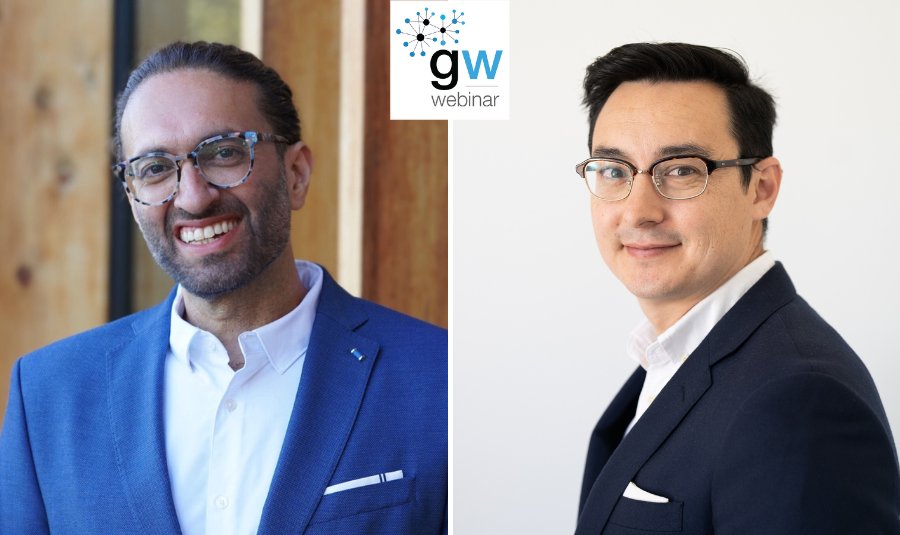
Mastering Imputation for Complex Trait Analysis
This webinar delves into the various techniques used for imputation and analysis of complex traits in genetic research. Expert speakers describe imputation methods for filling in missing genotype data, including phasing and imputation using reference panels, and guide attendees on how to optimize imputation workflows. The speakers also explore different complex trait analysis methods designed to support rapid genetic analysis and hypothesis-testing by researchers, regardless of programming experience or technical background.
- Address research roadblocks. Complex traits are traits that are influenced by multiple genetic factors, making it difficult to study them using conventional methods.
- Do more with less. Imputation is a statistical method that enables researchers to fill in missing data in genotype datasets, allowing for more comprehensive and accurate analysis of complex traits.
- Test different imputation strategies. There are several imputation strategies and test methods available. We will highlight a few.
- Validate imputed data. It is important to validate the imputed data to ensure that it is of high quality and that the results of the analysis are reliable.
Speakers
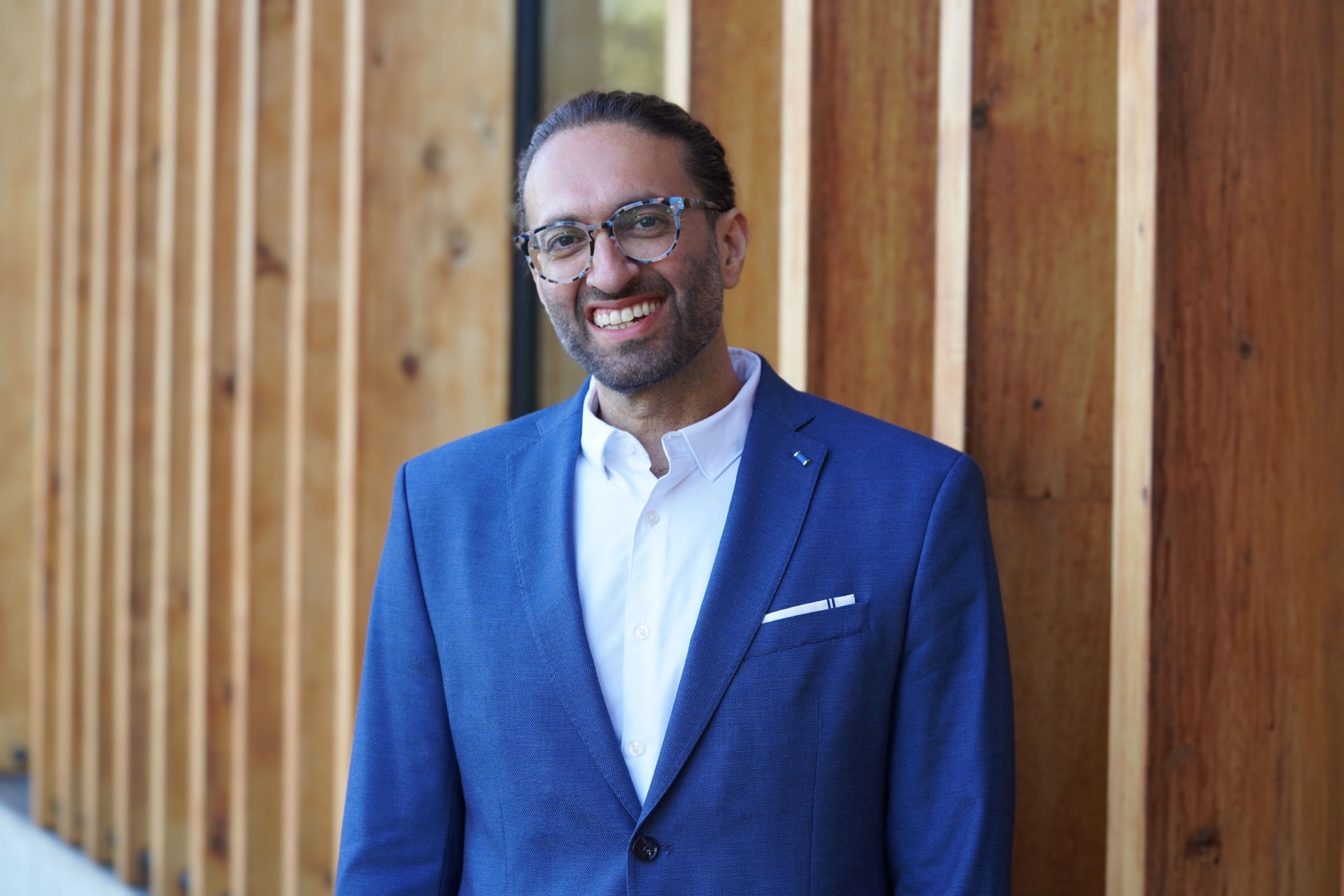
Chief Science Officer and Chief Medical Officer
SelfDecode
Puya Yazdi is the chief science officer and chief medical officer at SelfDecode. He is a physician-scientist with over 15 years of success in R&D, including over 7 years of executive experience. He received his undergraduate education at the University of California at Irvine, a medical doctorate from the University of Southern California, and he was a resident physician at Stanford University. He has successfully developed more than 10 precision medicine products. He has authored peer-reviewed publications, white papers, and lay publications and has overseen intellectual property acquisitions, clinical trials, and regulatory filings. His mission in life is to bring the power and promise of genomics and AI/ML to the masses and help transform healthcare in the 21st century.
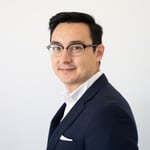
Chief Scientific Officer and Co-Founder
Almaden Genomics
Mark Kunitomi is the chief scientific officer and co-founder of Almaden Genomics. With over a decade of experience in research and development, he brings a wealth of knowledge and expertise to the company. Kunitomi received his doctorate from University of California, San Francisco. He focuses on the development of cutting-edge software and algorithms for the analysis and interpretation of bioinformatic data. He is a recognized leader in the field, with numerous publications and patents to his name. At Almaden Genomics, Kunitomi leads a team of highly skilled scientists and engineers, working to bring innovative solutions to the market and advancing the field of genomics.
Webinar Transcript
Julia Caro:
Good morning or good afternoon, everyone. Welcome to Genome Webinars. I'm Julia Caro Managing Editor GenomeWeb and I'll be your moderator today. The title of today's webinar is Imputation Strategies and Techniques for Analyzing Complex Traits. This webinar is sponsored by Almaden Genomics. Our speakers today are Dr. Paul Yazdi, Chief Science Officer and Chief Medical Officer itself decode. And Dr. Mark Kunitomi, Chief Scientific Officer and co-founder of Alden Genomics. You may type in a question at any time during this webinar. And you can do this through the Q&A panel, which appears on the right side of your webinar presentation. We'll ask our speakers your questions after the presentations have concluded. Also, if you look at the bottom tray of your window, you will see a series of widgets that can enhance your webinar experience. So, with that, let me turn it over to Dr. Yazdi. Please go ahead.
Dr. Puya Yazdi:
Thank you very much. It's a pleasure for me to give this little presentation virtually to everybody out there. So, let's just get started. I'm very excited to speak with everybody about "Genotype Imputation." Now, this is something that's quite popular. Alright, so there's a couple of things we're going to go over today. First, we're going to just generally talk about the general idea behind genotype imputation. What it actually is. Second, we're going to go through some various models and their strengths and limitations. And, finally, we're going to talk about a new imputation method that me and our team have developed that self to code that I'm quite proud of. So, we'll talk a little bit about that as well. Okay, so let's just dive right into simply put genotype imputation is when you predict missing genotypes, think about it this way, if anyone's had any kind of DNA microarray done before, you'll typically get around 700,000 genotypes, right? So, the idea behind imputation is, well, we have that information for those 700,000 genotypes, can we use that information to figure out other genotypes that you have, that were not part of the test. So, this is really popular in GWAS. If it wasn't for this GWAS, would just simply be not as powerful as it currently is. And it's increasing in popularity, for many reasons. One, as we'll discuss, it increases power in GWAS it allows you to do meta-analysis, when you take away result take results from multiple different GWAS. You can combine them to get a boost in power. And finally, it involves fine mapping of causal variants, right? GWAS is called GWAS. Because it's an associative of study, it's you know, when you find snips that are associated with some disease, but you know, association is not causation, right.
So, the whole idea behind that is eventually to try to pinpoint down what are the causal variants from the associated one. And that's another thing that imputation is important for boost power on that. And simply put the entire reason why this is even possible. And what all methods currently leveraged is linkage disequilibrium. And recombination. Simply put, like, the DNA you inherit comes in chunks from your mom and dad who inherited in chunks from their mom and dad, and so on, and so on back in evolutionary timescales. And you, as you go further, further, back in evolution timescales, you have more and more recombination that breaks up those chunks. Now, this is great, you know, it's, we all learned this initial biology class. But what it also tells you though, is that you could use this to find missing information, because it's not like you're getting those genotypes inherited individually, it's always coming in these blocks, right? You inherit them in blocks. And that's kind of like the theory. So, let's go through an example of how this works and why this is important. Okay. So, as you see in the bottom left over here, this is classically what you get, you'll get a microarray done. Let's say you wanted to do microarray sequencing, like 100,000 people, and you have a list of sites on the microarray, that the machine gives you the genotypes for those people. Now, if you did GWAS just on this, and you're associated with some disease, you might get something like these values for these genotypes. As you can see, it's there is an association there. But it's also not very clear, because typically a microarray you get one or two genotypes in certain chunks, and they're very spaced far apart on the genome. So, it's your it's not at the signal strength ratio is not that strong. But what you can do is first phase them, which is when we just put them into mom's chromosome or dad's chromosome, and you've known you have phase genotypes. And he asked his question, the ones that are missing the ones that we didn't genotype directly with the machine, can we infer what those are based on some other parameter, right? And that's basically what it allows you to do. Because what you can do in genotype imputation is you have a reference have a bunch of people who have maybe whole genome sequencing data down on the right.
So, you have all their genotypes, not just the ones you genotype in your study, but in this reference population that you know all the genotypes that they have, right. And based on this and take into account the fact that we all inherit DNA in chunks, and these kinds of linkage disequilibrium chunks of DNA, we have built statistical models that use this reference, and this information in order to guess what you're missing genotype. So that's why it's called genotype imputation. Right? So, you know, the over here, you can sort of see it. And we'll get a little bit more into how this is done through the line. But it's almost like a way to think about it. And the color coordinates kind of show it over here is you can start thinking of, okay, I'm inheriting image chunks. And so, we have these chunks, how can we kind of like stick these chunks together, in order to create your DNA in this person's DNA, right? And so that's what you almost do over here, it's almost like we're sticking these chunks from the reference, seeing what looks similar to what we have, based on that sticking different parts together. And from that, you can infer these missing genotypes. And as you see, then when you perform your GWAS, you suddenly get a big boost in power. And it makes it easier to find that the signal strength is over here, in this one that pops up, right? So, let's go through another example.
So, you'll see this one really clear. So, you know, you're black are all the observed snips, these are all the single nucleotide polymorphisms you had in your microarray kit, these are all the ones that were sequenced directly for all your test subjects. And then you do it in a used imputation. And you got a bunch of other snips to go along with it. And as you can see, the signal becomes a lot easier to pop a lot easier to see a lot easier to discover once you combine these kinds of imputed snips, with your directly genotype snips, and you can and this kind of helps you detect where the causal variants of it, right, so allows us kind of fine mapping allows this boosting power, all these things that were very highly interested in. Alright, so how does this work? We'll keep discussing this a little bit. But basically, every single genotype imputation method that's currently used, or that's popularly used all basically work with the same statistical framework, which is called a "Hidden Markov Model." Now, this is a very useful but complex model. But it basically works with this assumption. And so, we'll talk a little bit what Markov chains are, what hidden Markov models are. But the basic idea is this. You have an observation, which in our case, our observation is the observed genotypes. It's the genotypes you are directly sequencing from the microarray machine that you know exactly what those genotypes are. And the goal from that is to infer some hidden state, which is a reference haplotype. Right. So, it's a chunk of DNA that you're trying to infer based on only observing the genotypes that come off the machine. And so, the idea was, what kind of statistical model can you use for this, and a hidden Markov Model works perfectly for this. Now, the reason for that is a hidden Markov Model is called Hidden, because you're the thing you are trying to decipher is hidden is not observed directly. And the reason it's called a Markov Model is a base something we call the Markov property, which is the idea that any event or any state is only influenced by the preceding state, and not anything before that. So, let's go through that make it very simple.
So, in our situation would be a very way to think about it is, if you're trying to determine which haplotype somebody belongs to, based on just observing their genotypes, the only thing that matters is when you're looking at the current haplotype there are. So, when you're trying to figure out the next haplotype that is only influenced by the current haplotype, they're on and all the haplotypes that came before that, like all the chunks of DNA that came from all the other chromosomes don't really matter. Right. So that's a Markov property, because it's only really dependent on the state. Right. And so based on this, you know, they have these nice, fancy, hidden Markov models, they have these great algorithms for that, you can basically run these algorithms and kind of decipher what's going on. Now, I should also tell you that make it very simple, but that the guys have figured out how to do this actually, they got a very big paper, and it's quite popular, and it's called the Lee Stephens hidden Markov model after two guys, Lee and Stevens who came up with it. population genetics is really big, long field that has like over 100 years of research in it, if not more, and, you know, they've come up with tons of statistical models to explain evolutionary forces to figure out recombination to figure out, you know, all this information. But the issue was, most of these models, you know, while they were great mathematically, and they made a lot of sense in the review of For, you couldn't actually use them in real life when you started getting genotype data because they were computationally intractable. It wasn't something you can easily solve and have a computer in for. And so, what happens is Li-Stephens came up with a model. And they originally use it to figure out the recombination rate, that was the original thing, what they use it for. And then everyone else figured out well, you can use the same model to do other things like genotype imputation. And it's a very simple model. It's a haplotype copying model. And it forms the basis of everything. Not everything, but everything in genotype imputation, and phasing and a bunch of other things that statistical trends. So, it's a probabilistic generative model, which supposes that a sampled chromosome is an imperfect mosaic of other chromosomes found in a population. So, let's put this into normal English, right? Which is that, if I am trying to find out what your chromosome looks like, you can make an assumption that a way to think about what your chromosome looks like is that if I had the chromosomes of a bunch of other people, your chromosome would basically be a mosaic of all these other people's chromosomes, because you know, we all had ancestors, and we have tons of common ancestors. And if you stuck their pieces of their chromosomes together, you would reproduce your chromosome minus one edition, which is that it's an imperfect copy. Why? Because there's mutations, right?
So, as we don't always keep the same exact DNA that our ancestors had because you for mutations. And so, this was like a very simple deduction. But it's a very powerful deduction, because once you make this deduction, that you can view someone's chromosome as an imperfect mosaic of other chromosomes found in a population, then the idea is like, well, how can we use a statistical model in order to figure this out? And so, you can think of it as almost as a copying path through chromosome space. So, you have a bunch of people's chromosomes, data, ones and zeros, whether they have the reference allele, or the allele (11:59). And you can view the person's chromosome as literally almost taking a path through the space, does it go up? Does it go down, and there's a graph next slide will show what we mean by that. And this is how you can kind of stick people's chromosomes together and for your persons chromosomes. And now there's, there's a couple of things you should note, well, it's a population genetic model. And you know, there's always these two users specify parameters, (12:26) theta and rho. And usually, there's interpreted as the rates of mutation and recombination, that's what they're thought of the actual values that go into these algorithms have no bearing on reality of what's going on, just so this is always a point, because the first time I ever encountered this, I was like, this cannot be right. This recombination rate is way too large. This mutation rate is way too small, but it's just the parameters you have to use for the statistical models to work and give you good results. So, the biological phenomenon, they actually represent this off. So, if you're biologists and you see that you're going to think something wrong. It's just how it works. Okay, so here's what I mean that you know. So, we have your study sample here, you can easily see you know, what the genotypes, you've observed, the ones that come off the machine, and then you can see all the reference haplotypes. And you can see what we mean here. Now, really quick, before we even do imputation. Remember that when you get the genotypes off the machine, it always comes with genotypes. It doesn't come mom's chromosome, Dad's chromosomes split up.
So, there's always things that are pre phase, and you always have to face the data before you impute the data. Oddly enough, not surprisingly, the same exact algorithms, the least Stephens model is also used as a phasing elegant, right, so it's the same idea you can use it for face. So, let's say we face our data, we put a mom's chromosome dad's chromosome, and now we have our, user data. And we have like, you know, these three sites that we know their genotypes for, and we've already phased them, we know which chromosomes they belong to. Let's figure out where, how do we create this path through this chromosome space? How do we go through these reference haplotypes stick them together in order to create this person sample. And, you know, these hidden Markov models do exactly that, you know, these statistical algorithms thoroughly fully developed, you just have to apply the algorithms. And based on that, you know, you kind of stick it together, and it's literally you'll see the music in this picture. And based on that, now that you've stuck them together, you go well, it's very simple. So now I know, this reference, and then this reference, we stick them together here. All those missing question marks where you didn't know the genotypes for you didn't know what alleles they had taken them right off your reference. And now you have, and it's quite powerful because it's quite accurate. Now, let's go to something we did with this, which is, since there's all these different tools, there's all these different parameters. We wanted to figure out. Okay, so, what's a good imputation tool? What's a good phasing tool? What's a good reference set? Let's test that all these are and so, you know, I'm making it sound very simple, you got your data over here, you know, you have your reference populations over there, you run a simple hit mark off when you get results, well, you know, that's all great. But you also have to get the data format, in order to do this, there's a lot of preprocessing that goes on over here.
So, for instance, you always are going to get raw data of some sort, it may be data on a different genome bill, genome 37, versus genome 38, it may not be normalized, and so on. So, there's all this preprocessing, and most of the, there's a bunch of tools to do all this preprocessing. So, for instance, in our case, you know, we had to take the raw data, you know, we've used VCF tools to, you know, prefix, the annotations, you know, get the right annotations in place, you know, to split multi allelic sites, these programs don't work well on multiple sites, so you typically split them. And then you have to normalize, you know, you have to make sure that, you know, all your variants are kind of like in proper order that are left aligned so that when you say like the variant that references this position, we know exactly what it is. And then the alternative allele is exactly fixed into that position, you have to get rid of sometimes rare variants. What we mean by that is like, you know, a single 10, for instance, is a mutation, you find it an individual, you can impute that, right. So, if you have a part of your reference files, there's a bunch of Singleton's in there, you typically remove that if you only finding one or two copies, those are Singleton's, you're not going to be able to impute that it's just messes up your data anyway. And so on. So, you have to do all this, like processing to typically get started. And then what we did is we did all this processing, and then we test it out multiple variables, right. So, we basically did three different phasing algorithms, versus three different imputation algorithms, like I said, you'll notice Beagle 5.4, is in both of them, it's both the phasing and imputation algorithm, a lot of them, once again, they're all these things that mark up more. So, make sense that you would do it for both. And we also tested do two different reference panels, 1000s, both them or 1000, Genome Project reference panels, but you know, there's different iterations of that there's the 30x, and there's phase three, and so on. And, and part of our work over here was, you know, we tested out all these combinations in order to try to figure out like, you know, is there an optimal combination. So, in total, we ended up testing up 36 different combinations, to get our results. Now, we're going to show a very, very cool video over here, which I'm quite excited to show. So let me get this started.
Okay, so this cool video over here. So, part of the thing is, we decided to build this on the Almaden Genomics genome platform, because it kind of makes testing these things out much more efficient. It saves a lot of time, because as you'll notice, there's tons of different variables, you can change on these things for your software. And if you're using like command line tools, you're buying from your tissues, they're going to, you know, pull their hair out is they're constantly writing code. So, on the genome platform, you can get the stuff running, you can get it operational, it's almost like a plug and play. It's almost like a video game in many ways. And it's what I love about it, actually, because it looks fantastic visually, and you can get it in there. And based on that, you can kind of speed this up. So, if you're trying to figure out what the best parameters to use, and so on, you can, you know, get that going and, and move very forward. So, it's quite cool. And it basically ended up saving us a lot of time to play on it. And the other thing that's great about it is if you have a genomic scientists who know how to I don't know, tons of code, these are not data engineers, he's not like hardcore by information's, they can still get something like this operational, and still get you to the kind of quality data, which always I love hearing because I was originally was a physician, and I was never really trained to code properly. So anytime I hear about anything that makes it easier for me to get stuff up and running. It's exactly what I love to hear.
So. Okay, and as you see it kind of, you know, stole a little bit of thunder over here. But typically, on these kinds of pipelines, one of the real frustrations, things in bioinformatics are you always takes forever to get a pipeline going, you always have to iterate over it, you have to fix stuff. And typically, you know, you'll see stuff like you know, two weeks, three weeks, eight weeks, you know, in total, you're spending like 13 weeks. In total, you're spending weeks to months to build something right on the genome platform, because a lot of the utilities are already there. And it's simply plug and play, drag and drop stitch them together, you're going to save tons of time. So, in our use case over here, you're basically saving about 13 weeks, 13 weeks of work just to get the pipeline operational to do all these kinds of tests. So you know, 70% reduction in runtime, and 25x reduction in runtime costs, you know, it's going to save tons of money and it's and like I said, for me personally, what I about it as you can have actual scientists who know a little bit about computers, working with these kinds of datasets without the need to have, like, you know, a team full of foreign politicians running around write code that to me is by far the coolest prospect that in the fact that, you know, you can run through all these parameters, you can also test for multiple these things, and kind of get this, change things around and iterate really quickly to kind of get at your solution without spending weeks and months of writing code for everything. Okay, so we use that we got all those testing done. So, what did our results want? Now, there's a couple of interesting things.
So, this is phasing. Alright, so once again, remember, phasing is like impatient phasing is when you just split the mom and dad's chromosome, get them on the right one. And then you can do imputation. So, phasing effects imputation, right. And so, there's basically two different ways you can face, you can phase reference based or reference three. Reference base is simple as before, you have a bunch of reference samples in a population. And based on these reference samples in a population, you use that to phase one sample at a time. Typically, it doesn't have to be one sample of time. But a good typical use case for that is one sample at a time. And, you know, as a direct-to-consumer genetics company, you do that all the time, right? When you get the genotype data, it's not like it's some study where you had 300,000 samples all show up at the same time. So, you're getting one at a time. And as you're getting it, you're typically running it through with a reference set for phasing. And, based on that, you get your results. And as you can see, it's pretty accurate. And then there's also reference free. Now reference free is, you know, there is no reference for phasing. This is typically used when you have 1000s to hundreds of 1000s of millions of samples, it's almost typically done in a study, you know, and we phase them all together without reference. Now, there's first thing you'll really notice is the difference between accuracies for all these different software, even, you know, reference is miniscule, when you typically think of like accuracy measures, right? 93.4% versus 94.15%, you know, it seems minor, and you know, most people are going to brush it off. Now, depending on your use case. So, this could matter. Remember, the genome is quite large, we're talking about, you know, billions of pieces of information. So even 1% is, you know, a lot of differences, right. So, it really depends on your use case. Now, one of the things I really like to tell people is when you go reference base, or when you go reference three, reference free makes a lot of sense, if it's typically a study in which you genotype a bunch of people on the same exact machines, after you collected all the samples. One of the reasons why it works, so I'm going to do reference free is that the machines always have uncertainty in them. Remember it, you know, the rules of physics don't apply, don't stop, just because we're doing biology or genetics, right, any kind of machine that measures anything is always going to have a little bit of uncertainty, there's always going to be inherent biases in the machine, there's always going to be ones that you know, where the signal intensity of the laser was a little offensive zone. It's all this produces kind of loose kind of biases in your data.
Now, when you do these kind of reference free methods, what happens is because they were all done with the machine at the similar time, similar workflow, it's almost like baked into it, and like, you know, the algorithm can sort of figure this out and still, you know, phase them properly on the two. And you don't have that benefit. If you go reference based with reference base, you know, it's one sample at a time. But if you're, you know, a direct-to-consumer genetic company, or you have different kinds of machines using microarray data at different time points, you know, you know, at first one, we did dislocation, the second one, we did a completely different location way later, and so on. That makes sense as a reference base, right, you're going to run those for reference base, and based on that, you're going to decide which ones do you know, maybe in your case, you ship it for, you know, or if you do in reference free, you know, ship for also works? Well, you know, so yeah, you have these kinds of choices. Like I said, the difference in accuracies is very, very minor. So, you have to really, really think about what your use case and what the question is you're trying to answer. If it's something where you know, the accuracy really, really matters. And we'll actually get through a case why we've developed our own imputation method, then, you know, you want to make sure you pick up the most accurate one. For your use case, the accuracy could be you know, what's 1%, you're just interested in something else, maybe you'll pick on computational efficiency, or cost or something like that. So now, that was the phasing.
Now we'll talk about imputation. Now, one of the things you should know about imputation, as we'll go through some of this information is imputation is really, really kind of dependent. The equality or imputation is dependent on multiple factors. So, it's not a one ship all metrics. Total accuracy really is not that meaningful when it comes to imputation. And so, in this case, you should know that IQs Basic Stanford stands for "Imputation Quality Score." People in the field came up with a different metric Then accuracy in order to gauge imputation accuracy. And the reason he did for that is that chance always plays a factor especially, you know, you're imputing, a variant that's found in 95% of the population. Even the crappiest model is going to start guessing right after a while, you know, so you kind of want to take all the sudden council that came up with this IQs score as a way of doing it. And here, what we did is we basically broke down minor allele frequency stratification of these imputed variants, to figure out what happens as your minor allele frequency decreases. Now, the way this figure is set up, the real take home from the figure is something that's not so surprising, if you've read other papers is that as your minor allele frequency decreases, especially when you get to 1%, and lower imputation just falls off a cliff. This is typically the reason why a lot of times people will cap them amputate their GWAS at like some common allele frequency. And the reason is, you know, imputing rare variants is very hard, anything less than 1%, it's typically highly, highly inaccurate. Now, there's ways to get around that. One way to get around that is if you have like a massively deep Reference panel, you know, like, for instance, there was paper published last year in which they had, they started releasing some of the UK Biobank whole genome sequencing data. So, they had 150,000 of the 500,000 samples, you know, see deep sequence, and they use that to impute the rest of the UK Biobank and you know, they were able to impute lower than 1%. Because of this, you know, you have, but you may not have access to 150,000 samples for your job. And even if you had access to 150,000, whole genome sequencing, that might drive you, it's very expensive to use that reference for much more expensive. So, you may want to cap yours. But the basic idea is that as you especially when it comes to these rare variants, anything less than 1%, typically imputation is not very accurate. And as you go higher, it becomes more accurate. You know, so just makes intuitive sense. The more common an allele is in a population, the more times you see it in your reference population, the better your algorithm is at guessing who has this, and who doesn't have this, restrict poll. But here's some more interesting stuff, at least I find a little bit more interesting is that it's all affected by the ancestry of your dataset, whether it's the awareness, that's the ancestry of the actual reference samples you have, or the ancestry of the user, or the one you're trying to target population.
And so ancestry also affects your imputation quality score, which is another reason why you want to look for if you're imputing, a tons of variants with a broad ancestry background, you want to have a broad reference sample that has, you know, just not white Europeans in it, you want other kinds of ancestors in there, if your users if your users or your targets also happen. This graph basically shows the same thing is that you know, that in this one is a little bit different, because in this one, what they did is they go, okay, we're just going to use the same reference the same 120, you know, 120 samples for everybody. And now we'll just look at what happens to different users with different ancestral backgrounds. And not surprisingly, that, you know, like I said, both whether you have which ancestors you have in your reference sample, and the target ref ancestry also affects imputation. So, as you can see, there's some limitations there, which is sort of why we kind of developed our own imputation method, we're not going to get into too much of the technical details, I'll kind of blitz through this a little bit. But basically, we wanted it for specific use case. rare variants, we're very interested in rare variants, because rare variants have much higher medical significance than common variants are, they typically have much larger effect sizes, when it comes to disease, and so on, so on. So, we're obsessed with this idea of like, we should be able to get much better at imputing rare variants. So, we specifically tried to come up with an imputation method that was much better at doing rare variants. Like everything else, it uses the Li-Stevens Hidden Markov Model. But what we did was slightly different is that we basically did this really cool algorithm called a bidirectional PBWT. PBWT stands for "Positional Burrows Wheeler Transform." Before you know, you start Googling and actually figure out, the best way to think about it is it's just an efficient algorithm for storing, compressing and searching genetic data at basically what it is. And the reason it's bidirectional is that it does this in two directions. So, you don't just go you know, left to right, you can also go right to left. And so, this is very ideal when it comes to the imputation.
So, if you have a snip of interest, you have a marker of interest, you know, you can search both like you know, in one direction, and then the other direction. And based on this, you can find what the potential haplotypes are, that are most likely to be found in this target one. And you know, the other thing we did over here, I shouldn't even say really, it's really the scientists I had who are working on this who might be or Adriana and Abdullah, basically, the other cool thing they did is that a lot of times, you and somebody else might share an allele of a specific population. And this could you know, and this is either shared because it's identical, identical by state or identical by descent, if we know for sure that we share the same allele, because we inherited from a common ancestor, we call that identical by descent, as opposed to identify state. And why does this matter? Because, well, that is what you're looking for, when you're doing imputation, what you're really trying to find is, let's find people who share a common ancestor with me, and hence, would share alleles that are identical by descent. So, they also wrote this really cool ristic for, you know, making it easier, being more precise of when you think we have identical by descent. And you know that they typically ran the same hidden Markov model, and, you know, they use the forward backward algorithm to, you know, compute the, the necessary data, and so on, and so on. But here's the data. Okay. So it's very cool algorithm, it's, you know, like I said, it uses least seven, we just did some minor texture, because we're interested in making it easier and better at, you know, finding where this music is, because that's what it's really about, you know, the least students came up with this idea, you want to find which mosaics to stick together. And you know, and what they did is they basically came up with this cool way of sticking these better together. And, you know, we did a little test, we compute a comparative against Beagle, 5.4, and cute five and minimac, which you saw previous data for. Now, this graph to make it easier to visualize, basically, we just looked at, you know, the errors, and we did like a log folder enrichment of the errors. So, it's just counting how many errors each half. So, the lower the number, the more accurate is, and we broke, bend it into different minimum allele frequencies. And as you can see, the greenest selfie, which is the model that they came up with, this consistently performs better at all middle allele frequencies compared to the others. But it's really at the bottom here is why, why we've done all this work on this is it's much better at imputing, his rare variants, which is really what we're interested in. And the same thing, we did it, we wanted to make sure because like I said, the ancestral origin also affects this, we tested this in different ancestries, you know, African, East Asian, European, South Asian, and you know, consistently, once again, the green, selfie, it was much better at the imputing overall, compared to the other ones. And so, with that said, let's try to get a little summary of what we discussed today. And then move forward.
Okay, so like I said, you know, imputation has tons of benefits, right? Let's just repeat some because, you know, this is important stuff. It increases statistical power by predicting unobserved genetic variations, right? Li-Stephen said, Markov model, the whole idea is like, whenever I'm looking at anybody's DNA, whether it's my DNA or anybody else's DNA, if you're looking at the entire chromosome that somebody has, they, we can think of it as an imperfect mosaic of other people's chromosomes, right? So, once you have an imperfect mosaic of other people's chromosomes, we can infer what is going on from there based on that, right. And it's cost effective, right? If you want to do whole genome sequencing on, you know, your 150,000 samples, that is going to cost a lot more than taking those 150,000 samples and doing genotype imputation on them, and then followed by a microarray and then followed by genotype imputation. So that's much more cost effective, right? And then feels meta-analysis. What do we mean by that? There's different GWAS, right? Group A did a GWAS last year, and one point in time Group B did another GWAS later, you can take that information, and then do a meta-analysis with imputation. In order to combine those results into one meta-analysis, which boosts statistical power, enables fine mapping. Once again, if you have more information on more of these variants, especially the rare variants as well, that you know, who I've been working on that you can start fine mapping, you know, it's no longer about associations. It's about causality. Okay, so which variant actually causes this disease, right? Its causal in this case. Like we said, imputation accuracy varies across ancestral populations. Our own algorithms, selfie achieves higher accuracy and variants across various ancestral populations, and also as importantly, as much better at rare variants. So those are ones where the most interested in Bioinformatics Pipeline Development for application specific use cases and mutation requires testing out many tools and parameters. As you saw on that you got to constantly changes some of these parameters. Each one of these parameters can affect what your use cases so you have to constantly testing these things out, which is ideally why something like the Almaden Genomics genome platform is now with your use case, because you can start testing those things out, and based on that get much higher turnaround times with your data. So, I will now hand this off to mark, and to take the rest of the presentation. Thank you.
Dr. Mark Kunitomi:
Thanks so much Dr. Puya Yazdi, yeah, no, it's really fantastic work, I have to say, you know, from the first times, I saw the work on different ancestral populations that really blew my mind. And, of course, seeing the way that selfie is able to get higher accuracy for invitation, not just within, you know, the large corpus of data, but across different ancestral populations, I think that it's just has tremendous potential. So, I'd like to tell you guys a little bit more about our platform at Almaden Genomics. Think that from working together with Dr. Puya Yazdi team, we kind of learned that the key to successful imputation strategies is to employ lots of different tooling, explore lots of different parameters, and then be able to move from the types of proof of concepts that get developed to solutions that kind of scale seamlessly to the large amount of data that they need to be applied to. And this is really where our platform at Almaden Genomics Genome, it really shines. First and foremost, it's kind of an intuitive drag and drop graphical user interface, combined with a curated library of tools and prebuilt workflows, which allows you to rapidly collaborate, build and run Bioinformatics Pipelines, then, in addition, genome has this automatic scalability and proven cloud computing architecture, which is built in and that allows you to process these large-scale datasets with great efficiency. Well, that's just what makes it really easy to use, and to work together with groups like Puya Yazdi's, at self to code. So, you know, for you out in the audience, whether you're really looking to run premade workflows right out of the box, to solve your bioinformatics problems. Or you're looking to develop new solutions, custom solutions to kind of solve your science and business needs. Genome really has you covered. So, we'd love to hear back from members of the audience, to have people reach out. On the right hand of this slide, we've got a QR code, so you can look us up, you can also check us out on the web at almaden.io or, or reach out to me personally, and we'd love to show you, you know how the platform can help accelerate your research and work together to show how it can be applied to your organization's problems. So, thank you so much for your time. And we'd love to take any questions that come from the audience. So, I'll turn it over.
Julia Caro:
Yeah, thank you very much Mark, for this quick introduction to your product. And also thank you to Dr. Puya, for your nice overview of your work itself to code. As a quick reminder, if you have a question, please type it in the Q&A panel. And we'd also like to ask our attendees to take a brief moment after the webinar is finished to take our exit survey and provide us with your feedback. So, let's see what we have for questions. Yeah, here's a question that came in during Puya's presentation when you were talking about the reference based versus referenced free phasing. And the question is, how's this approach viewed by the FDA? Can you say anything about that?
Dr. Puya Yazdi:
So, like I said, originally, this method was really used for research purposes, and wasn't really used in any kind of setting where you need FDA approval. Now, that being said, is, as I understand it, there is actually a couple places where it's used. So, I think it's fine from an FDA perspective. Now, obviously, if you were trying to, you know, build a test based on imputation, you most likely would have to have a more, we'll have to have a stringent cut off of whether your application is actually accurate or not. But from a regulatory standpoint, it should be fine. Currently, there's a lot of AI models being approved by the FDA, and so on. So, I think it'd be fine. It's just that you would probably have to use a much more stringent cut off of where you're imputing correctly and where you're not imputed correctly. If you have had to, you know, take this through an FDA regulatory process, but I don't see a problem with it and at just announced, but I do know a couple other groups that are actually in the process of trying to, you know, get a microarray based with field data imputation method through an FDA process for polygenic risk score. And I do know, what they basically decided is just good, we're going to do all the common variants. So, they didn't see a problem with that from their FDA oversight. So, I don't think it's going to be a major issue. Okay.
Julia Caro:
Okay. That's good to know. Great, thank you. Another question for you, would it be possible to bring Pedigree Information into the imputation? process?
Dr. Puya Yazdi:
So yeah, but so typically, not none of these. So, there are like some people who use that information for the different ways of doing imputation. But this kind of information, is it? It is possible. Not that many people do it. Because if you, for instance, like I'll give you an example, if you, for instance, sometimes a lot, sometimes adding more information to these statistical things don't actually yield better results. Sometimes what they do is they kind of, you know, make it less accurate and other use cases. But yeah, you could theoretically do that, whether it's going to give you a higher overall result at the end or not, depends on, you know, kind of skill framework you use or not. So, oh, yeah.
Julia Caro:
And then a very quick technical question, what would be the smallest number of snips and individuals to do imputation on?
Dr. Puya Yazdi:
Okay, so this, you know, the right you need, you know, early on, they only had 120 reference samples. So, you know, if you have 120, they got some sort of data on there. So, you know, if you have one target 120 references, you get something now, how accurate is going to be that it's, you know, it's not going to be the world's most accurate thing, it's, it's not really an idea of possibility, it's really an idea of how many is needed for your accuracy that you're going forward. So basically, you know, the more variants you have on your genotype ship, the better it is, and the more references you have, the better it is. Now, there is a couple of things you should be aware of really old genotype shifts, that weren't properly spaced out, like the first ones that came up, it's really hard to do imputation, you need to have at least one marker from the haplotype. From the section of DNA, you're interested in order to find the missing pieces of information. If you don't have any observable data there, if you don't have any genotype data there from that section, you're not going to be able to compute it. So once again, it's a question of what's your use case, you know, let's say you have a genotype shape where you only have 50,000 snips, well, you know, you're not going to be able to impute the whole genome based on that, but you will be able to impute larger sections of those 50 50,000 snips, way to think about imputation is, you know, almost, it's always like, Okay, I have a snip here, this allows me to find the information on this larger block of DNA, you know, and the whole idea is, well, if I have enough well positioned snips all throughout your genome, you can find information from all over the chromosome, right? So, it's really a use case question. Right?
Julia Caro:
Okay. Are there cases where phasing can be avoided, avoided altogether? And what are those cases?
Dr. Puya Yazdi:
So actually, some of the software phases that while your period, so you don't have to even pre phase it, but it's impossible to impute without the above analysis possible. The whole idea is trying to figure out where What shocks is you basically need to have them phase in one form or another. So, but you don't sometimes I believe it's Beagle, don't hold me to this, but I'm pretty sure it's people, you can actually give it just data, it'll phase while in imputes at the same time, so you don't have to worry about that part. I believe people fly Flipboard. Does that. So, there are cases if, if that's what you mean by avoided? Yes. That's what you mean by avoided? Most of these hidden Markov models are they're all haplotype replication. So, you need to have some phasing support, but the software can take care of it in many cases.
Julia Caro:
Yeah. And then this is probably something you are not interested in personally with your business. But what about using this for non-human species? Do you know anything about that? That? Yeah, absolutely.
Dr. Puya Yazdi:
So, a couple of things to be aware of, you can use this for any species. That you know, as long as it's eukaryotic, this basically takes advantage of eukaryotic DNA, right? We all eukaryotes, inherit DNA chunks, and they all have recombination, you know, stuff like that. The key though, is you do need a decent amount of reference samples. If you're, you know, for instance, I'll give an example. I mean, I'd love to do this, but you could definitely do this in dogs, right. But you would need to have sequence that good number of breeds because, you know, it's not one reason that the same, so the amount of reference samples you have, but it's absolutely able to, and in fact, it's used a lot in non-human use cases, a lot of different companies for various reasons. You know, they want to like for instance, genotype array, a bunch of, you know, crops, they don't want a whole genome sequencing. So, they have some reference panels and based on that they can you know, do that, but yeah, it means anything. It's completely agnostic to what species it comes to. So long as it's, you know, it's it undergoes recombination and has linkage to sickle, right?
Julia Caro:
Yeah. Okay. Great. Good to know. And then I mean, this is all what you talked about was in the context of keywords and using microarrays. But there's a lot being done with sequencing nowadays. And so, the question about how does long read sequencing, in particular change the problem of phasing and imputation?
Dr. Puya Yazdi:
Well, it's actually a great question of that. So, imputation, is also used with sequencing data. Okay, so this is cool. So, you know, I give an example of the microarray, because that's typically what's been useful. But, you know, a current hot thing is what they call like, you know, low, you know, low coverage, or low read sequencing, or whatever you want to say, it's like, instead of doing a 30x, you know, someone does like a point 1x, or point 5x 1x, and then imputes, to fill in the rest, right and the most, but it's a little bit different, I got to tell you, it's a little bit different. So, the slight difference is, when you observe your genotypes from the microarray machine, you're assuming it's 99% accurate. So, your hidden Markov model, these are parameters that go in there, you're assuming it's pretty accurate. Now, if it's a low coverage, it's your it's not the same. So. But if the same basic concept works, just the parameters change, they have their own hidden Markov models. In essence, they're also least even hidden Markov models, it the same thing works even that. And, you know, I'll give you a use case, I'm not sure anyone has done this. But you know, someone probably does, it's internally somewhere. I mean, it's probably even possible to, you know, take these long-read sequences, you know, the new machines, they have accuracy issues. Now, they're great at giving you large chunks of DNA, but they have accuracy issues, and then you could probably just set one of these up with the imputation panel. And based on that, improve the results of your laundry sequence. So, there's great Interplay here, you know, as that new technology comes about, and we're going to get better phase data, which is going to make our phasing but work better. And additionally, then you can use the same algorithms with some slight variations, it's going to be you typically have their own algorithms for it. But once again, they're very similar, at least in some market. Well, so. But yeah.
Dr. Mark Kunitomi:
Great, seems like it could be useful to kind of check how well the phasing is doing using long read technology as well, since, you know, inherently since the longer you technology is, you know, only doing one haplotype at a time, you get much longer you much more confidence in the connections or the phasing between the variants there, because we also used to check your methodology.
Julia Caro:
Yeah. Mark has a question for you. So, the platform that Ahmed Engine service offers, offers the possibility to perform imputation in an automatic way. That's the question as to that.
Dr. Mark Kunitomi:
Yeah, so for workloads that we have that are prebuilt, you can just bring in your data and start to try it out of the box. Now, for something like imputation, there's still going to be parameterization that you're going to check, as well as being able to check it against your specific expectations of the data. And so, workflow can be, you know, usable out of the box to give you that start, I still think that you're going to want to be able to modify the parameters of that workflow, in order to make sure that it's optimized for your use case. But absolutely, that and that's part of what genome is for, is having those premade workflows in addition to being able to build your own.
Julia Caro:
Okay, and then maybe a related question, how many tools are currently available on Genome? And are you adding new tools?
Dr. Mark Kunitomi:
Yes, that's a great question. We're adding new tools all the time, I wouldn't be able to give you the count as of today, because our competitions are bringing them in constantly. So, we have over 100 at this point, and we have massive expandability of a platform. In fact, our users can bring in their own tools using a graphical user interface, as well. And so bringing in your own tool, whether it's your custom code, a piece of open source software that we haven't brought in ourselves, or bringing in kind of the latest and greatest tools, something like selfie, if you needed to bring in something that was published a week ago, that's something you can bring in very rapidly on your own, using a graphical user interface takes about anywhere from 15 minutes to half hour to bring in any given tool that we don't already have wrapped. Okay, we're expanding our tool collection all the time, but you also have the ability to expand it yourself in a very simple way.
Julia Caro:
Good to know great question probably for either of you. Our integration techniques suitable for single cell genome data. I mean, I guess there are so long as it's different people, right? But of course, what's your take on it?
Dr. Puya Yazdi:
Yea, I don't, obviously, if I understand the question they are. So, you know, you know, DNA is DNA. So, if you have, but there could be some things here. I don't fully understand if I would love if that person actually sent me an email. Tell me exactly what why they were interested in this. But yeah, the thing about it is at the literal, I know about a single cell. Yeah, I'm actually interested if that person contacts me a little further purpose on the surface, they are absolutely suitable. But I'm not sure why you would use in single cell data, because of the fact that I thought most people just doing because of the quality was so bad that the most people had to do whole genome sequencing on it anyway. So, I'm a little bit one.
Dr. Mark Kunitomi:
One place that it might be really valuable is that you're getting differential coverage Puya right? So, in the same way that you want to unify multiple different GWAS studies, or multiple different sequencing studies, where they're sequencing different parts of the genome, and your coverage isn't great. Purcell finding a way to unify that dataset could have tremendous value.
Dr. Puya Yazdi:
It makes sense. So, there you go, Mark, it answered. I was wondering?
Dr. Mark Kunitomi:
That's, but it's a cool question. I would love to see some exploration there purely from a science perspective. But yeah.
Julia Caro:
OK thank you. Thanks. Um, question for Puya. Itself is available for academic use?
Dr. Puya Yazdi:
Absolutely, it is. We have not, it's currently undergoing finished final touches. So, we submitted as a paper, so you can't find it online. But it's absolutely available for academic use. For anybody who's interested, just contact me, I believe my email is going on. But anyone who's interested in academic use, or even a collaboration on it for academic use, please contact me, we would love to collaborate. We can even help you set it up and run and all that stuff. So please contact me.
Julia Caro:
Yea okay. And question that just come in. Can you comment on the concordance rate between whole genome sequencing and imputed data in general, I guess, and what is the advantage of using whole genome sequencer compared to imputed data, you have to do this and is it only is the advantage only on the discovery of rare variants.
Dr. Puya Yazdi:
I don't actually know what the concordance rate is between the two. Off the top my head. So but I will tell you this, the real advantage to doing whole genome sequencing is, you know, the rare variants that literally is, it's literally the by far the most powerful aspect of it, when you do whole genome sequencing, especially if it's like 30x, when you say this person has a really, you know, good loss of function, rare variant knockout, like you figured out this, this protein is knocked out here, because of this rare variant that you've just discovered in three people, you know, they have it, imputation is as good as like, for instance, our ad changes our tip tissue, it's just never going to be as accurate, there's always going to be some uncertainty whether they have it or not. So that's really the real advantage of whole genome sequencing. But it comes at like a huge, massive costs, right? Not only the cost of doing a sequencing, but the cost of storing the data processing, and so on. And so, when people have hundreds of 1000 samples, it just makes more sense to do imputation. But the really, the real advantage is that if you aren't more certain, and this really matters when it comes to the rare variants. Additionally, whole genome sequencing, depending on the kind of techniques you use, it might be more possible that there's certain kinds of variants, you might be able to figure out more like structural variations, that's just not going to happen with imputation. Those kinds of things, especially if during like what, you know, long read sequencing and stuff. So that's really the big advantage.
Julia Caro:
Okay. Okay, great. And, Mark, can you cook a comment on academic use of genome? Is it a commercial product only? Or is there any other academic usability?
Dr. Mark Kunitomi:
Yeah, we're currently working with academics already. And so, I highly encourage you to reach out and connect with us because we'd love to discuss how we can bring it to your lab or to your organization. Absolutely.
Julia Caro:
Sounds good. Let me see. A question that just came in, how complex would it be to impute a low pass genome was 2x mean coverage? Very specific question. And wouldn't the phasing be difficult for that?
Dr. Puya Yazdi:
So, you know, low pass, it's actually the dirt. It's very accurate. Some of these new newer statistical models they have, you're doing like not even two exits, like literally Point 5.1x They've got to work well. So, here's the thing is that phasing and you would think would be difficult, but what happens is when you have like, bigger chunks of the DNA, and it's like falls on top of each other, the phasing algorithm is going a little bit better, actually. So, you know, like I said, there at least even send Markov models with this, they don't use the same thing, like you don't use Beagle for that you don't use shape it for that, and so on. You know, they all work similarly, right, they have the same general idea, but there's specific ones that are designed for low, you know, for low pass sequences for these kinds of low coverage, whatever you want to call sequences, like 1x, one 1x, they work on the same general idea, and they get very good results, they get very, very good results. It's, it's quite startling how good the results are.
Julia Caro:
So right, okay. Just see. So, there's something about something earlier in your presentation Puya, you showed there was an increase in accuracy between the reference three versus the reference-based results? Could that have anything to do with differences in population?
Dr. Puya Yazdi:
Um, yes, or no? So, it really kind of depends. Obviously, if you, I'll give you an example. If you're testing, you're facing with a population, you don't have samples of in your reference, it's going to get lower results. So, in that way, that the question is, right, yes, yes, that could affect it. In our case, that was not the case to kind of control for that. So, we know that for, but yes, you could get if you have your targets, or a different ancestral background, your reference, or vice versa, or anything we want to work in their accuracy, phasing is also going to be a little bit worse. If you're using reference base. Now, if you're using graphics, doing all these together, kind of gets around this stuff.
Julia Caro:
Yeah. Okay. And a related question related to the accuracy of imputation of different populations, what could be done to improve that? Would put more data health?
Dr. Puya Yazdi:
The answer to all of us as always, the same, more data. Alright, so that's always the answer, you know, that's think out of the ditch, you know, believes in that. So, you keep saying more data, more data, more data? So, the answer is always more data, you really do the ideally, you would want tons of references from tons of different ancestral backgrounds, right? Tons of different kinds of people that are admixed, that have these kinds of answers wrapped up, this is always going to improve accuracy. This is a statistical model. And it's a great statistical model. But, you know, it's not like a magic show. Like it can't figure out what haplotypes someone has, if it's never seen that before in reference, right, right. But you have to have the data in order for it to learn, you know, it's not a magic show. So, the key is always to get better and deeper and reference sets that are not just like, you know, we've sequenced 1 million British people living in England, so.
Julia Caro:
Okay, oh, yeah. One more question that just came in. What is the influence of having a database of a mixed population in the prediction of a 1x? Genome?
Dr. Puya Yazdi:
I'm not, I don't have the question. Yeah. And I think I might know what it says. I'll just answer this the last. Yeah. All right. It's the same idea. So, I think what they're what they're saying is that, you know, if you're doing this kind of low, low coverage, once is it the same, it's always the same. You need different ancestral backgrounds in your population, in order for accuracy to be improved. The reason is, it's purely biological, it has nothing to do with the algorithms, right? different populations of ancestry, what really sets them apart is the kind of like linkage blocks they have, how big or how much diversity find it. This, you can't get around lists. You know, the only way to get around that is if you have those in reference, and that you could see it, your algorithm can see it and for it, learn from that and use that to do things properly. But if you can't get around, if you have some ancestral populations, because they're newer, they've had greater population bottleneck. There's just less diverse and you're like, oh, wow, repetition works great in them. But other ancestral populations, like a lot of these African tribes that you know, have been over there, you have a lot of genetic diversity, right. So, you need way more references in order to impute those with accuracy. You can't get around that you have to have a diverse panel in order to compute.
Julia Caro:
Yeah, okay. That's a good closing statement. And this is also all the time we have for questions today. So let me thank our speakers Puya Yazdi and Mark Kunitomi, and our sponsor, Almaden Genomics, and as a reminder, please, please look out for the survey after you after you log out to provide us with your feedback. And if you missed any part of this webinar, or you would like to listen to it again. We will send you a link to an archived version via email Now, with that, thank you very much for attending this genome webinar. Thanks, everyone.