that it's good to start your analysis with the end in mind. And by the end we're talking about the figures, which is what everybody wants to see.
And so for this analysis, we are going to generate a couple different flavors of t-SNE. What is a t-SNE? A t-SNE is a data visualization method that allows you to visualize very high dimensional data in low dimensional space. When I say high dimensional data, I'm talking about each of these cells has tens of thousands of genes that it's expressing, and each of those is a variable. So how do we take all of these variables and then condense it down to something that we can actually look at and digest? So that's what t-SNE does is on a t-SNE, the axes really are arbitrary, they are representations of many, many, many variables kind of behind it.
What's really important to know about t-SNE is that on t-SNE, proximity is a measure of similarity. So on this plot, each point represents a cell and points that are very close together are cells that are similar, of the same type; cells that are apart or that are far apart from each other are really different and of different types. So the types of t-SNEs that we're going to generate are this one colored by disease type, this next one we're going to look at is a t-SNE colored by expression of specific genes, then we're going to look at a t-SNE colored by cell type, and then lastly, we're going to look at proportion of each cell type in the different conditions.
Okay. So now we know what the end of our analysis is going to be. Let's back up and talk about the starting data. So we are going to start from the very beginning in FASTQ format. So we have 20 samples, each sample has an R1 and an R2 FASTQ file, and those samples consist of four healthy individuals, five flue individuals, and then 11 COVID individuals. And as I mentioned, it's in FASTQ and the specific sequencing chemistry is 10x 3’ v3.
So how do we actually do this analysis? I'm going to start with just this high-level overview. These are all of the steps that we're going to walk through. As I mentioned before, you know, a lot of times bioinformatics can be this black box. We're using these tools that we don't necessarily know how to use them. Each box here corresponds to an actual step that you would use in a Seurat analysis.
Seurat is a very common package used for single cell analysis, and on our platform g.nome, this is what we use for our analysis as well. On our platform, we like to break analysis down into pre-processing and analysis. Pre-processing is just going from a FASTQ file to a count matrix by performing an alignment. This typically is, you know, you're doing it in one go.
You should only have to run it once as long as you know everything goes well and as long as you're using the same kit, the parameters that you're going to use are the same. But this stuff is computationally intensive and can take some time. So we like to just isolate that, get it done. And then the analysis is where people tend to spend more time tweaking parameters and changing things.
So we have that as a separate set. So as I mentioned earlier, during pre-processing, really what you're doing is you're just taking these raw sequencing reads and then aligning them against a reference genome and developing a count matrix of number of transcripts per team. First of all, the tools that I recommend using to do this are Cellranger and STARsolo. Cellranger is the 10x software and then STARsolo is an open source method designed to reproduce the results of Cellranger, but at significantly faster speeds.
STARsolo has settings for all of the different 10x kits, so that's really nice. And for my bioinformatics friends, if you're thinking what about duplication and adapter clipping, those are both included in STARsolo and there are specific settings depending on the mkit chemistry that you're using. An adjacent step that should be going on here is FASTQC, so that's quality control of your actual raw sequencing data. But I'm just specifically talking about, I don't want to spend too much time on FASTQC, but always recommend doing that.
So now that we have our count matrix, we are ready to start our analysis and every analysis should begin with quality control of your counts data. So I'm going to walk you through five metrics that are good to look at when performing QC. The first is genes per cell—so we want to make sure that our cells are actually expressing something. So typically a good threshold to set here is about 200. You're going to filter out any cells that express fewer than 200 genes.
Another good metric is number of UMIs per cell, so number of unique transcripts within each cell. And a good metric to set there is around 250-500. We want to see a higher distribution of UMIs than genes per cell. Another interesting one is percent of mitochondrial genes per cell. So mitochondrial DNA and high volumes of mitochondrial DNA can be indicative of cell stress and cell damage because the mitochondria has its own little membrane that keeps it safe.
So we want to filter out any cells where we're observing large quantities of mitochondrial DNA. So a good filter to set there is anywhere from 10 to 20, it depends on the cell type—with PBMCs, you can usually go up to 20. With other cell types I'd recommend somewhere from 10 to 15. This data here looks pretty good and I believe the authors set their threshold at 15.
Second to last, we have complexity. So by complexity I mean a measurement of the diversity of the transcriptome within a cell. We want to make sure that lots of different genes are being expressed, it's not just, you know, one single gene. So a good threshold that I would set here is around 0.8. And I think in Seurat, actually the main ones that they advise you to set are just genes per cell, UMIs per cell, and mitochondrial DNA. So this is kind of going the extra mile.
And then the last one that we can look at is the ratio of genes to UMIs. What this actually lets us do when we're when we're looking at this plot, what we should be looking for is patterns of of cell clusters. So in good, high quality data, we see kind of a nice clean line here. But you should watch out if you see kind of different clusters breaking out just based on technical effects or technical metrics alone. It's just something to keep an eye on.
Once we've performed QC, we're ready for normalization. So during normalization, what we're doing is we are dividing the feature counts per cell over the total counts per cell, and then multiplying by scale factor. What this is doing is it's accounting for the varied sequencing depth across cells. So just because of the way experimental preparation is, some cells get sequenced higher than other cells and normalization allows us to correct for that and kind of make cells equal before we begin our analysis.
Okay. So the way that I like to describe single cell, I think a good analogy for it is that it's a funnel. You're starting with a ton of high dimensional data and you are funneling it down to something that's actually digestible and interpretable. And there's a couple of different steps that we use to do that. The first one was quality control, where we just eliminated the junk and next is fine variable features where we can eliminate genes that don't actually tell us anything interesting about the data.
So, you know, even though cells are different, sometimes they express the same genes and they don't tell us anything about the diversity of cell types. So one of the steps we can use to pare down our data is find variable features. The default is typically to take the 2000 most variably expressed genes forward in your analysis and then leave the rest.
When I first started working with single cell data, I watched this talk from someone who was doing their PhD on batch correction. So yeah, batch integration, people will spend entire seven year PhDs on this and we're super lucky that we get to benefit from the tools that they generate that make our lives easier so that we don't have to do seven year long PhDs on that integration.
But I'm going to walk you through some tools that you can use and also what's actually going on under the surface when we run those tools. So I took this example from a paper, sorry, I should have linked it here because it's actually a great paper on benchmarking batch effect correction methods. But they took two samples, one that was done on Microwell Seq and another that was done on Smart Seq, and we see that these these cells break out into different clusters just based on the technology that was used to, you know, process them, which we don't want to see.
So then the authors performed a batch integration or batch effect correction and batch integration using Seurat 3 and Harmony. And you can see, okay, now these cells are overlapping a lot more, which we'd expect. You know, there there are the same cell types in each batch. We should see them overlapping more.
Now what's actually going on under the surface here, at least I'll speak to Seurat 3 is that it's taking these clusters of cells based on batches, and it's identifying cells that demonstrate similar expression profiles. but they may have shifts that are accounted for by the technical batch effects. So saying, okay, this cell over here actually has a really similar expression pattern to this other cell in this other batch, I'm going to use those cells as anchors to correct for the batch effects and merge them together.
And I should say you only perform batch integration if you have multiple samples, of course. But then also this is typically if you have data that was produced off of different sequencers, maybe different slightly different experimental preparation, definitely across different labs, and if you're trying to integrate like a public dataset with your own data set, it's a good idea to do.
But I also recommend if you want to perform batch integration on your own data, let's say that you have one person prepping all of your samples, they're all treated the same way. It would be a good idea to do batch integration or batch effect correction and batch integration, but also just try merging the data because you may not actually have batch effects there and you don't want to overcorrect and actually lose some true biological variation between your batches because you're trying to remove batch effects.
So the next step is scaling the data. Typically, the reason that we do this is to ensure that highly expressed genes don't dominate the analysis downstream. Typically, we see that highly expressed genes also demonstrate the highest variation in expression. And so what we do is we scale the variation with the expression level. So what this involves is shifting the mean expression of each gene across cells to zero, as well as shifting the expression of each gene across cells so that the variance is one. Really the takeaway here is just we want to give high expressing genes and also low expressing genes that do demonstrate interesting variance, more equal weight.
So the next step is another reduction step. So remember, that's at the top of our funnel. We got rid of the junk data. Then we eliminated genes that were interesting to us. Next step is a dimensionality reduction step called principal component analysis (PCA). So what principal component analysis does is it takes all of your input variables and then it develops new variables that represent the starting variables. And these new variables are called principal components. Each principal component represents a linear combination of all of your starting variables, so your genes.
And so principal component one, over here we have this PCA by principal component one is one representation of all the sorting variables. Principal component two is another representation of all the starting variables. And as you move down the number principal, but one principal can put two, three, four or five, the early principal components or principal component one explains most of the variation in the data, and then it gets less and less and less as you move on.
So one way of visualizing that is an elbow plot, which I have done here. Hopefully it's not cutting off at the bottom of the screen, but as you can, as you see, as you move forward or to greater levels of principal component, it explains less of the variation in the data.
So once you have done a PCA, you're going to select a number of those principal components to take with you for the rest of your analysis. And one way of doing that is by looking at this elbow plot here and observing where it kind of stagnates and keeping more and more principal components doesn't really help you in terms of preserving variation in your data. So if I were looking at this elbow plot, I would probably set my threshold somewhere between 13 and 15, another one that you can use.
Another thing about principal analysis is it's always a good idea to just take a look at your PCA plot because you'll see like once you do a PCA, are my cells starting to break out into unique clusters because if you do a PCA and you're just seeing all of your cells in like one blob, that's a sign, an early sign that things are probably not going to go super well from you from that point forward.
So the next steps kind of go hand in hand, and find neighbors happens before find clusters. But during find neighbors, the find neighbors tool is taking in the principal components that we chose from the PCA step, and it's using them to generate something called a shared nearest neighbors (SNN) graph. What this is, is a representation of the local neighborhood relationship between cells based on those input principal components. The graph contains two things: nodes and edges. Each node is a cell, and then the edges represent connections between those cells, and the edges are weighted based on the number of shared connections between a node and its connecting node.
I think a nice analogy to sort of simplify that is, let's say that you are a wedding planner and you have to design a seating chart for a wedding and you have a bunch of circular tables with whatever, you know, ten seats or something you want, or you wouldn't want to put person A at a table with person B, and person B is the only person person he knows and person B knows the rest of the table because then that would just be weird for person A. You'd rather have a table where most of the people kind of know each other. So you want tables that grouped together really nicely.
The important parameter here is K, which is the number that you would expect your smallest cluster to have. We take the output of find neighbors, which was that SNN graph, and pass it into the find clusters step. So what find clusters does is it takes the SNN graph and it uses it to identify the best communities which minimize the distance between nodes within a cluster. So just this example over here we have these five nodes that connect really well to one another, but then this node over here also connects to this node over here and this node over here next to this node over here.
But reasonably, what find clusters, let's say, does is you know what, these cells cluster really well together, I'm going to group them together. And the important parameter here is resolution. Typically the resolution or I think the default resolution in Suerat is like 0.6 or 0.8. But what's important to know is that the higher resolution you set means that you would expect to see more smaller clusters, whereas the lower resolution that you've set, you're going to see fewer big clusters.
So now we're at our really exciting stuff, which is generating this beautiful canonical UMAP or t-SNE plot that's associated with single cell analysis and UMAP or t-SNE is similar to PCA, is a dimensionality reduction step, but it's slightly different than PCA. PCA is really designed for dimensionality reduction data analysis, UMAP and t-SNE are more optimized for data visualization. So one of the ways that they're different is that t-SNE allows you to represent nonlinear combinations of the relationships between input variables. So what's actually going in to UMAP or t-SNE are those principal components that you selected to carry with you. And so t-SNE one is a nonlinear combination of all the principal components and then t-SNE two is another nonlinear combination between all the principal components.
But what's important to know about t-SNE and UMAP is that they're really designed for data visualization because they're designed to optimize the condensing of clusters and the distributing of neighborhoods. So they're trying to form the tightest clusters with the greatest distance between them.
Lastly, if you're a biologist, you're like dying to know what those actual clusters are, so you'll probably want to do a cell type annotation at the end of your analysis. The way that this has traditionally been done is with manual annotation based on marker genes. So you're looking at how a cluster actually expresses a marker gene like CD14 if you're trying to annotate monocytes. But as you can imagine, that can be, you know, painstaking and slow, especially if you have lots of clusters. So more recently we've seen tools developed for automated cell type annotation.
What we use on g.nome is called SingleR. SingleR is automated reference based annotation, so it takes an already annotated reference dataset and it compares it to your own annotated reference dataset. And based on a similarity comparison between the two, it assigns labels from the reference dataset to your unannotated dataset.
Okay. So let's take a step back again. This is kind of our guide to single cell analysis broken out into pre-processing and analysis. One thing that I want to mention here is that I think this makes it look like it's just one clean line, but every experiment is different and you have to make choices during your analysis that are going to change the course of the analysis or how it could kind of branch off. So one of the features that I want to show you on g.nome is how we handle that for you.
Okay, so now it's time for a demo of g.nome using this paper. So I'm just going pull up g.nome. So now I'm in my g.nome workspace. I have set up a project for us to work in, so I'm just going to go into that project and then head over to the data tab.
So every analysis begins with grabbing your data. The authors publish their data in two places that I want to talk about. The first is GEO, which is for Gene Expression Omnibus, this is typically where we see counts data posted, so we can see down here they have the 10x and the barcodes features as a matrix file. One thing that I kind of caution about, if you're trying to reproduce analysis and you choose to start from the counts data, is that you might not know what exactly is there in the counts data, whether it may already have some preliminary filtering done to it.
In this case, they've already integrated the data. And so if you truly want to reproduce the analysis from the beginning, it's best to start over on SRA, which is where people host FASTQ files publicly. So SRA is Sequencing Read Archive. And so I've just navigated here and I can see all of 20 of the runs as well as some metadata associated with the runs.
So I'm going to download this accession list and then head back over to my g.nome workbench. You can see I've downloaded this many times and then go to “Add” and select a local file, and then I can just choose this accession list that I just downloaded and import it to my g.nome workspace. So I'm going to head back over to my modules page and select this SRA import tool.
Go to edit workflow and Builder and then grab this accession list. And I've already connected an accession list here, but you just connect it to the required input. I do briefly want to touch on what's actually going on here. So on g.nome we host a bunch of different bioinformatics tools. Some of those tools are the SRA toolkit tools, and so by popping out this window, you can see the actual tools that are going out and fetching that data from SRA and dumping it into the g.nome workspace.
So then I'm going to head over to my “Runs” tab and I've already done this SRA import and I'm going to go into outputs and I can see all of these FASTQ files listed here. So all my samples, the read one and read two files for me. I do want to touch on two things. One is you can see that this took 15 hours and that's because all of these files, even in their compressed format, are, you know, 20, 30, 35 gigabytes, and the entire dataset is almost a terabyte of data.
So you could try to do this locally, download it on your local laptop, but I definitely would not recommend doing that.
Nicole Doherty:
Nora, can you explain how you would pull data from GEO into g.nome?
Nora Kearns:
Yeah, so GEO, because, because it's in counts data format, which is typically much smaller, what I would do there is I would just download the files in GEO format locally and then head back to the data tab and add those local files.
And we have a tool that allows you to upload a folder. So I just put those files in a folder and upload it to your g.nome. Yeah. The reason that we have the SRA tool for sequencing data is because you would never want to download or take a very long time to download, you know, 35 gigabyte file or a terabytes of data to your local machine and then have to push it up to g.nome.
Okay. So now that we have our FASTQ files, we're ready to actually start our analysis. So I'm going to head over to my guided workflows page and click or run a guided workflow and then select scRNAseq pre-processing. So this is that alignment stuff that we talked about earlier. You can start with FASTQ data or gene counts data. This is going to do some preliminary filtering actually, so you can start with either one. And then next week we are coming out with the ability to work with 10x MEX data in the guided workflow. So I've actually just created a small data set so that you don't have to watch me work on 20 different samples.
I’m just going to go in and select that data set and then select the right sequencing and chemistry. I'm working with 10x 3’ v3, and then I'm going to make sure that my files are paired correctly. These sample names are not super informative to me, so I'm going to replace them with COVID one, COVID two, flu, and healthy.
Then I'm going to assign some metadata. so I know that I have a COVID mild, COVID severe, flu, and healthy. I'm just going to assign each of these to their correct condition and then click next. Then I'm going to select the reference genome to align my sequencing rates against. So I know that I'm starting with human data. We host the Cellranger human reference as well as the mouse reference, but if you are working with a different organism, you can upload your own genome fasta and .gtf file.
And then lastly, we're going to set some optional preliminary filtering parameters. So this is eliminating cells that basically express nothing and genes that are observed nowhere. So parameter one is filter out features found in fewer than x number of cells. I know that the authors set this to one. They just filtered out any genes that weren't observed in any cells. And then in features, so filter out cells that have fewer than x amount of genes. I mean, they set this to 200 and typically what you see here is three for cells and 200 for features.
So then we're going to review and launch the run. I've done this cooking show style, so I already have one for us to look at. So I'm just going to head back to my guided workflows page and then I can go into this pre-processing run and view my results. So I can see that I have my counts, QC report, as well as STARsolo reports, some multiQC information. So I'm going to dive into what those results look like, if I can get this zoom window to disappear. Okay, so here's our first STARsolo report. This is just some some information about the alignment itself. Let's see if I can get this pesky zoom bar away.
Okay. And then our next report is our count QC report that shows us the number. And again, I just did four samples here, but the number of cells observed in each sample and the distribution of genes per cell, all these nice QC graphics that I spoke about earlier. So once we have done our preprocessing, we're ready to get into the true analysis portion of things.
So I'm going to head back over to guided workflows and select run a guided workflow, and then I'm going to choose a RNAseq analysis. So in the analysis, you're going to choose the counts data that you generated in a previous pre-processing run. So I'm going to click this pre this run that I just showed you and then my QC graphics will appear and I'll use those to choose what filtering parameters I'm going to set.
I know that there's lots of different parameters here that you can set, but I know that in this paper the authors just set percent mitochondrial genes to 15 and then the minimum genes per cell to 200, and then they're going to apply the same filters to all of the samples. So it's generally a good idea to try to apply the same filtering parameters across your samples.
You can actually introduce batch effects by treating each sample differently and filtering it a different way. Obviously, there would be some cases where maybe one sample is just worse QC than others and you may have to treat that a little differently. But based on what I'm seeing here, I think all of these samples demonstrate pretty good quality and I'm going to apply the same filters across them.
Then there's an option to regress out the effect of cell cycle genes. So when we get to the end of our analysis and we have a nice t-SNE or UMAP plot, we ideally want to see those cells break out into clusters because they are biologically similar, because they're the same cell type. But there are many reasons why cells may be biologically similar, and one of those sources of biological variation is that they express the same cell cycle genes because they're in the same phase of the cell cycle.
So there's an option on g.nome to regress out the effect of the cell cycle. And you can do that with the default Seurat human cell cycle genes or the mouse homologues or supply your own input genes. But I know that in this paper the authors did not do this step. Then you can choose to perform integration and batch effect correction we talked about earlier.
On g.nome, we host two different options for performing integration. One is with CCA, which is canonical correlation analysis and the other is a reciprocal PCA. So let's step back and talk about what that means. So CCA is Seurat's default method and it's typically best for samples where you're anticipating seeing conserved cell types across samples, but they're demonstrating shifts in expression based on experimental conditions.
The other option is rPCA, which is, as I mentioned, reciprocal PCA. And this is significantly faster than CCA because it's using principal components instead of the count matrix. So it's basically working with smaller data, I guess is one way of putting it. And it's also a lot more conservative. So as I mentioned before, one of the dangers with performing batch of a correction is that you actually remove true biological variation.
So rPCA is more conservative and less likely to do that. The authors in this paper used CCA integration, that's what I'm going to choose. And then lastly, we are going to choose to perform cell-type annotation. So I know that the authors of this paper did manual annotation with marker genes, but I'm going to use g.nome’s tool, which is automated cell type annotation with SingleR against a reference dataset.
And I actually tried two different ones for this, I’ll select the database of immune cell expression data as my reference. So I'm going to select or review and then launch my run again. I've already finished this run, so I would just go into my analysis view results and I'm going to walk you through some of the results that we output from our analysis.
So each run gets its own reports for both The batch effect corrected integrated data well as the individual samples and there's lots of good content in these reports. But to start, this is the t-SNE that I walked us through earlier so we can see on the t-SNE, clusters of monocytes, of t cells, k cells, as well as b cells and we can walk back over to the figure that we started with and we're seeing the same things, so B cells, CD4+ and CD8+ t cells and k cells, monocytes.
One of the things that I want to mention here is that t-SNE and UMAP are both non-deterministic, so both non-deterministic methods. And what that means is that even when you use the exact same and do the exact same analysis, you will end up with slightly different, a slightly different appearance to your t-SNE or UMAP from time to time, from one analysis to the next.
And that's because there's kind of an element of randomness there. And unless you are within one single session and you set a seed, you're going to see that across your t-SNEs. So a good way to measure if you're basically reproducing the exact same thing from time to time, isn't if you're taking a look at the exact same, it's if you're seeing similar kind of groupings of cells like proximity.
So you're seeing, you know, the monocytes break out and you're seeing the t cells and k cells break out, similar proximity between clusters and things like that. And then we can also see how Seurat identified these clusters down below. And then lastly, we have our t-SNE colored by our specific patient.
The next one that we wanted to reproduce was this distribution of cell counts. So we have them here by patient as well as condition. And so we can look here and see the proportion of different cells within each patient. And one of the insights from the paper was that COVID and flu patients, specifically severe COVID patients, tend to demonstrate a higher proportion of monocytes in their cell population, their PBMC population, than in normal, which we're seeing here.
So in normal with five patients, it's about 30% flu, 52% COVID, severe 43%. So kind of confirming the analysis that we saw in the paper. And the last one I wanted to talk about is interactive gene visualization so we can go in and look at some of those marker genes that are interesting to us.
This is CD14, which we tend to see associated with monocytes. Go to this dropdown and select another one. Look at CCR7, for example. I think this is associated with B cells. So yeah, just kind of a nice way to see how we track your cell types. This, as I mentioned earlier, is also traditionally been used to perform cell type annotation.
So that's kind of the overview of the guided workflows and what they produce. The last thing that I wanted to mention for my bioinformaticians is, you know, maybe you were thinking, okay, like that's a good amount of steps, but I know that there's actually a lot more options, a lot more things that we could tweak, and we know that too.
So what you can do is go into the canvas version of the workflow. So this is our canvas plug and play and then you can pop out to the complete workflow and see exactly what steps are going on under the surface. And each step is represented as a tile. So we have a filtering tile, we have that normalization step, the fine variable feature step, so all the steps that I talked about earlier in our analysis overview. And so if you do want to go in and tweak something, let's say that you want to go over to find neighbors or find clusters and tweak K or the resolution, you can do that. And then those changes will propagate to the guided workflow in the future within that project space.
So remember that this is our starting goal. Nicole Is this the right screen that we're seeing?
Nicole Doherty:
Yeah. Yes. Thank you. That was awesome. Great presentation. We did receive several questions, so we'll address as many as we have time for, so we can just jump right in. The first question that came up, is there a reason to use Seurat over Scanpy or are there situational reasons to use one or the other?
Nora Kearns:
Yeah. So great question. So I personally prefer Seurat, Seurat is R based, I know some people don't like R, which is the main reason to use scanpy but yeah. Seurat, is R based, I think a lot of the single cell community is just R based, I think because of that, there are lots of R tools for single cell analysis.
I also think that if you're starting out, the Seurat vignettes, there's a lot of great Seurat vignettes about how to do different portions of the analysis that are super helpful. And I personally just find their documentation to be a bit better than scanpy. So that's my personal preference. But yeah, one of the things I mentioned earlier is with data formats, so scanpy uses and data objects as opposed to Seurat, which uses a R data object. So if you're working with tools downstream that require an H5AD file as input, it might be a good idea to kind of start in scanpy and use that.
Nicole Doherty:
Next question—how do you decide how many principal components to use?
Nora Kearns:
Yeah, so I think I had a slide earlier. So I kind of mentioned like the elbow plot and scree plot earlier, but a good metric is to try to keep the number of principal components which preserve 90% to 95% of the variation in the data. So again, if I was looking at this elbow plot, I would basically choose the number of principal components on this x axis where it starts to level off.
So in this plot, if I follow it down, I can see that it starts to level off somewhere between 12 and 15. So I would take I would probably it's a good idea to error on the high end. So if you're between 12 and 15, take 15. I think one of the insights from the Seurat maybe this right paper or this documentation is that yeah, one you want to on the side of keeping principle components as opposed to getting rid of them and then it actually doesn't change your outcome. Like let's say if you did 15 or 16 principal components, your t-SNE is probably not going to look super different in the end as long as you have enough principal components to start with.
Nicole Doherty:
Let's see, what is the difference between finding clusters and UMAP?
Nora Kearns:
Yeah, so that's a good question as well. Finding clusters is for assigning the cells to actual clusters, and it's using that SNN graph that we talked about earlier to identify those clusters. So that's really a data analysis tool, and then UMAP and t-SNE are data visualization tools.
Nicole Doherty:
Okay, awesome. When you use automated annotation, how do you know annotations are correct?
Nora Kearns:
Yeah. So let me pull up another graphic. So that the specific tool we use is called SingleR, and when you do annotation with SingleR, it generates this nice annotation score heatmap, and on this heatmap you can see the actual labels that were assigned to the cells. And then the color on the heatmap indicates the confidence that a cell was a certain cell type. So for example, this label here is NK cells and we were pretty confident that these cells were NK cells. Basically each line down the x axis of this plot is a cell and then the other potential cell types that could have been assigned to it.
So with NK cells, pretty confident it was an NK cell, but it could have also been a T cell. And we actually we kind of expect that because NK cells and T cells demonstrate similar. So I would look at this plot and I would say, you know what, I am fairly confident that these annotations are good.
If I looked at this plot and it was just like a sea of green, I would think, okay, this probably wasn't a good reference dataset to use. It's not very confident that the annotations are what it assigned it to be. Let's try a different one or try manual annotation.
Nicole Doherty:
Awesome, Awesome. Thank you so much. We do have several more questions and we are out of time. So if your question wasn't answered, we will follow up with you directly and then of course, happy to have a more in-depth conversation if that simple email doesn't answer your question all the way. So thank you all so much for joining us today. I thank you again, Nora, for that wonderful presentation. And we look forward to speaking with some of you soon. Thanks, everyone. Bye.